فهرست مطالب
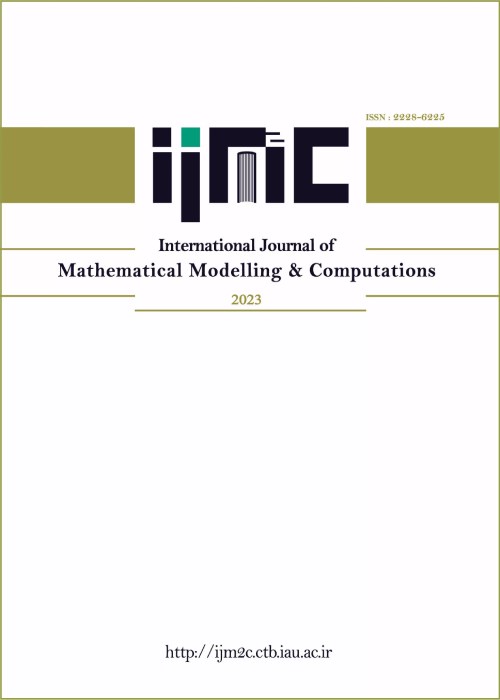
مجله بین المللی محاسبات و مدل سازی ریاضی
سال دوازدهم شماره 4 (Autumn 2022)
- تاریخ انتشار: 1402/01/30
- تعداد عناوین: 6
-
-
Pages 213-224In this paper, a new approach based on weighted sum algorithm is applied to solve multi-objective optimal programming problems (MOOPP) and multi-objective optimal control problems (MOOCP). In this approach, first, we change the problem into a new one whose optimal solution is obtained by solving some single-objective problems simply. Then, we prove that the optimal solutions of the two problems are equal. Numerical examples are presented to show the efficiency of the given approach.Keywords: Multi-objective optimal control problem, Pareto solution, Programming problem, Nondominated solution
-
Pages 225-247The user cold start challenge, occurs when a user joins the system which used recommender systems, for the first time. Since the recommender system has no knowledge of the user preferences at first, it will be difficult to make appropriate recommendations. In this paper, users’ demographics information are used for clustering to find the users with similar preferences in order to improve the cold start challenge by employing the kmeans, k-medoids, and k-prototypes algorithms. The target user’s neighbors are determined by using a hybrid similarity measure including a combination of users’ demographics information similarity and users rating similarity. The asymmetric Pearson correlation coefficient utilized to calculate the user rating similarity, whereas GMR (i.e., global most rated) and GUC(i.e., global user local clustering) strategies are adopted to make recommendations. The proposed method was implemented on MovieLens dataset. The results of this research shows that the MAE of the proposed method has improved the accuracy of the proposals up to about 26% compared to the GMR method and up to about 34% compared to the GUC method. Also, the results show about 60% improvement in terms of rating coverage compared to the GMR and GUC methods.Keywords: Recommender Systems, user cold start, Clustering, hybrid similarity measure, asymmetric Pearson
-
Pages 249-263This paper deals with a spectral collocation method for the numerical solution of linear and nonlinear fractal Mobile/Immobile transport (FM/IT) model with Caputo-Fabrizio fractional derivative (C-F-FD). In the time direction, the finite difference procedure is used to construct a semi-discrete problem and afterwards by applying a Chebyshev-spectral method, we obtain the approximate solution. The unconditional stability of the proposed method is proved which provides the theoretical basis of proposed method for solving the considered equation. Finally, some numerical experiments are included to clarify the efficiency and applicability of our proposed concepts in the sense of accuracy and convergence ratio.Keywords: Fractal Mobile-Immobile Transport (FM, IT) Model, Caputo-Fabrizio Fractional Derivative (C-F-FD), Spectral Approximation, Stability Analysis
-
Pages 265-274The modeling of uncertainty resources is very important in insurance pricing. In this paper, fuzzy set theory is implemented to model interest rates as an uncertainty resources for calculating the price of enhanced annuities. In this regard, the single fuzzy premium for a fixed annuity payouts is calculated using adjusted mortality probabilities for an insured with health problems and the results are compared with standard status. As the adjustment multiplier increases, which means that the health problems of the insured are worse, the life expectancy of the person decreases. In addition, as adjustment multiplier increases, the insurance premium decreases, which is due to the adjustment of survival and mortality probabilities based on the individual's health status. Also, to show the validity of the proposed fuzzy method, the random interest rate has been used. The results of the fuzzy and random models are close to each other which indicates the validation of proposed method.Keywords: Adjustment multiplier Interest rate, Enhanced annuities, Single premium, Fuzzy Set Theory
-
Pages 275-297
The importance of the capital market in economic development is undeniable through the effective management of capital and the optimal allocation of resources. In this study, according to capital market behaviors and research, Statistical Learning (SL) algorithms compared to Artificial Neural Networks (ANN) to analyze time-series data and predict stock prices have been investigated. In studies to compare methods or provide hybrid models, most statistical learning algorithms are limited and examined without the comparison of other algorithms. In this study, to eliminate this shortcoming by implementing and comparing statistical learning algorithms in the two categories of Regression Learner and Classification Learner, the most efficient algorithm has been identified based on the selected shares and based on the presented parameters. The first category (Regression Learner) includes Linear Regression, Interaction Linear Regression, Robust Linear Regression, Stepwise Linear Regression, Fine Tree, Medium Tree, Coarse Tree, Linear Support Vector Machine (SVM), Quadratic SVM, Cubic SVM, Fine Gaussian SVM, Medium Gaussian SVM, Coarse Gaussian SVM, Ensemble Boosted Trees, Ensemble Bagged Trees, Squared Exponential Gaussian Process Regression, Matern 5/2 Gaussian Process Regression, Exponential Gaussian Process Regression, Rational Quadratic Gaussian Process Regression. The second category (Classification Learner) includes Gaussian, Naive Bayes, K-nearest neighbors. The results show that Regression Learner methods are more effective in predicting the price of selected stocks.
Keywords: Statistical Learning Algorithm, Artificial Neural Network, Data Analysis, Capital Market, Prediction -
Pages 299-312
The adaptive fuzzy neural inference system (ANFIS) is an efficient estimation model not only among fuzzy neural systems but also among other types of machine learning techniques. Despite its acceptance among researchers, ANFIS cited limitations such as inefficiencies in large data and data problems, cost of computation, processing time and optimization, and error training. The ANFIS structural design is a complex optimization problem that can be improved using meta-heuristic algorithms. In this study, to optimize and reduce errors, a new meta-heuristic algorithm inspired by nomadic migration was designed and used to design an adaptive fuzzy neural system called the Qashqai nomadic meta-heuristic algorithm. The results of the hypothesis test showed that the Qashqai optimization algorithm is not defeated by the genetic algorithm and particle swarm and works well in terms of convergence to the optimal answer. In this hybrid algorithm, random data set are first generated and then trained by designing a basic fuzzy neural system. Subsequently, the parameters of the basic fuzzy system were adjusted according to the modeling error using the meta-heuristic optimization algorithm of Qashqai nomads. The fuzzy nervous system with the best values was obtained as the final result.The main achievements of the study are:• Improving ANFIS accuracy using a novel meta-heuristic algorithm.• Fix and remove some problems and Limitations in the Anfis model, such as inefficiencies in large data, cost of computation, Answer accuracy, and reduce errors.• Comparing the proposed ANFIS+QA with some recent related work such as ANFIS+QA and ANFIS+Pso.
Keywords: Optimization, Adaptive Neural Fuzzy Inference System (ANFIS), Meta-heuristic Algorithm, Genetic Algorithm (GA), Particle swarm algorithm (PSO), Qashqai algorithm (QA)