فهرست مطالب
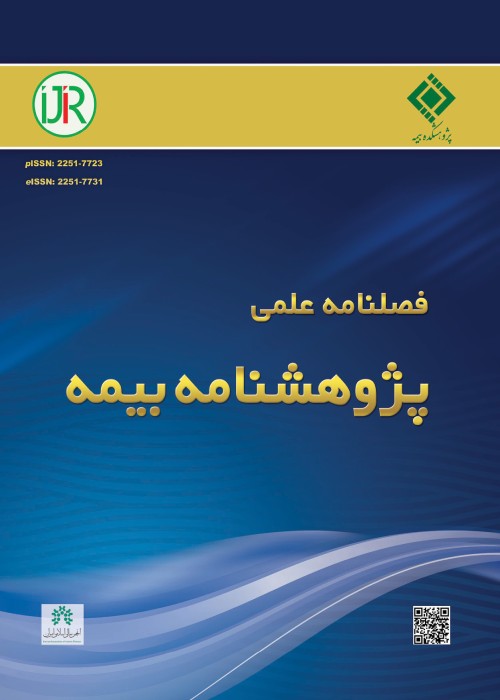
پژوهشنامه بیمه
سال سی و نهم شماره 1 (زمستان 1402)
- تاریخ انتشار: 1402/10/05
- تعداد عناوین: 6
-
-
صفحات 1-14پیشینه واهدافصنعت بیمه درمانی در پیش بینی هزینه های بیمه افراد که براساس پارامترهای پیچیده ای مانند سن و ویژگی های فیزیکی است، با چالش مهمی مواجه است. شرکت های بیمه برای مدیریت ریسک و جلوگیری از زیان احتمالی، بیمه گذاران را به دو گروه پرخطر و کم خطر دسته بندی می کنند. بااین حال، برآورد دقیق هزینه ها برای هر فرد می تواند کار سختی باشد. برای مقابله با این چالش، ما رویکردی مبتنی بر علم داده و یادگیری ماشین را پیشنهاد می کنیم که از یادگیری جمعی برای پیش بینی افراد پرخطر و کم خطر استفاده می کند.روش شناسی: روش پیشنهادی شامل مراحل مختلفی از جمله پیش پردازش داده ها، مهندسی ویژگی ها و اعتبارسنجی متقابل برای ارزیابی عملکرد مدل است. در مرحله اول، داده ها را با پاک کردن، مدیریت مقادیر ازدست رفته و رمزگذاری متغیرهای طبقه بندی، پیش پردازش می کنیم. در مرحله دوم، ما ویژگی های جدیدی را با استفاده از روش های مهندسی ویژگی ها مانند مقیاس بندی، نرمال سازی و کاهش ابعاد تولید می کنیم. این روش ها به استخراج اطلاعات معنادار از داده ها و بهبود عملکرد مدل کمک می کند. در مرحله بعد، ما از یادگیری جمعی برای ترکیب روش های رگرسیون متعدد، مانند رگرسیون لجستیک، شبکه های عصبی، ماشین های بردار پشتیبانی، جنگل های تصادفی، LightGBM و XGBoost استفاده می کنیم. هدف از ترکیب این روش ها این است که از نقاط قوت آن ها استفاده کنیم و نقاط ضعف آن ها را به حداقل برسانیم تا به دقت پیش بینی بهتری دست یابیم. در نهایت، عملکرد مدل را با استفاده از روش اعتبارسنجی متقاطع k-fold ارزیابی می کنیم. این روش به اعتبارسنجی دقت مدل و جلوگیری از برازش بیش از حد کمک می کند.یافته هارویکرد پیشنهادی ما به AUC برابر با 73/0 دست می یابد که اثربخشی آن را در پیش بینی افراد پرخطر و کم خطر نشان می دهد.نتیجه گیریبا استفاده از علم داده و روش های یادگیری ماشین، شرکت های بیمه می توانند دقت برآورد هزینه خود را بهبود بخشند و ریسک را بهتر مدیریت کنند. این رویکرد می تواند به شرکت های بیمه کمک کند تا پوشش بیمه ای و قیمت گذاری دقیق تری را برای افراد ارایه دهند که به رضایت بیشتر مشتریان و کاهش زیان های مالی منجر می شود.کلیدواژگان: داده کاوی، ریسک، هزینه بیمه درمان، یادگیری جمعی، یادگیری ماشین
-
صفحات 15-28پیشینه واهدافارزیابی صحیح و علمی ریسک صدور بیمه نامه یکی از حساس ترین و مهم ترین مراحل ارزیابی ریسک است و انجام آن باعث شناسایی مشتریان پرریسک و تعیین نرخ بیمه نامه، متناسب با ریسک مشتریان و در نتیجه پوشش مناسب خسارت های مالی ادعاشده به وسیله حق بیمه های دریافتی می شود. در این پژوهش روشی جدید برای تبیین دقیق تر و کاربردی تر از ریسک فاکتور ارایه شده است. در این روش که مبتنی بر الگوریتم بدون نظارت خوشه بندی است، ابتدا بازه های مختلف هر عامل موثر بر خسارت بررسی و با توجه به میزان تاثیرگذاری بر سطوح خسارت مشتریان به چند ریسک فاکتور تقسیم می شوند. سپس با توجه به میزان ارتباط آن با بازه دیگر عوامل، از لحاظ ایجاد سطوح خسارت مشابه در مشتریان، با آن ها ترکیب می شود و پکیجی شامل بازه های عوامل تاثیرگذار بر سطوح مختلف خسارت را تشکیل می دهد. به این ترتیب به جای یک ریسک فاکتور، پکیج های مختلفی ایجاد می شود که هرکدام از آن ها یک عامل ریسک یا همان ریسک فاکتور در نظر گرفته می شوند.روش شناسی: با استفاده از روش خوشه بندی کا-میانگین، بیمه گذاران به خوشه هایی با ریسک همگن که در واقع ریسک پکیج های متناظر با میزان پرخطر بودن مشتریان هستند، تقسیم شده اند. براساس ساختار الگوریتم کا-میانگین تعداد خوشه های مورد نظر باید از پیش تعیین شود. این موضوع چالش اصلی استفاده از الگوریتم مزبور است. در همین راستا دو رویکرد اصلی اعتبارسنجی سایه نما (ضریب سیلویت) و روش آرنج برای حل این مشکل ارایه شده است.یافته هابا توجه به نمودار آرنج و ضریب سیلویت و همچنین در نظر گرفتن نیاز شرکت های بیمه به ارزیابی کاربردی و منطبق بر واقعیت، 4 خوشه به دست آمد که با توجه به اینکه خوشه 2 و 3 در یک طیف نزدیک به هم و در نتیجه قابل پیوستن به یکدیگر هستند و خوشه با سطح ریسک متوسط را تشکیل می دهند، 3 خوشه به عنوان بهترین خروجی دسته بندی بیمه گذاران لحاظ شد.نتیجه گیریاز بررسی ویژگی های به دست آمده در 3 خوشه مطرح شده می توان پکیج های ریسک ذیل را معرفی کرد.افراد با سنین بالا، متوسط و پایین (چگال در بازه 30 تا 58 سال) با ماشین ارزان قیمت و دارای جنسیت مرد را می توان به عنوان بیمه گذاران با بالاترین سطح ریسک معرفی کرد.افراد با سنین متوسط و بالا (چگال در بازه 32 تا 53 سال) با ارزش ماشین متوسط و بالا را می توان بیمه گذاران دارای ریسک های متوسط در نظر گرفت.افراد با سنین متوسط به بالا (چگال در بازه 51 تا 63 سال) با ماشین گران قیمت را می توان بیمه گذاران با پایین ترین سطح ریسک در نظر گرفت.کلیدواژگان: الگوریتم بدون نظارت، خوشه بندی، ریسک پکیج، شخص ثالث
-
صفحات 29-42پیشینه واهدافاستفاده از شیوه های نوین بازاریابی در کسب وکارهای امروزی به شدت مورد نیاز تمام سازمان هاست. یکی از مسایل مهم در حوزه حفظ و نگهداشت مشتری ارزش طول عمر مشتری است. ارزش طول عمر مشتری تاثیر زیادی در بهینه سازی عملکردهای شرکت ها، از جمله شرکت های بیمه دارد. هدف از اجرای این پژوهش، شناسایی عوامل اثرگذار بر افزایش ارزش طول عمر مشتری شرکت های بیمه است.روش شناسی: این پژوهش از نوع پژوهش های آمیخته با رویکرد کیفی و کمی است که از نظر هدف، کاربردی و از لحاظ نحوه گردآوری داده، از نوع مطالعات پیمایشی است. جامعه آماری پژوهش، مدیران و کارشناسان شرکت ها و سازمان های بیمه، خبرگان و اساتید دانشگاهی بودند که با استفاده از روش نمونه گیری گلوله برفی انتخاب شدند. در بخش کیفی ابزار گردآوری اطلاعات، مصاحبه و در بخش کمی پرسش نامه بود که برای شناسایی مقوله ها، از مصاحبه نیمه ساختاریافته و از پرسش نامه به منظور اعتباریابی الگو استفاده شد. در بخش کیفی روش تحلیل داده ها، رویکرد نظریه داده بنیاد با روش استراوس و کوربین بود که با استفاده از نرم افزار MAXQDA و با استفاده از روش کدگذاری تدوین شد و در بخش کمی، روش تحلیل بر مبنای آزمون همبستگی کندال بود. به منظور بررسی روایی پژوهش بخش کیفی، از مدل کرسول به همراه روایی محتوایی و پایایی درون کدگذار و میان کدگذار و در بخش کمی به منظور آزمون روایی پژوهش از روایی اعتبار محتوا و بازآزمون بهره گرفته شد.یافته هادر پژوهش حاضر پس از تجزیه وتحلیل داده های به دست آمده در فرایند پژوهش در آخر تعداد 9 عامل علی، 3 عامل راهبردی، 4 عامل مداخله گر، 3 عامل زمینه ای و در نهایت پیامدهای افزایش ارزش ویژه مشتری در صنعت بیمه شناسایی شدند.نتیجه گیریپژوهش شامل ارایه مدلی مشتمل بر شرایط علی، زمینه ای و مداخله گر، به همراه معرفی پدیده محوری و ارایه راهبردهای افزایش ارزش طول عمر مشتری و شناسایی پیامدهای آن است.کلیدواژگان: ارزش طول عمر مشتری، بیمه، شرکت های بیمه، مدیریت ارتباط با مشتری
-
صفحات 43-60پیشینه واهدافتوزیع وایبول که فیزیک دانی سویدی به نام وایبول معرفی کرد، امروزه رایج ترین مدل مورد استفاده در مطالعات قابلیت اطمینان، طول عمر، کنترل کیفیت و به طور وسیعی در شاخه های مختلف علوم از جمله بیمه، پزشکی و مهندسی استفاده می شود. این توزیع برای الگوسازی داده های مختلف، انعطاف پذیری زیادی دارد. هدف اصلی این پژوهش محاسبه حق بیمه و برآورد پارامترهای توزیع وایبول با استفاده از روش های مختلف برآورد استروش شناسی: در این مقاله به برآورد پارامترهای توزیع وایبول و حق بیمه خالص با استفاده از برآودگرهای گشتاوری، ماکسیمم درست نمایی، کمترین توان های دوم خطا ، کمترین توان های دوم وزنی، صدکی، کرامر- فون- میسز، آمیخته گشتاوری و ماکسیمم درست نمایی در حضور داده های پرت پرداخته شده است. از نرم افزار R برای شبیه سازی و محاسبات عددی و از نرم افزار Easyfit برای برازش توزیع وایبول به داده های مثال واقعی استفاده شد. در پایان دو مثال واقعی برای به دست آوردن برآوردگرهای متفاوت با حق بیمه درصورتی که دو پارامتر β و θ مجهول و α معلوم است، ارایه شده است.یافته هادر این پژوهش اریبی، میانگین توان دوم خطای حق بیمه خالص و پارامترهای مجهول β و θ با استفاده از برآوردگرهای مختلف برای داده های توزیع وایبول و همچنین واریانس تعمیم یافته پارامترهای مجهول β و θ به دست آمد.نتیجه گیریدر این قسمت به بررسی و مقایسه برآوردگرها با استفاده از داده های واقعی و شبیه سازی شده پرداخته شد که برای داده های واقعی در روش های مختلف k-max متفاوت به دست آمد. برای نمونه، در روش گشتاوری k-max برابر 5 شد که براساس آن حق بیمه خالص 3.37657 است. در داده های شبیه سازی شده نیز با توجه به k (تعداد داده های دورافتاده)، n (حجم نمونه) و مقادیر β و θ، مقادیر اریبی، میانگین توان دوم خطا و واریانس تعمیم یافته حق بیمه و برآوردگرهای مختلف به دست آمد که برای نمونه برای n=10، k=1، β=1.5، θ=3 و70 α= با مقایسه اریبی و واریانس تعمیم یافته برآوردگرها به این نتیجه می رسیم که براساس اریبی، برآوردگر صدکی دارای عملکرد بهتری نسبت به دیگر برآوردگرهاست، یعنی دارای اریبی کمتری است و با توجه به واریانس تعمیم یافته، برآوردگر ماکسیمم درست نمایی دارای عملکرد بهتری نسبت به سایر برآوردگرهاست و برآوردگرها سازگارند (با افزایش حجم نمونه واریانس تعمیم یافته کاهش می یابد). براساس میانگین توان دوم خطا، برآورد گشتاوری دارای عملکرد بهتری نسبت به سایر برآوردگرهاست.کلیدواژگان: برآورد، توزیع وایبول، حق بیمه خالص، داده های دورافتاده
-
صفحات 61-70پیشینه واهدافسود آوری یکی از مهم ترین شاخص های عملکرد شرکت های بیمه است، زیرا نشان دهنده توانایی بیمه گر (شرکت بیمه) برای سرمایه گذاری و رشد آن است و ناظران با تکیه بر ویژگی های مالی در رابطه با سودآوری، بقای بیمه گر را تعیین می کنند. در سال های اخیر، محققان از روش تحلیل پوششی داده ها (DEA) به طور گسترده ای برای ارزیابی عملکرد انواع مختلف شرکت های بیمه استفاده کرده اند. سودآوری نیز به دلیل کاستی نسبت های مالی متعارف در بسیاری از مطالعات، با روش های تحلیل پوششی داده ها اندازه گیری می شود. با توجه به رشد سریع صنعت بیمه در کشور ایران و ضرورت بررسی بیشتر سودآوری صنعت بیمه اموال (غیرزندگی) در ایران و گسترش تحلیل پوششی، تحقیق حاضر انجام شده است.روش شناسی: این مطالعه به بررسی تحول و عوامل تعیین کننده سودآوری 18 شرکت بیمه پذیرفته شده در بورس اوراق بهادار تهران در سال های 1393 تا 1400 می پردازد، اطلاعات این شرکت ها در دوره پژوهش کامل و در دسترس هستند. در این مطالعه سودآوری به وسیله تحلیل پوششی داده ها اندازه گیری می شود. علاوه بر این، مدل برآوردگر توبیت نیز برای مطالعه و بررسی تاثیر اندازه شرکت، سن شرکت و تنوع محصول بر سودآوری بیمه گران استفاده می شود تا فرضیه ها آزمون و بررسی شود..یافته هانتایج تجربی اهمیت ترتیب مناسب هزینه ها و درآمدها برای بیمه گر را نشان می دهد؛ همچنین نتایج نشان می دهد که اندازه شرکت، سن شرکت و تنوع محصول با سودآوری رابطه معناداری ندارند.نتیجه گیریشرکت های بیمه باید ساختار مخارج و انواع مختلف کسب و کار خود را به درستی ترتیب دهند. ترکیب اشتباه مخارج ورودی و درآمد خروجی به شکست در دستیابی به حداکثر نسبت سود منجر می شود. ازاین رو، ازیک سو، ساختار هزینه های یک شرکت بیمه باید بهینه شود. از سوی دیگر، به جای اتکای بیش از حد بر عملکرد ادغام ریسک و ریسک، باید انواع مختلف کسب و کار را در نظر گرفت. این مطالعه به درک بهتر عملکرد شرکت های بیمه کمک می کند تا موقعیت نسبی سودآوری خود را در صنعت درک کند و نیز راهبردهای هدفمند را تدوین کند، و برای دولت کمک کننده است که درک بهتری از توسعه صنعت داشته باشد و سیاست های مربوطه را تدوین کند.کلیدواژگان: تحلیل پوششی داده ها، رگرسیون توبیت، صنعت بیمه ایران، شاخص تغییر نسبت سود
-
صفحات 71-86پیشینه واهدافبا توجه به رشد روزافزون فناوری اطلاعات و کسب وکارهای مبتنی بر داده و روند رو به رشد اطلاعات در پایگاههای داده، صنعت بیمه نیز به عنوان یکی از کسب وکارهای یادشده با حجم انبوهی از اطلاعات ذخیره شده روبه رو است. این حجم انبوه دادهها شرکتهای بیمهای را ملزم میسازد تا با شیوههای دادهکاوی، دانش موجود در پایگاههای داده را کشف کنند. ازسوی دیگر توسعه روشهای نوین دادهکاوی و کشف الگوهای پنهان در پایگاههای داده به سرعت رو به افزایش است.روش شناسی: در این مقاله با هدف بررسی جامع بر کاربرد دادهکاوی در صنعت بیمه دنیا، جدیدترین مستندات علمی مربوطه در چهار سناریوی مختلف شامل تحقیقات صنعت بیمه، دادهکاوی در بیمه، کشف تخلفات و ریسک در بیمه از وب سایت علمی Web of Science دریافت و با رویکرد علمسنجی تحلیل و بررسی شده است. نتایج به دست آمده نشان میدهد رشد دادههای موجود در شرکتهای بیمه و شناسایی ریسک و فاکتورهای آن، شرکتهای بیمه و پژوهشگران را به بررسی روشهای نوین مدیریت پایگاههای داده و دادههای بزرگ و همچنین شناسایی ریسک و فاکتورهای آن با استفاده از شیوه های دادهکاوی ملزم میسازد.یافته هابدین منظور برای تسهیل در تحقیقات پژوهشگران آینده و استفاده از مطالعات پیشین دادهکاوی در صنعت بیمه، در این مقاله شبکه هم استنادی کشورهای مختلف و معتبرترین مجلات علمی براساس شاخص ارزیابی استناد به مقالات معرفی شده است.نتیجه گیریدر نتایج این مقاله فهرست مجلات معتبر علمی و موضوعاتی را که در هریک به طور تخصصی به آن پرداخته شده، ارایه کرده ایم تا مرور ادبیات جامع در این مقاه مروری مسیر مطالعات آینده صنعت بیمه را هموارتر سازد. همچنین رتبه بندی برترین کشورهای جهان که متمرکز بر مطالعات داده کاوی در صنعت بیمه بوده است، آورده شده و رتبه ایران در میان سایر کشورها نیز مشخص شده است. استفاده از مطالعات و مجلات علمی معتبر می تواند راه پژوهش های آینده را در پیشرفت این مطالعات در صنعت بیمه ایران را هدفمندتر کند.کلیدواژگان: شناسایی ریسک، صنعت بیمه، علمسنجی، کشف تخلفات
-
Pages 1-14BACKGROUND AND OBJECTIVESThe healthcare insurance industry faces a significant challenge predicting individuals' insurance costs, which are based on complex parameters such as age and physical characteristics. Insurance companies categorize policyholders into high-risk and low-risk groups to manage risks and avoid potential losses. However, the accurate estimation of costs for each individual can be a daunting task. By leveraging data science and machine learning techniques, insurance companies can improve their cost estimation accuracy and better manage risks. This approach can help insurance companies to provide more accurate insurance coverage and pricing for individuals leading to higher customer satisfaction and lower financial losses.METHODSTo address this challenge, a data science and machine learning-based approach that uses ensemble learning to predict high-risk and low-risk individuals is used. The method involves several steps including data preprocessing, feature engineering, and cross-validation to evaluate the model's performance. The first step involves preprocessing the data by cleaning it, handling missing values, and encoding categorical variables. The second step generates new features using feature engineering techniques such as scaling, normalization, and dimensionality reduction. Next, ensemble learning is used to combine multiple regression methods such as logistic regression, neural networks, support vector machines, random forests, LightGBM, and XGBoost. By combining these methods, the aim is to leverage their strengths and minimize their weaknesses to achieve better prediction accuracy. Finally, the model's performance is evaluated using cross-validation techniques such as k-fold cross-validation. These techniques help to validate the model's accuracy and prevent overfitting.FINDINGSThe proposed approach achieves an AUC of 0.73 demonstrating its effectiveness in predicting high-risk and low-risk individuals.CONCLUSIONIn conclusion, the healthcare insurance industry can benefit greatly from data science and machine learning-based approaches. By accurately predicting high-risk and low-risk individuals, insurance companies can better manage risks and provide more accurate coverage and pricing for their customers. This can lead to the improvement of customer satisfaction and the reduction of financial losses for insurance companies.Keywords: Data mining, Ensemble learning, Healthcare insurance cost, Machin learning, risk
-
Pages 15-28BACKGROUND AND OBJECTIVESThe accurate and scientific assessment of the risk to issue an insurance policy is one of the most critical and important stages of risk assessment frameworks. This leads companies to identify high-risk customers and determine the policy rates in accordance with their risks, and as a result, the claims will be covered appropriately through the insurance premiums. In this paper, a new method is presented to define the concept of risk factor in more practical, flexible and accurate way. In this method, which is based on an unsupervised clustering algorithm, initially, every single factor is examined based on different ranges and their corresponding impact on customer loss levels. Then, considering their connection with the ranges of other factors in terms of creating similar levels of customer loss, they are combined to form a package. Thus, different packages are created, each of which is considered a risk factor and comprise the ranges of factors affecting different levels of loss.METHODSThe k-means clustering method was used to divide insurers into clusters with similar risks, which correspond to the risk packages associated with the customers' risk level. The number of desired clusters should be determined in advance, which is the main challenge of using this algorithm. Two main approaches for validation, namely the silhouette score and the elbow method, were presented.FINDINGSBased on the elbow plot and silhouette coefficient, as well as considering the practical and realistic evaluation needed by insurance companies, four clusters were obtained. Cluster 2 and 3 are similar and can be merged to form a cluster of medium risk level. Therefore, three clusters were considered the best outcome for categorizing insurance policyholders.CONCLUSIONThe risk packages can be introduced from the examination of the 3 clusters including People with high, medium and low age (confidence interval) with low price car whose gender is male can be introduced as the highest level of risk; People with medium and high ages (confidence interval) with medium and high car prices can be considered as medium risks, and Middle-aged and older people (confidence interval) with expensive cars were considered the lowest level of risk. From the results of these risk packages, it can be concluded that although a significant population of older policyholders falls into the first package (first cluster), they have the highest level of risk. On the other hand, the older people in the third package (even though their average age is the highest among the clusters) have the lowest level of risk. Another important point is that the risk level decreases as income increases simultaneously with age.Keywords: Clustering, Risk package, Third Party, Unsupervised algorithm
-
Pages 29-42BACKGROUND AND OBJECTIVESThe use of new marketing techniques in today's businesses is highly needed by all organizations. One of the important issues in the field of customer retention is the customer's lifetime value. Customer lifetime value has a great impact on optimizing the performance of companies, including insurance companies. The purpose of this research is to identify the influencing factors on increasing the customer lifetime value of insurance companies.METHODSThis research is a type of mixed research with a qualitative and quantitative approach, which is a survey study in terms of its purpose, application, and in terms of data collection. The research population includes managers, experts, and university professors from insurance companies and organizations. who were selected using the snowball sampling method. In the qualitative part, the data collection tool was an interview, and in the quantitative part, a questionnaire was used to identify the categories, and a semi-structured interview was used, and a questionnaire was used to validate the model. In the qualitative part of the data analysis method, the data theory approach was based on the Strauss and Corbin method, which was compiled using MAXQDA software and using the coding method, and in the quantitative part, the analysis method was based on Kendall's correlation test. In order to examine the validity of the research in the qualitative part, the Cressol model was used along with content validity and intra-coder and inter-coder reliability, and in the quantitative part, in order to test the validity of the research, content validity and retest validity were used.FINDINGSThe findings of the study identify 9 causal factors, 3 strategic factors, 4 intervening factors, and 3 contextual factors that contribute to increasing customer lifetime value in the insurance industry.CONCLUSIONThe conclusion of the research is the presentation of a model that includes causal, contextual, and intervening conditions, along with strategies to increase customer lifetime value and their consequences in the insurance industry.Keywords: Customer Lifetime Value, Customer Relationship Management, Insurance, Insurance Companies
-
Pages 43-60BACKGROUND AND OBJECTIVESWeibull distribution, introduced by a Swedish physicist named Weibull, is the most common model used in studies of reliability, longevity, quality control. It is widely used in various fields of science including insurance, medicine, and engineering . This distribution is flexible enough to model different data. The main goal of this research is to calculate insurance premiums and estimate Weibull distribution parameters using various estimation methods.METHODSIn this article, the parameters of Weibull distribution and net premium have been estimated using moment estimation, maximum likelihood, least squares of error, weighted least squares, percentage, Cramer-Von- Mises, mixture of moment and maximum-likelihood against outliers. R software was used for simulation and numerical calculations purposes and also Easyfit software was used to fit Weibull distribution to the real example data. In the end, two real data examples for obtaining various estimators of the premium in case of unknown parameters β and θ and known α are presented.FINDINGSIn this research bias, the mean square error of net premium and unknown parameters β and θ were obtained using different estimators for Weibull distribution data as well as the generalized variance of unknown parameters β and θ.CONCLUSIONIn this part, the evaluation and comparison of the estimators using real and simulated data was done, which was obtained by different for real data. For example, in the moment method, was equal to 5, based on which the net premium is 3.37657. In the simulated data, according to k (number of outliers), n (sample size) and β and θ values, bias values, mean squared error and generalized variance of premium and different estimators were obtained. As an example, for n=10, k=1, β=1.5, θ=3 and α=70, by comparing the bias and generalized variance of the estimators, we come to the conclusion that based on the bias, the percentile estimator has a better performance than the other estimators. Simply put, it has less bias and according to the generalized variance, the maximum likelihood estimator has a better performance than other estimators and the estimators are consistent (the generalized variance decreases with the increase of the sample size). Based on the mean square error, the moment estimation has a better performance than other estimators.Keywords: Estimation, Net premium, Outliers, Weibull distribution
-
Pages 61-70BACKGROUND AND OBJECTIVESProfitability is a crucial indicator of insurance company performance, as it reflects their ability to invest and grow. Supervisors also rely on financial characteristics, including profitability, to determine the viability of insurers. The data envelopment analysis (DEA) method has been widely used to evaluate the performance of insurance companies. However, conventional financial ratios are often lacking in such studies, making DEA an effective alternative for measuring profitability. Given the rapid growth of the insurance industry in Iran, this research aims to examine the profitability of non-life insurance companies in Iran and expand coverage analysis.METHODSThe study focuses on 18 insurance companies listed on the Tehran Stock Exchange from 2013 to 2014, with complete and available information during the research period. Profitability is measured using DEA, and the Tobit estimator model is used to investigate the impact of company size, company age, and product variety on profitability.FINDINGSThe results highlight the importance of properly managing expenses and incomes for insurers. Additionally, the study finds that company size, company age, and product variety do not have a significant relationship with profitability.CONCLUSIONInsurance companies need to effectively organize their expenses and various types of business to maximize profit ratios. Optimizing cost structures and diversifying business ventures are key strategies for achieving profitability. This research provides insights into insurance company performance, allowing for a better understanding of their relative profitability within the industry and the formulation of targeted strategies. It also aids the government in developing policies that support the industry's growth.Keywords: Data Envelopment Analysis, IRAN insurance industry, Profit ratio change index, Tobit regression
-
Pages 71-86BACKGROUND AND OBJECTIVESData mining is known as a process of discovering patterns in large datasets through a combination of statistical tools and techniques. In recent years, data mining and its applications in different businesses have increasingly grown. Insurance industry is one of the data-driven businesses whose survival is so dependent on satisfying customers besides achieving the highest benefit. Information or data is a vital asset of the insurance industry ;accordingly, using data mining techniques to discover patterns behind large datasets is a need. Having seen the increasingly high rate of information technology and recorded data in data-driven businesses, lots of industries like the insurance industry have been urged to use state-of-the-art data mining techniques to turn raw data into useful information using Big Data Analytics.METHODSLooking at the current research on data mining applications in the insurance industry proves the fact that we should recognize the state-of-the art techniques in data mining and set new strategies to focus on Big Data Analytics more. Big Data Analytics consists of the algorithms which are more efficient and less time-consuming so it can help to identify patterns and rules in complex datasets. For this purpose, this paper presents a comprehensive literature review regarding the usage of data mining techniques in the insurance industry by the scientometrics approach. For this purpose, first we searched and gathered bibliometrics files of recent researches from Web of Science and Scopus into four different scenarios. In each scenario, we looked up for different keywords regarding “Data Mining”, “Insurance Industry”, and “Risk Management” to make sure that all the results would be specifically focused on the research topic. Then, we used R programming software to analyze the results of each scenario based on keywords co-occurrence in the given research.FINDINGSThe results of keywords co-occurrence and a word cloud of recent research confirm that insurance companies should focus on Big Data Analytics instead of traditional data processing to get information systematically from too large or complex datasets. Big Data Analytics has been used for several years, but in recent years many data-driven businesses, like the insurance industry, have used its techniques associated with risk and risk factor identification. Risk management in the insurance industry has been widely considered in recent researches. Therefore, in this paper, some high-ranked journals and the most significant researches have been identified and recommended in order to pave the way for future researches in this field.CONCLUSIONWe hope that the comprehensive literature review provided in this paper can help the researchers to focus on the relative journals and researches published then get into more details. For this purpose, the lists of all journals and conferences besides the most cited researches are provided in the experimental section of this paper. Also, the ranking list of different countries from all around the world related to data mining and Big Data Analytics in the insurance industry is presented. The results show that Iran is the 15th country that uses data mining techniques and it is the 17th country in the world focusing on risk management in the insurance industry.Keywords: Data mining, fraud detection, Insurance Industry, Risk Management, Scientometrics