فهرست مطالب
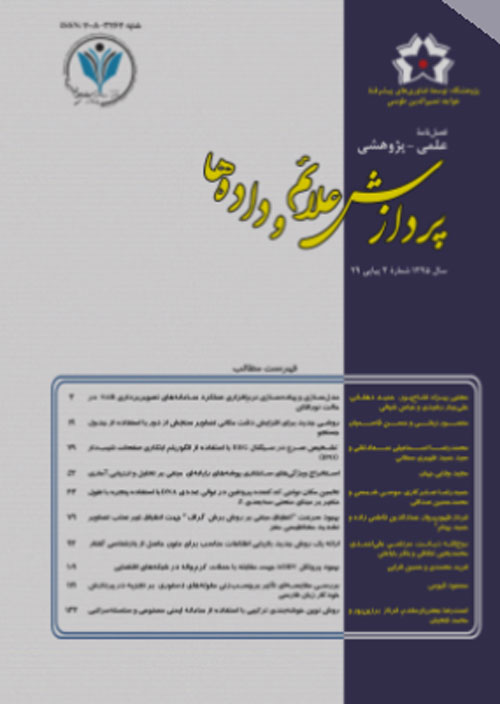
فصلنامه پردازش علائم و داده ها
سال شانزدهم شماره 3 (پیاپی 41، پاییز 1398)
- تاریخ انتشار: 1398/09/10
- تعداد عناوین: 10
-
-
صفحات 3-22
برون سپاری اجرا به عنوان یک راه حل مهم برای اجرای برنامه های کاربردی سنگین روی سامانه های تلفن همراه است. در سامانه های تلفن همراه تغییرات پهنای باند در دسترس، به طور معمول اتفاق می افتد که روی بخش بندی بهینه تاثیر می گذارد. به منظور اجتناب از تکرار این فرایند سنگین بخش بندی باید به صورت تطبیقی و یک بار انجام شده و با تغییرات پهنای باند سازگار باشد. در این مقاله با در نظر گرفتن تغییرات پهنای باند و محدودیت تبادل داده، مساله بخش بندی تطبیقی و برون سپاری اجرای کاربردهای مبتنی بر خدمات وب به صورت سه مدل جداگانه با اهداف متفاوت شامل بهینه سازی زمان اجرا، بهینه سازی مصرف انرژی و بهینهسازی ترکیب وزن دار زمان اجرا و مصرف انرژی، فرموله شده و روشی ابتکاری مبتنی بر الگوریتم ژنتیک برای حل هر مساله بهینه سازی در زمان معقول ارائه شده است. نتایج شبیه سازی و ارزیابی الگوریتم پیشنهادی نشان می دهدکه در مقابل تغییرات پهنای باند در دسترس سامانه سیار، عملکرد الگوریتم ارائه شده به نحو قابل ملاحظه ای بهتر از کارهای مشابه است.
کلیدواژگان: برون سپاری اجرا، بخش بندی، تطبیق با پهنای باند، خدمات وب، محدودیت تبادل داده -
صفحات 23-36
عدم دقت در طراحی دستورهای مستقل از متن و استفاده از ساختارهای نامناسب مانند فرم نرمال چامسکی به خودی خود می تواند عملکرد تجزیه گرهای آماری مستقل از متن را تضعیف کند. در این پژوهش ساختار ترکیبات عطفی درخت بانک فارسی را مورد بررسی قرار دادیم. نتایج حاصل از این پژوهش نشان می دهد که با اضافه کردن وابستگی های ساختاری به دستورهای مستقل از متن و اصلاح قواعد اولیه، می توان از ترکیبات عطفی رفع ابهام کرد و صحت عملکرد تجزیه گر دستور مستقل از متن آماری را افزایش داد. فرض استقلال ضعیف، یکی از مشکلات مربوط به دستورهای مستقل از متن است که سعی شده است تا با تزریق وابستگی های ساختاری از طریق نشانه گذاری گره های والد و فرزند مرتفع شود. تاثیر ریزدانگی و درشت دانگی برچسب های اجزای واژگانی کلام و همین طور ادغام ناپایانه ها بر تجزیه گر دستور مستقل از متن آماری فارسی از جمله موارد مورد بررسی قرار گرفته شده در این پژوهش است.
کلیدواژگان: دستور مستقل از متن آماری، تجزیه گر، ترکیبات عطفی، نشانه گذاری قواعد، برچسب اجزای واژگانی کلام -
صفحات 37-48
همانند بسیاری از زمینه های دیگر زبان شناسی محاسباتی، ارزیابی نقش مهمی در سامانه های پرسش و پاسخ تعاملی ایفا می کند. با این وجود، در زمینه ارزیابی سامانه های پرسش و پاسخ تعاملی به طورتقریبی هیچ روش خاصی وجود ندارد که به ارزیابی کلی این سامانه ها پرداخته و همواره انسان باید در فرآیند ارزیابی مشارکت داشته باشد. ارائه مدلی که بتواند جایگزین انسان در فرآیند ارزیابی شود، یکی از موضوعات مورد توجه در این حوزه است. در این مقاله، یک مدل آماری مناسب برای ارزیابی سامانه های پرسش و پاسخ تعاملی جهت جایگزین کردن به جای انسان توسط مجموعه ای از ویژگی های جدید و رگرسیون ارائه شده است. با استفاده از چهار سامانه تعاملی موجود پایگاه داده ای مناسب ایجاد شد. تعداد 540 نمونه به عنوان داده مناسب در نظر گرفته شد تا مجموعه آزمون و آموزش بر اساس آن تشکیل شود. ابتدا پیش پردازش بر روی مکالمات صورت پذیرفت و بر اساس روابط تعریف شده، ویژگی های آماری از متن مکالمه ها استخراج و بر اساس آن ماتریس ویژگی تشکیل و سپس با استفاده از انواع رگرسیون سعی شد تا بهترین مدل استخراج شود که در نهایت رگرسیون غیرخطی سری توانی با RMSE به میزان 13/0 بهترین مدل را ارائه کرد.
کلیدواژگان: ارزیابی، سامانه پرسش و پاسخ تعاملی، رگرسیون غیرخطی، استخراج ویژگی -
صفحات 49-60
این مقاله فرایند طراحی الگویی را گزارش می کند که برای حاشیه گذاری ساخت وابستگی زبان فارسی به عنوان بخشی از پروژه تهیه درخت بانک وابستگی برای زبان فارسی تدوین شده است. این الگو بر توصیفی جامع از نحو زبان فارسی بنا بر نظریه ای تحت عنوان نظریه گروه های خودگردان پایه گذاری شده است. تاکید عمده در نظریه بالا بر ضرورت توجه به اهمیت مفهوم گروه در تحلیل های وابستگی به علت واقعیت شناختی آن و مفهوم ظرفیت نیز در آنجا فراتر از فعل گسترده شده است. بر آن مبنا، هر وابسته هر نوع هسته به عنوان متمم یا افزوده طبقه بندی می شود؛ به علاوه، برای اینکه الگوی حاصل آن طور که باید برای مخاطبان احتمالی مفهوم باشد، جدیدترین الگوی حاشیه گذاری معیار موجود، موسوم به وابستگی های جهانی، متناسب با نیازهای چهارچوب اتخاذی مطابقت یافته است. نتیجه، مجموعه برچسبی است متشکل از پنجاه و سه رابطه وابستگی، شامل پانزده برچسب جدید در کنار برچسب هایی که از وابستگی های جهانی وام گرفته شده است.
کلیدواژگان: حاشیه گذاری، درخت بانک، ظرفیت، فارسی، وابستگی های جهانی -
صفحات 61-78
سامانه های تشخیص حریق با توجه به قابلیت گسترش سریع و توانایی بالای آتش در تخریب، از اهمیت بالایی برخوردار هستند. روش های سنتی شناسایی آتش که بر مبنای اثرات ناشی از آتش مانند دود و حرارت هستند، در محیط های بزرگ و سرباز کارایی لازم را ندارند. هدف از این مقاله ارائه روشی کارآمد برای تشخیص آتش به کمک تصاویر ویدئویی در محیط های سرباز شهری با تمرکز بر انبار شرکت گاز با هزینه محاسباتی پایین است. در روش پیشنهادی از مولفه رنگ و ویژگی های زمانی و مکانی که خاصیت تفکیک کنندگی بالایی دارند، استفاده شده است. ابتدا نواحی نامزد آتش با کمک مدل رنگی انتخاب و سپس با توجه به تغییرات نامنظم پیوسته شعله آتش در قاب های متوالی و پراکندگی سطوح روشنایی کانال قرمز، نواحی آتش در تصاویر استخراج می شوند. آزمایش های انجام شده حاکی از آن است که روش پیشنهادی علاوه بر این که در نمونه ویدئوهای بررسی شده، قابلیت تشخیص صد درصدی وقوع آتش را در محیط های سرباز شهری دارد، زمان تشخیص را در شرایط مشابه نسبت به روش مقاله پایه 35/87 درصد کاهش داده است.
کلیدواژگان: تشخیص هوشمند وقوع آتش، تصاویر ویدئویی، پردازش تصویر، زمان تشخیص -
صفحات 79-88
امروزه با فراگیر شدن دسترسی به اینترنت و به خصوص شبکه های اجتماعی، امکان به اشتراکگذاری عقاید و نظرات کاربران فراهم شده است. از سوی دیگر تحلیل احساس و عقاید افراد می تواند نقش به سزایی در تصمیم گیری سازمان ها و تولیدکنندگان داشته باشد. از این رو وظیفه تحلیل احساس و یا عقیدهکاوی به زمینه پژوهشی مهمی در حوزه پردازش زبان طبیعی تبدیل شده است. یکی از چالش های استفاده از شیوههای یادگیری ماشینی در حوزه پردازش زبان طبیعی، انتخاب و استخراج ویژگی های مناسب از میان تعداد زیاد ویژگی های اولیه برای دست یابی به مدلی با صحت مطلوب است. در این پژوهش دو روش فشرده سازی براساس تجزیه های ماتریسی SVD و NMF و یک روش بر اساس شبکه های عصبی برای استخراج ویژگی های موثرتر و با تعداد کمتر در زمینه تحلیل احساس در مجموعه داده نظرات به زبان فارسی مورد استفاده و تاثیر سطح فشرده سازی و اندازه مجموعه داده در صحت مدلهای ایجاد شده مورد ارزیابی قرارگرفته شده است. بررسی ها نشان می دهد که فشرده سازی نه تنها از بار محاسباتی و زمانی ایجاد مدل کم می کند، بلکه می تواند صحت مدل را نیز افزایش دهد. بر طبق نتایج پیاده سازی، فشرده سازی ویژگی ها از 7700 ویژگی اولیه به دوهزار ویژگی با استفاده از شبکه عصبی، نه تنها باعث کاهش هزینه محاسسباتی و فضای ذخیره سازی میشود، بلکه می تواند صحت مدل را از % 05/77 به % 85/77 افزایش دهد. از سوی دیگر در مجموعه داده کوچک با استفاده از روش SVD نتایج بهتری به دست می آید و با تعداد ویژگی دوهزار می توان به صحت % 92/63 در مقابل % 57/63 دست پیدا کرد؛ هم چنین آزمایش ها حاکی از آن است که فشرده سازی با استفاده از شبکه عصبی در صورت بزرگی مجموعه داده برای ابعاد پایین مجموعه ویژگی، بسیار بهتر از سایر روش ها عمل می کند. به طوری که تنها با یکصد ویژگی استخراج شده با استفاده از فشرده ساز شبکه عصبی از 7700 ویژگی اولیه می توان به صحت قابل قبول % 46/74 در مقابل صحت اولیه % 05/77 با 7700 ویژگی دست یافت.
کلیدواژگان: پردازش زبان طبیعی، تحلیل احساس، کدگذار خودکار، تجزیه مقدار تکین، تجزیه نامنفی ماتریس -
صفحات 89-100
در سامانه های ردیابی هدف، از فیلتر ردیابی برای تخمین پیاپی و هموار موقعیت و سرعت هدف متحرک با کمینه خطا استفاده می شود. در این مقاله، روشی برای طراحی و پیاده سازی سخت افزاری فیلتر کالمن در چنین کاربردی ارائه شده است. روش پیشنهادی شامل یک پیاده سازی ممیز ثابت فیلتر روی FPGA است که در آن سرعت اجرای الگوریتم از طریق موازی سازی عملیات غیر وابسته بهبود یافته است. پس از طراحی بر اساس مساله داده شده، نسخه های ممیز شناور و ممیز ثابت فیلتر شبیه سازی و نسخه ممیز ثابت روی سخت افزار پیاده سازی شده است. برای ارزیابی کارایی فیلتر، داده های فاصله -سرعت یک هدف متحرک با مدل مناسب تولید و پس از چندی سازی و درآمیختن با اغتشاش به فیلتر اعمال می شوند. نتایج نشان می دهد که با انتخاب طول بیت مناسب، فیلتر پیاده سازی شده سریع و کارآمد بوده و با زمان اجرای حدود µs 4/0، موجب dB 11 کاهش در خطای تخمین فاصله شده و عملکردی نزدیک به نمونه ممیز شناور فراهم می آورد.
کلیدواژگان: فیلتر کالمن، پیاده سازی FPGA، ردیابی، تخمین فاصله، تخمین سرعت -
صفحات 101-116
در این مقاله با استفاده از روشی ساده، اما کارا سعی شده دامنه جستجوی زیرواژگان به شدت کاهش یابد. در گام آموزش، داده های آموزشی بر اساس موقعیت علائم گروه بندی می شوند، در گروه هایی که تعداد عناصر بیش از ده زیرواژه است، برای کاهش فضای جستجو با توجه به تعداد عناصر گروه، با استخراج ویژگی های ساده ای از پروفایل های افقی و عمودی خوشه بندی صورت می گیرد. در مرحله بازشناسی در نخستین مرحله با تعیین نسبت پهنا به ارتفاع زیرواژه (با علائم و بی علائم) و کد موقعیت نقاط و علائم، دامنه جستجو به زیرواژگانی با این کد موقعیت که در محدوده ای از نسبت های یاد شده باشند، محدود می شود؛ در صورتی که تعداد زیرواژگان محدود شده در این مرحله کمتر از ده باشد، این محدوده پذیرفته و در غیر این صورت در مرحله بعد با استخراج ویژگی های ساده ای از پروفایل های افقی و عمودی فضای جستجو به تعدادی از نزدیکترین خوشه ها به این زیرواژه که شرط نسبت پهنا به ارتفاع را نیز ارضا کنند محدود می شود. با اعمال روش پیشنهادی این مقاله فضای جستجو تا حد قابل قبولی کاهش یافته است.
کلیدواژگان: بازشناسی، زیرواژگان تایپی فارسی، کاهش فضای جستجو، موقعیت نقاط و علائم -
صفحات 117-128
طراحی و پیاده سازی ابزارهای پردازش زبان طبیعی فارسی، بر اساس ویژگی های خاص این زبان، همواره با چالش هایی مواجه است. با توجه به این که سامانه های تصحیح املای خودکار در حوزه های مختلفی از قبیل تصحیح پرس و جوها، بررسی املای واژگان در اینترنت و برنامه های ویراستاری متنی کاربرد دارد، لازم است تا برای زبان فارسی نیز نرم افزارهای مناسب ایجاد شود. در این مقاله ابتدا مقدمه ای در خصوص انواع خطاهای املایی، راه کارهای شناسایی و تصحیح خطاها شرح داده شده و سپس به معرفی سامانه پارسی اسپل که بر اساس معنای واژگان فارسی، خطاها را شناسایی و تصحیح می کند، می پردازیم. با توجه به نتایج حاصله از ارزیابی سامانه پارسی اسپل با سایر نرم افزارهای مشابه رایج، مشخص شد که سامانه پارسی اسپل به عنوان ابزار موثری جهت شناسایی و پیشنهاد واژه های صحیح برای خطاهای غیر واژه و واژه حقیقی است. در مراحل شناسایی و پیشنهاد، معیارF- به صورت معناداری بهبود یافته است. همچنین نتایج ارزیابی نشان داده که سامانه پارسی اسپل خطاهای واژه حقیقی بیشتری را شناسایی کرده و قادر به ارائه و پیشنهاد واژه های جایگزین صحیح، برای واژه های نادرست است و مقدار معیار بازخوانی در شناسایی خطای واژه حقیقی به صورت معناداری بیشتر از نرم افزارهای رقیب آن است.
کلیدواژگان: سیستم خطا یاب فارسی، تصحیح خطای واژگان، شناسایی خطای واژگان، پردازش زبان طبیعی، مدل زبان فارسی -
صفحات 129-148
پردازش تصویر روشی برای اعمال برخی عملیات ها بر روی یک تصویر است به طوری که با استفاده از آن، تصاویری با کیفیت بالاتر به دست آمده یا برخی اطلاعات مفید از تصویر استخراج می شود. الگوریتم های سنتی پردازش تصویر در شرایطی که تصاویر آموزشی (دامنه منبع) که برای یاددهی مدل استفاده می شوند، توزیع متفاوتی از تصاویر آزمایش (دامنه هدف) داشته باشند، نمی توانند عملکرد خوبی داشته باشند. با این حال، بسیاری از برنامه های کاربردی دنیای واقعی به علت کمبود داده های برچسب دار آموزشی دارای محدودیت هستند؛ از این رو از داده های برچسب دار دامنه های دیگر استفاده می کنند. به این ترتیب به خاطر اختلاف توزیع بین دامنه های منبع و هدف، طبقه بند یادگرفته شده براساس مجموعه آموزشی بر روی داده های آزمایشی عملکرد ضعیفی خواهد داشت. یادگیری انتقالی و انطباق دامنه، با به کارگیری مجموعه داده های موجود دو راه حل برجسته برای مقابله با این چالش هستند، و حتی با وجود اختلاف توزیع قابل ملاحظه بین دامنه ها می توانند دانش را از دامنه های مرتبط به دامنه هدف انتقال دهند. فرض اصلی در مساله تغییر دامنه این است که توزیع حاشیه ای یا توزیع شرطی داده های منبع و هدف متفاوت باشد. تطبیق دامنه به طور صریح با استفاده از معیار فاصله ازپیش تعیین شده تفاوت در توزیع حاشیه ای، توزیع شرطی یا هر دو توزیع را کاهش می دهد. در این مقاله، ما به یک سناریوی چالش برانگیز می پردازیم که در آن تصاویر دامنه های منبع و هدف در توزیع های حاشیه ای متفاوت بوده و تصاویر هدف دارای برچسب نیستند. بیش تر روش های قبلی دو استراتژی یادگیری تطابق ویژگی ها و وزن دهی مجدد نمونه ها را به طور مستقل برای تطبیق دامنه ها مورد بررسی قرار داده اند. در این مقاله، ما نشان می دهیم زمانی که تفاوت دامنه ها به طور قابل توجهی بزرگ باشد، هر دو استراتژی مهم و اجتناب ناپذیر هستند. روش پیشنهادی ما تحت عنوان تطبیق دامنه مبتنی بر نمونه برای طبقه بندی تصاویر (DAIC)، یک فرایند کاهش بعد بوده که با کاهش اختلاف توزیع تصاویر آموزشی و آزمایشی و به کارگیری هم زمان تطابق ویژگی ها و وزن دهی مجدد کارایی مدل را افزایش می دهد. ما با گسترش واگرایی برگمن غیرخطی برای اندازه گیری تفاوت توزیع حاشیه ای و اعمال آن به الگوریتم کاهش بعد آنالیز تفکیک خطی فیشر، از آن برای ساخت یک نمایش ویژگی موثر و قوی برای تفاوت های توزیع قابل ملاحظه بین دامنه ها استفاده می کنیم؛ همچنین، DAIC از مزیت برچسب گذاری اولیه برای داده های هدف به صورت تکرار شونده برای هم گرایی مدل استفاده می کند. آزمایش های گسترده ما نشان می دهد که DAIC به طور قابل توجهی بهتر از الگوریتم های یادگیری ماشین پایه و دیگر روش های یادگیری انتقالی در نه مجموعه داده بصری تحت سناریوهای مختلف عمل می کند.
کلیدواژگان: پردازش تصویر، یادگیری انتقالی، واگرایی برگمن، کاهش اختلاف توزیع حاشیه ای، کاهش ابعاد
-
Pages 3-22
Computation offloading is known to be among the effective solutions of running heavy applications on smart mobile devices. However, irregular changes of a mobile data rate have direct impacts on code partitioning when offloading is in progress. It is believed that once a rate-adaptive partitioning performed, the replication of such substantial processes due to bandwidth fluctuation can be avoided. Currently, a wide range of mobile applications are based on web services, which in turn influences the process of offloading and partitioning. As a result, mobile users are prone to face difficulties in data communications due to cost of preferences or connection quality. Taking into account the fluctuations of mobile connection bandwidth and thereby data rate constraints, the current paper proposes a method of adaptive partitioning and computation offloading in three forms. Accordingly, an optimization problem is primarily formulated to each of three main objectives under the investigation. These objectives include run time, energy consumption and the weighted composition of run time and energy consumption. Next, taking into consideration the time complexity of the optimization problems, a heuristic partitioning method based on Genetic Algorithm (GABP) is proposed to solve each of the three objectives and with the capability of acceptable performance maintenance in both dynamic and static partitionings. In order to evaluate and analyze the performance of the proposed approach, a simulation framework was built to run for random graphs of different sizes with the capability of setting specific bandwidth limits as target. The simulation results evidence improved performance against bandwidth fluctuations when compared to similar approaches. Moreover, it was also seen that once the problem circumstances are modified, the offloading can take place in the vicinity of the target node. Furthermore, we implemented the proposed method in form of an application on Android platform to conduct experiments on real applications. The experiments prove that those partitions of the applications requiring higher processing reqources rather than data rate are the best candidates for offloading.
Keywords: Computation offloading, partitioning, bandwidth adaptation, web service, data rate limitation -
Pages 23-36
In linguistics, a tree bank is a parsed text corpus that annotates syntactic or semantic sentence structure. The exploitation of tree bank data has been important ever since the first large-scale tree bank, The Penn Treebank, was published. However, although originating in computational linguistics, the value of tree bank is becoming more widely appreciated in linguistics research as a whole. For example, annotated tree bank data has been crucial in syntactic research to test linguistic theories of sentence structure against large quantities of naturally occurring examples. The natural language parser consists of two basic parts, POS tagger and the syntax parser. A Part-Of-Speech Tagger (POS Tagger) is a piece of software that reads text in some languages and assigns parts of speech to each word (and other token), such as noun, verb, adjective, etc., although generally computational applications use more fine-grained POS tags like 'noun-plural'. A natural language parser is a program that works out the grammatical structure of sentences, for instance, which groups of words go together (as "phrases") and which words are the subject or object of a verb. Probabilistic parsers use knowledge of language gained from hand-parsed sentences to try to produce the most likely analysis of new sentences. These statistical parsers still make some mistakes, but commonly work rather well. Inaccurate design of context-free grammars and using bad structures such as Chomsky normal form can reduce accuracy of probabilistic context-free grammar parser. Weak independence assumption is one of the problems related to CFG. We have tried to improve this problem with parent and child annotation, which copies the label of a parent node onto the labels of its children, and it can improve the performance of a PCFG. In grammar, a conjunction (conj) is a part of speech that connects words, phrases, or clauses that are called the conjuncts of the conjunctions. In this study, we examined the conjunction phrases in the Persian tree bank. The results of this study show that adding structural dependencies to grammars and modifying the basic rules can remove conjunction ambiguity and increase accuracy of probabilistic context-free grammar parser. When a part-of-speech (PoS) tagger assigns word class labels to tokens, it has to select from a set of possible labels whose size usually ranges from fifty to several hundred labels depending on the language. In this study, we have investigated the effect of fine and coarse grain POS tags and merging non-terminals on Persian PCFG parser.
Keywords: Probabilistic context free grammar, parser, tree bank, conjunction phrases, parent annotation, child annotation, part of speech tags -
Pages 37-48
The development of computer systems and extensive use of information technology in the everyday life of people have just made it more and more important for them to make quick access to information that has received great importance. Increasing the volume of information makes it difficult to manage or control. Thus, some instruments need to be provided to use this information. The QA system is an automated system for obtaining the correct answers to questions posed by the human in the natural language. In these systems, if the response is found, and if it is not the user's expected response or if it needs more information, there is no possibility of exchanging information between the system and the user to ask more questions and get answers related to it. To solve this problem, interactive Question answering (IQA) systems were created. Interactive question answering (IQA) systems are associated with linguistic ambiguous structures, so these systems are more accurate than QA systems. Regarding the probability of ambiguity (ambiguity in the user question or ambiguity in the answer provided by the system), the repetition is possible in these systems to obtain the clarity. No standard methods have been developed on IQA systems evaluation, and the existing evaluation methods have been developed based on the methods used in QA and dialogue systems. In evaluating IQA systems, in addition to quantitative evaluation, a qualitative evaluation is used. It requires users’ participation in the evaluation process to determine the success level of interaction between the system and the user. Evaluation plays an important role in the IQA systems. In the context of evaluating IQA systems, there is partially no specific methodology for evaluating these systems in general. The main problem with designing an assessment method for IQA systems lies in the rare possibility to predict the interaction part. To this end, human needs to be involved in the evaluation process. In this paper, an appropriate model is presented by introducing a set of built-in features for evaluating IQA systems. To conduct the evaluation process, four IQA systems were considered based on the conversation exchanged between users and systems. Moreover, 540 samples were considered as suitable data to create a test and training set. The statistical characteristics of each conversation were extracted after performing the preprocessing on them. Then a feature matrix was formed based on the obtained characteristics. Finally, using linear and nonlinear regression, human thinking was predicted. As a result, the nonlinear power regression with 0.13 Root Mean Square Error (RMSE) was the best model.
Keywords: Evaluation, Interactive Question Answering Systems, Nonlinear Regression, Feature Extraction -
Pages 49-60
A treebank is a corpus with linguistic annotations above the level of the parts of speech. During the first half of the present decade, three treebanks have been developed for Persian either originally or subsequently based on dependency grammar: Persian Treebank (PerTreeBank), Persian Syntactic Dependency Treebank, and Uppsala Persian Dependency Treebank (UPDT). The syntactic analysis of a sentence in these corpora involves a series of relations introducing each word in the sentence as a dependent of another, referred to as the head. Examination of head-dependent pairs extracted from similar contexts in the above treebanks reveals frequent, apparently systematic inconsistencies observed particularly in the cases of nominal and adjectival heads. This can be explained in terms of the failure to postulate valency structures for nouns and adjectives as well as for verbs, taking for granted that tokens receive the proper labels regardless of such an assumption. When the notion of valency was borrowed from chemistry to refer to the number of controlled arguments, it was meant to apply only to verbs. Later developments of dependency grammar included the proposal of nominal and adjectival valency as well. The significance of the idea seems to have been underestimated, though. It has been highly improbable, therefore, for developers of dependency treebanks to design their annotation schemes otherwise. As far as Persian is concerned, Uppsala Persian Dependency Treebank and Dependency Persian Treebank (DepPerTreeBank, the dependency version of PerTreeBank) have used the Stanford Typed Dependencies. The later version of the former treebank, Persian Universal Dependency Treebank, has used the Universal Dependencies. These are standard annotation schemes that do not recognize valency for nouns and adjectives. Furthermore, Persian Syntactic Dependency Treebank has used its own set of dependency relations, where little attention has been paid to the idea. This paper reported the design process of a scheme for annotation of Persian dependency structure as part of an ongoing project of developing a dependency treebank for Persian. The scheme was based on a comprehensive description of Persian syntax according to a theory introduced as the Autonomous Phrases Theory. The main idea is that the significance of phrases should be appreciated in dependency analyses due to their cognitive reality, and the notion of valency is also extended beyond verbs, on which basis every dependent of whatever head type is classified as either a complement or an adjunct. Moreover, to make the resulting annotation scheme reasonably intelligible to the target audience, the latest standard available annotation scheme, Universal Dependencies (UD), was adapted to suit the requirements of the adopted framework. The outcome was a tag set of fifty-three dependency relations, including fifteen original labels and the rest borrowed from the universal dependencies. Although it provides more detailed annotation than UD does by making finer distinctions, our scheme does not involve too many tags more than UD does, mainly because a large number of the additional relations are shared by two or three head types.
Keywords: annotation, Persian, treebank, Universal Dependencies, valency -
Pages 61-78
Nowadays automated early warning systems are essential in human life. One of these systems is fire detection which plays an important role in surveillance and security systems because the fire can spread quickly and cause great damage to an area. Traditional fire detection methods usually are based on smoke and temperature detectors (sensors). These methods cannot work properly in large space and out-door environments. They have high false alarm rates, and to cover the entire area, many smoke or temperature fire detectors are required, that is expensive. Due to the rapid developments in CCTV (Closed Circuit Television) surveillance system in recent years and video processing techniques, there is a big trend to replace conventional fire detection techniques with computer vision-based systems. This new technology can provide more reliable information and can be more cost-effective. The main objective of fire detection systems is high detection accuracy, low error rate and reasonable time detect. The video fire detection technology uses CCD cameras to capture images of the observed scene, which provides abundant and intuitive information for fire detection using image processing algorithms.
This paper presents an efficient fire detection system which detects fire areas by analyzing the videos that are acquired by surveillance cameras in urban out-door environment, especially in storage of Yazd Gas Company. Proposed method uses color, spatial and temporal information that makes a good distinction between the fire and objects which are similar to the fire. The purpose is achieved using multi- filter. The first filter separates red color area as a primary fire candidate. The second filter operates based on the difference between fire candidate areas in the sequence of frames. In the last filter, variation of red channel in the candidate area is computed and compared with the threshold which is updating continuously. In the experiments, the performance of these filters are evaluated separately. The proposed final system is a combination of all filters. Experimental results show that the precision of the final proposed system in urban out-door environment is 100%, and our technique achieves the average detection rate of 87.35% which outperforms other base methods.Keywords: Fire Detection, Video Sequences, Image Processing, Detection Time -
Pages 79-88
Nowadays, users can share their ideas and opinions with widespread access to the Internet and especially social networks. On the other hand, the analysis of people's feelings and ideas can play a significant role in the decision making of organizations and producers. Hence, sentiment analysis or opinion mining is an important field in natural language processing. One of the most common ways to solve such problems is machine learning methods, which creates a model for mapping features to the desired output. One challenge of using machine learning methods in NLP fields is feature selection and extraction among a large number of early features to achieve models with high accuracy. In fact, the high number of features not only cause computational and temporal problems but also have undesirable effects on model accuracy. Studies show that different methods have been used for feature extraction or selection. Some of these methods are based on selecting important features from feature sets such as Principal Component Analysis (PCA) based methods. Some other methods map original features to new ones with less dimensions but with the same semantic relations like neural networks. For example, sparse feature vectors can be converted to dense embedding vectors using neural network-based methods. Some others use feature set clustering methods and extract less dimension features set like NMF based methods. In this paper, we compare the performance of three methods from these different classes in different dataset sizes. In this study, we use two compression methods using Singular Value Decomposition (SVD) that is based on selecting more important attributes and non-Negative Matrix Factorization (NMF) that is based on clustering early features and one Auto-Encoder based method which convert early features to new feature set with the same semantic relations. We compare these methods performance in extracting more effective and fewer features on sentiment analysis task in the Persian dataset. Also, the impact of the compression level and dataset size on the accuracy of the model has been evaluated. Studies show that compression not only reduces computational and time costs but can also increase the accuracy of the model. For experimental analysis, we use the Sentipers dataset that contains more than 19000 samples of user opinions about digital products and sample representation is done with bag-of-words vectors. The size of bag-of-words vectors or feature vectors is very large because it is the same as vocabulary size. We set up our experiment with 4 sub-datasets with different sizes and show the effect of different compression performance on various compression levels (feature count) based on the size of dataset size. According to experiment results of classification with SVM, feature compression using the neural network from 7700 to 2000 features not only increases the speed of processing and reduces storage costs but also increases the accuracy of the model from 77.05% to 77.85% in the largest dataset contains about 19000 samples. Also in the small dataset, the SVD approach can generate better results and by 2000 features from 7700 original features can obtain 63.92 % accuracy compared to 63.57 % early accuracy. Furthermore, the results indicate that compression based on neural network in large dataset with low dimension feature sets is much better than other approaches, so that with only 100 features extracted by neural network-based auto-encoder, the system achieves acceptable 74.46% accuracy against SVD accuracy 67.15% and NMF accuracy 64.09% and the base model accuracy 77.05% with 7700 features.
Keywords: Natural Language Processing, Sentiment Analysis, Opinion Mining, Auto-Encoder, Singular Value Decomposition, Nonnegative Matrix Factorization -
Pages 89-100
Tracking filters are extensively used within object tracking systems in order to provide consecutive smooth estimations of position and velocity of the object with minimum error. Namely, Kalman filter and its numerous variants are widely known as simple yet effective linear tracking filters in many diverse applications. In this paper, an effective method is proposed for designing and implementation of a Kalman filter in an object tracking application. The considered tracking application implies the capability to produce a smooth and reliable output stream by the tracking filter, even in presence of different disturbing types of noise, including background or spontaneous noises, as well as disturbances with continues or discrete nature. The presented method includes a fixed-point implementation of the Kalman filter on FPGA, which targets the joint estimation of position-velocity pair of an intended object in heavy presence of noise. The execution speed of the Kalman algorithm is drastically enhanced in the proposed implementation. This enhancement is attained by emphasis on hardware implementation of every single computational block on the one hand, and through appropriate parallelization and pipelining of independent tasks within the Kalman process on the other hand. After designing the filter parameters with respect to the requirements of a given tracking problem, a floating-point model and a fixed-point hardware model of the filter are implemented using MATLAB and Xilinx System Generator, respectively. In order to evaluate the performance of the filter under realistic circumstances, a set of appropriately defined scenarios are carried out. The simulations are carefully designed in order to represent the extremely harsh scenarios in which the input measurements to the filter are deeply polluted by different kinds of noises. In each simulation the position-velocity data corresponding to a moving object is generated according to an appropriate model, quantized, and contaminated by noise and fed into the filter. Performances of the Kalman filter in software version (i.e. the floating point replica) and hardware version (i.e. the fixed-point replica) are quantitatively compared in the designed scenario. Our comparison employs NMSE and maximum error values as quantitative measures, verifying the competency of our proposed fixed-point hardware implementation. The results of our work show that, with adequate selection word length, the implemented filter is fast and efficient; it confines the algorithm execution time to 50 clock pulses, i.e. about 0.4 µs when a 125 MHz clock is used. It is also verified that our implementation reduces the position and velocity estimation errors by 11 dB and 1.2 dB, respectively. The implemented filter also confines the absolute values of maximum error in position and velocity to 10 meter and 0.7 meter/sec. in the considered scenario, which is almost resembles the performance of its floating point counterpart. The presented Kalman filter is finally implemented on Zc706 evaluation board and the amount of utilized hardware resource (FFs, LUTs, DSP48, etc.) are reported as well as the estimated power consumption of the implemented design. The paper is concluded through comparison of the proposed design with some recent works which confirms the efficacy of the presented implementation.
Keywords: Kalman filter, FPGA implementation, Tracking, Distance estimation, Velocity estimation -
Pages 101-116
In the field of the words recognition, three approaches of words isolation, the overall shape and combination of them are used. Most optical recognition methods recognize the word based on break the word into its letters and then recogniz them. This approach is faced some problems because of the letters isolation dificulties and its recognition accurcy in texts with a low image quality. Therefore, an approach based on none separating recognition could be useful in such cases. In methods based on the overall shapes for subword recognition after extraction of subword features usually these features are searched in the image dictionary created in the training phase. Therefore, by considering that we are faced with massive amounts of classes, proposing ways to limit the scope of the search are the main challenges in the overall shape methods. Thus, the information of the overall shape usually is used to reduce the scope search in a hierarchical form. In this paper, it is tried to reduce the search space of the subwords severely by using a simple and efficient method. In training phase, training data is grouped based on the location of the points and signs, in the groups where have more than 10 subwords, to reduce the search space, according to the number of elements in the group, by extracting the simple features of horizontal and vertical profiles clustering takes place. In recognition phase, in the first step, by determining the width to height ratio of the subword (with signs and without signs) and the position code of the points and signs, the search scope is limited to subwords with this position code that are within the range of the ratios mentioned. This range would be accepted if the number of subwords in this phase is less than ten. Otherwise, in the next step, by extracting the simple features of the horizontal and vertical profiles of the subwords, the search space will be limited to a number of the closest clusters to this subword that also satisfies the width-to-height ratio. By using the proposed method of this paper, the search space has fallen to an acceptable level. In this study, a database of 12700 subwords with five Lotus, Zar, Nazanin, Mitra and Yaghut fonts scanned 400 dpi was used. The four Lotus, Zar, Nazanin and Mitra fonts were used in the training phase and in the test phase, Yaghut font is used.
Keywords: Recognition, Farsi Typed Subwords, Search Space Reduction, Position of the Points, Symbols -
Pages 117-128
Persian Language has a special feature (grapheme, homophone, and multi-shape clinging characters) in electronic devices. Furthermore, design and implementation of NLP tools for Persian are more challenging than other languages (e.g. English or German). Spelling tools are used widely for editing user texts like emails and text in editors. Also developing Persian tools will provide Persian programs to check spell and reduce errors in electronic texts. In this work, we review the spelling detection and correction methods, especially for the Persian language. The proposed algorithm consists of two steps. The first step is non-word error detection and correction by intelligent scoring algorithm. The second step is read-word error detection and correction. We propose a spelling system "Perspell” for Persian non-word and real-word errors using a hybrid scoring system and optimized language model by lexicon. This scoring system uses a combination of lexical and semantic features optimized by learning dataset. The weight of these features in scoring system is also optimized by learning phase. Perspell is compared with known Persian spellchecker systems and could overcome them in precision of detection and correction. Accordingly, the proposed Persian spell-checker system can also detect and correct real-word errors. This open challenge category of spelling is a complicated and time consuming task in Persian as well as, assessing the proposed method, the F-measure metric has improved significantly (about 10%) for detecting and correcting Persian words. In the proposed method, we used Persian language model with bootstrapping and smoothing to overcome data sparseness and lack of data. The bootstrapping is developed using a Persian dictionary and further we used word sense disambiguation to select the correct related replaced word.
Keywords: Spell Error Detection, Spell Error Correction, Persian spell Checker, NLP, Persian Language Model -
Pages 129-148
Image processing is a method to perform some operations on an image, in order to get an enhanced image or to extract some useful information from it. The conventional image processing algorithms cannot perform well in scenarios where the training images (source domain) that are used to learn the model have a different distribution with test images (target domain). Also, many real world applications suffer from a limited number of training labeled data and therefore benefit from the related available labeled datasets to train the model. In this way, since there is the distribution difference across the source and target domains (domain shift problem), the learned classifier on the training set might perform poorly on the test set. Transfer learning and domain adaptation are two outstanding solutions to tackle this challenge by employing available datasets, even with significant difference in distribution and properties, to transfer the knowledge from a related domain to the target domain. The main assumption in domain shift problem is that the marginal or the conditional distribution of the source and the target data is different. Distribution adaptation explicitly minimizes predefined distance measures to reduce the difference in the marginal distribution, conditional distribution, or both. In this paper, we address a challenging scenario in which the source and target domains are different in marginal distributions, and the target images have no labeled data. Most prior works have explored two following learning strategies independently for adapting domains: feature matching and instance reweighting. In the instance reweighting approach, samples in the source data are weighted individually so that the distribution of the weighted source data is aligned to that of the target data. Then, a classifier is trained on the weighted source data. This approach can effectively eliminate unrelated source samples to the target data, but it would reduce the number of samples in adapted source data, which results in an increase in generalization errors of the trained classifier. Conversely, the feature-transform approach creates a feature map such that distributions of both datasets are aligned while both datasets are well distributed in the transformed feature space. In this paper, we show that both strategies are important and inevitable when the domain difference is substantially large. Our proposed using sample-oriented Domain Adaptation for Image Classification (DAIC) aims to reduce the domain difference by jointly matching the features and reweighting the instances across images in a principled dimensionality reduction procedure, and construct new feature representation that is invariant to both the distribution difference and the irrelevant instances. We extend the nonlinear Bregman divergence to measure the difference in marginal, and integrate it with Fisher’s linear discriminant analysis (FLDA) to construct feature representation that is effective and robust for substantial distribution difference. DAIC benefits pseudo labels of target data in an iterative manner to converge the model. We consider three types of cross-domain image classification data, which are widely used to evaluate the visual domain adaptation algorithms: object (Office+Caltech- 256), face (PIE) and digit (USPS, MNIST). We use all three datasets prepared by and construct 34 cross-domain problems. The Office-Caltech-256 dataset is a benchmark dataset for cross-domain object recognition tasks, which contains 10 overlapping categories from following four domains: Amazon (A), Webcam (W), DSLR (D) and Caltech256 (C). Therefore 4 × 3 = 12 cross domain adaptation tasks are constructed, namely A → W, ..., C → D. USPS (U) and MNIST (M) datasets are widely used in computer vision and pattern recognition tasks. We conduct two handwriting recognition tasks, i.e., usps-mnist and mnist-usps. PIE is a benchmark dataset for face detection task and has 41,368 face images of size 3232 from 68 individuals. The images were taken by 13 synchronized cameras and 21 flashes, under varying poses, illuminations, and expressions. PIE dataset consists five subsets depending on the different poses as follows: PIE1 (C05, left pose), PIE2 (C07, upward pose), PIE3 (C09, downward pose), PIE4 (C27, frontal pose), PIE5 (C29, right pose). Thus, we can construct 20 cross domain problems, i.e., P1 → P2, P1 → P3, ..., P5 → P4. We compare our proposed DAIC with two baseline machine learning methods, i.e., NN, Fisher linear discriminant analysis (FLDA) and nine state-of-the-art domain adaptation methods for image classification problems (TSL, DAM, TJM, FIDOS and LRSR). Due to these methods are considered as dimensionality reduction approaches, we train a classifier on the labeled training data (e.g., NN classifier), and then apply it on test data to predict the labels of the unlabeled target data. DAIC efficiently preserves and utilizes the specific information among the samples from different domains. The obtained results indicate that DAIC outperforms several state of-the-art adaptation methods even if the distribution difference is substantially large.
Keywords: Image processing, Transfer learning, Bregman divergence, Marginal distribution difference reduction, Dimensionality reduction