فهرست مطالب
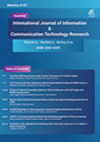
International Journal Information and Communication Technology Research
Volume:11 Issue: 3, Summer 2019
- تاریخ انتشار: 1399/08/19
- تعداد عناوین: 7
-
-
Pages 1-8
We study the problem of physical layer security in massive multiple-input multiple-output (MaMIMO) cognitive radio networks (CRN). In particular, we investigate the design of a smart jamming attack on the uplink transmission of a CRN in the presence of a single antenna jammer. The jammer is aware of the transmission protocol as well as the pilot set used for channel training in the MaMIMO systems. It attacks both the training and data transmission phases but with different powers. To have the most destructive attack, the jammer optimally divides its power between two phases to minimize the maximum energy efficiency of the secondary system. The resulting power optimization is a non-convex problem and to solve it, we propose a method to transform it into a convex optimization problem. Numerical results illustrate the effectiveness of the proposed smart jamming attack in decreasing the energy efficiency of the secondary MaMIMO system.
Keywords: Physical layer security, massive MIMO, cognitive radio network, jamming, energy efficiency, convex optimization, power allocation -
Pages 9-18
Due to the increasing use of wireless communications, infrastructure-less networks should be highly considered. Delay Tolerant Network (DTN) as such networks does not have the end to end path between the source and destination nodes. Consequently, routing in DTN as an open issue needs to be studied. Many replication protocols such as epidemic routing are proposed in these challenging wireless environments. The main idea of epidemic routing is to send a copied message to each node without that message. The replication process consumes a high amount of network resources such as energy. In this work, a probabilistic epidemic (p-epidemic) forwarding scheme is suggested that minimizes the energy consumption and maximizes the message delivery probability within the heterogeneous sets of nodes using Non-dominated Sorting Genetic Algorithm II (NSGA-II). Current research considers all the nodes of the network with the equal transmission radii and the network is assumed homogeneous. In our work, the nodes have two different amounts of available energies and two different transmission radii. The radius of each node is chosen according to the current energy of that node. The node energy has a significant role in successfully delivering the messages. Regarding the node energy, the lower transmission radius the node has, the lower its chance to send the message with the lower probability. The node with the higher transmission radius sends the message with a higher probability. The optimal transmission probabilities are obtained by NSGA-II. The front of optimal solutions according to these probabilities for delivery probability and energy consumption are observed.
Keywords: Delay Tolerant Networks, Node Current Energy, NSGA-II, Probabilistic Epidemic Forwarding, Transmission Probability -
Pages 17-30
Wireless sensor network (WSN) is one of the most important components of the Internet of Things (IoT). IoT on the WSN layer, measures different parameters by different sensors embedded in the multi-sensor nodes. The limitation of energy sources in the sensor nodes is one of the most important challenges in exploiting WSNs. Routing and data aggregation are two basic methods to reduce the energy consumption in the WSNs. Compressive sensing (CS) is one of the most effective techniques for data aggregation in WSNs. The most studies related to the use of CS techniques to reduce communication cost in the network are based on the single-sensor node WSNs. So, in this paper, we show that how CS techniques can be applied to the multi-sensor IoT-based WSNs. Given that the sparsity of the environmental data read by multi-sensor nodes is an important parameter for using the CS techniques in WSNs, various different scenarios have been analyzed from the point of view of data sparsity in this study, as well as transmission methods, and data aggregation techniques in a multi-sensor WSN. To evaluate the performance of the CS techniques in a multi-sensor WSN, all investigated scenarios are evaluated for two important techniques of CS named compressive data gathering (CDG) and hybrid compressive sensing (HCS), and in order to show the efficiency of the system in using of the CS, these techniques have been compared to the conventional Non-CS method. We show that the use of HCS technique has a considerable effect on reducing communication costs in a multi-sensor IoT-based WSN.
Keywords: compressive sensing, hybrid compressive sensing, internet of things, wireless sensor network, multi-sensor -
Pages 31-41
An input selection strategy is an important part of a router that is done by an arbitration process. When an output channel is requested by two or more input channels simultaneously, the best input channel will be selected by the input selection strategy. This research presents a new input selection strategy called DTIS (Destination Traffic based Input Selection). The DTIS uses local and non-local congestion information on the path to distribute traffic more evenly over the network. Also, a global congestion aware method called DCA is used to give priority to an input channel according to the destination. The simulation results prove that DTIS improves the average latency and throughput for various synthetic and real traffic patterns with acceptable overhead in terms of area consumption. The simulation results show the average delay improvements of DTIS to the CAIS and Round Robin strategies are 26% and 77%, respectively.
Keywords: Keywords-component, Network on Chip, Arbitration, Input selection strategy, Destination traffic, Performance evaluation -
Pages 42-48
In recent years, data mining has played an essential role in computer system performance, helping to improve system functionality. One of the most critical and influential data mining algorithms is anomaly detection. Anomaly detection is a process in detecting system abnormality that helps with finding system problems and troubleshooting. Intrusion and fraud detection services used by credit card companies are some examples of anomaly detection in the real world. According to the increasing volumes of the datasets that creates big data, traditional data mining approaches do not have efficient enough results. Various platforms, frameworks, and algorithms for big data mining have been presented to account for this deficiency. For instance, Hadoop and Spark are some of the most used frameworks in this field. Support Vector Machine (SVM) is one of the most popular approaches in anomaly detection, which—according to its distributed and parallel extensions—is widely used in big data mining. In this research, Mutual Information is used for feature selection. Besides, the kernel function of the one-class support vector machine has been improved; thus, the performance of the anomaly detection improved. This approach is implemented using Spark. The NSL-KDD dataset is used, and an accuracy of more than 80 percent is achieved. Compared to the other similar approaches in anomaly detection, the results are improved.
Keywords: Anomaly detection, support vector machine, big data, improvement of anomaly detection, one-class support vector machine, Mutual Information -
Pages 49-56
Web Application Firewall (WAF) is known as one of the Intrusion Detection System (IDS) solutions for protecting web servers from HTTP attacks. WAF is a tool to identify and prevent many types of attacks, such as XSS and SQL-injection. In this paper, deep machine learning algorithms are used for enriching the WAF based on the anomaly detection method. Firstly, we construct attributes from HTTP data, to do so we consider two models namely n-gram and one-hot. Then, according to Auto-Encoder LSTM (AE-LSTM) as an unsupervised deep leaning method, we should extract informative features and then reduce them. Finally, we use ensemble isolation forest to train only normal data for the classifier. We apply the proposed model on CSIC 2010 and ECML/ PKDD 2007 datasets. The results show AE-LSTM has higher performance in terms of accuracy and generalization compared with naïve methods on CSIC dataset; the proposed method also have acceptable detection rate on ECML/PKDD dataset using n -gram model.
Keywords: Web Application Firewall, Anomaly Detection, LSTM, AE-LSTM, Ensemble Isolation Forest -
Pages 57-67
Sentiment analysis is considered as one of the most essential tasks in the field of natural language processing and cognitive science. In order to enhance the performance of sentiment analysis techniques, it is necessary to not only classify the sentences based on their sentimental labels but also to extract the informative words that contribute to the classification decision. In this regard, deep neural networks based on the attention mechanism have achieved considerable progress in recent years. However, there is still a limited number of studies on attention mechanisms for text classification and especially sentiment analysis. To fill this lacuna, a Convolution Neural Network (CNN) integrated with attention layer is presented in this paper that is able to extract informative words and assign them higher weights based on the context. In the attention layer, the proposed model employs a context vector and tries to measure the importance of a word as the similarity between the context vector and word vector. Then, by integrating the new vectors obtained from the attention layer into sentence vectors, the new generated vectors are used for classification. In order to verify the performance of the proposed model, various experiments were conducted on the Stanford datasets. Based on the results of the experiments, the proposed model not only significantly outperforms other existing studies but also is able to consider the context to extract the informative words which can be considered as a value in analysis and application.
Keywords: Natural language processing, Sentiment analysis, Deep Learning, Convolutional neural network, Attention mechanism