فهرست مطالب
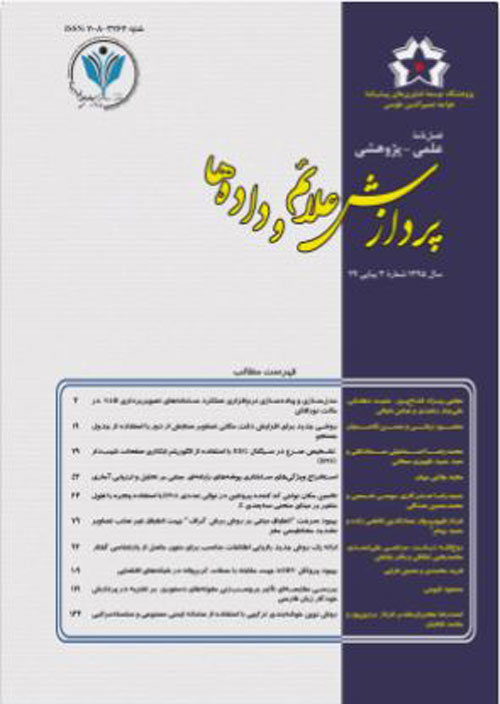
فصلنامه پردازش علائم و داده ها
سال هجدهم شماره 3 (پیاپی 49، پاییز 1400)
- تاریخ انتشار: 1400/10/27
- تعداد عناوین: 10
-
-
صفحات 3-18
امروزه مبدل های دیجیتال از مهم ترین ادوات یک سامانه پردازش سیگنال و داده به شمار رفته و به صورت گسترده در زمینه پردازش صوت، تصویر و سیگنال های حیاتی به کار گرفته می شوند. در طراحی مبدل های دیجیتال VLSI، سنتز سطح بالا (HLS) یکی از مراحل مهم و تاثیرگذار به شمار می رود. هدف اصلی از انجام این کار، کمینه کردن واحدهای پایه دیجیتالی مورد استفاده در پروژه مفروض جهت بهبود توان، تاخیر، و سطح مصرفی آن ها است. این کار عمدتا با تحلیل گراف مسیر داده (DFG) اتفاق می افتد. بهبود در این مرحله، علاوه بر بازدهی بیشتر باعث کاهش زمان طراحی در مراحل پایین تر می شود. ماهیت پیچیده، گسترده و گسسته مسایل سنتز سطح بالا، باعث شده است که آنها در زمره مسایل بسیار دشوار در مهندسی مدارات VLSI به شمار آیند؛ از این رو استفاده از روش های فراابتکاری و هوش جمعی جهت حل پروژه های مرتبط با سنتز سطح بالا، گزینه ای مطلوب به نظر می رسد. در این مقاله روشی مبتنی بر الگوریتم فراابتکاری "شعله و پروانه"(MFO) جهت یافتن بهترین طرح سخت افزاری برای انواع مبدل های دیجیتال ارایه شده است. نتایج مقایسه ای در کنار نتایج حاصل از روش مبتنی بر الگوریتم ژنتیک (GA) نشان داد که روش پیشنهادی از توانایی بالاتری در ارایه ساختار سخت افزاری مناسب و سنتز سطح بالای انواع مبدل ها برخوردار است. همچنین ویژگی دیگر روش پیشنهادی، سرعت بالای آن در یافتن پاسخ بهینه است (میانگین برتری بیش از 20% نسبت به GA).
کلیدواژگان: سنتز سطح بالا، مسیرداده، مبدل های دیجیتال، الگوریتم های فراابتکاری، الگوریتم بهینه سازی شعله و پروانه -
صفحات 19-28
الگوریتم کمینه میانگین ترکیب نرم (LMMN)، الگوریتمی مبتنی بر شیب تصادفی خطا است که هدف آن کمینه سازی ترکیبی از توابع هزینه الگوریتم های کمینه میانگین مربعات (LMS) و کمینه میانگین چهارم (LMF) است. این الگوریتم بسیاری از ویژگی ها و مزایای الگوریتم های LMS و LMF را با خود به ارث برده است و از جهاتی ضعف های این دو الگوریتم را هم برطرف کرده است. بزرگ ترین مشکل الگوریتم LMMN فقدان یک مدل تحلیلی برای پیش بینی رفتار آن است، به طوری که کاربرد عملی آن را محدود کرده است. ما در این مقاله باهدف حل این مشکل، مدلی تحلیلی را ارایه می کنیم که قادر است رفتار میانگین مربعات خطا و میانگین خطای وزن ها را با دقت بالایی پیش بینی کند. دقت مدل استخراج شده از طریق آزمایش های متعددی تایید می شود.
کلیدواژگان: الگوریتم های وفقی، الگوریتم LMMN، مدل تحلیلی -
صفحات 29-44
امروزه باج افزارهای رمز کننده تبدیل به یکی از مهم ترین تهدیدات حوزه سایبری شده است. یک باج افزار رمزکننده با رمز کردن داده های با ارزش قربانی، دسترسی به داده ها را از بین می برد و در ازای رمزگشایی آن ها درخواست پرداخت باج می کند. به علت نوظهور بودن باج افزارهای رمزکننده، پژوهش چندانی در جهت شناسایی آن ها انجام نشده است و بیش تر پژوهش های مرتبط روی سیستم فایل و نظارت بر رفتار فرآیندها روی فایل ها انجام شده است. از آن جایی که سرعت در تشخیص باج افزارها اهمیت فراوانی دارد، تمرکز این مقاله روی تشخیص دقیق و زودهنگام باج افزارها بر اساس تحلیل لاگ های رفتاری است. در این مقاله، ابتدا محیط آزمایشی مناسبی را ایجاد می کنیم تا بتوانیم رفتار 572 نمونه باج افزار از خانواده TeslaCrypt، 535 نمونه باج افزار از خانواده Cerber و 517 نمونه باج افزار از خانواده Locky را ثبت کنیم که محیط مهیا شده قابلیت کاربرد در سایر پروژه ها و پژوهش های مشابه را دارد. برای دسته بندی و شناسایی نمونه های باج افزار، با بهره گیری از روش کاوش الگوهای متوالی، ویژگی هایی را به دست می آوریم تا قابل استفاده برای الگوریتم های دسته بندی کننده یادگیری ماشین باشد. دقت 99% در تشخیص نمونه های باج افزار و همین طور دقت 96.5% در شناسایی و دسته بندی خانواده آن ها روی الگوریتم های متداول یادگیری ماشین نشان از کیفیت بالای ویژگی های پیشنهادی دارد.
کلیدواژگان: بدافزار، باج افزار، باج افزار رمز کننده، شناسایی باج افزار، شناسایی خانواده باج افزار -
صفحات 45-64
توییتر یکی از محبوب ترین و مشهورترین شبکه های اجتماعی برخط برای گسترش اطلاعات است که در عین قابل اعتماد بودن، می تواند به عنوان منبعی برای گسترش شایعات باشد. شایعاتی غیرواقعی و فریبنده که می تواند تاثیرات جبران ناپذیری برروی افراد و جامعه به وجود بیاورد. در این پژوهش مجموعه کاملی از ویژگی های جدید ساختاری مربوط به درخت پاسخ و گراف کاربران در تشخیص مکالمه های شایعه توییتر استخراج شدند. این ویژگی ها با توجه به معیارهای سنتی گراف ها و معیارهای مخصوص انتشار شایعه، در بازه های زمانی مختلف به مدت 24 ساعت از زمان شروع مکالمه ها در خصوص رویدادهای بحرانی در توییتر استخراج شده اند. نتایج حاصل از بررسی ویژگی های جدید، دیدگاه عمیقی از ساختار انتشار اطلاعات در مکالمه ها را فراهم می کند. بر اساس نتایج به دست آمده، ویژگی های جدید ساختاری در تشخیص مکالمه های شایعه در رویدادهای توییتر موثر هستند؛ ازاین رو، الگوریتم دسته بند شایعه مبتنی بر ویژگی های جدید ساختاری، زبانی و کاربران در تشخیص مکالمه های شایعه زبان انگلیسی توییتر ، پیشنهاد داده شد. روش پیشنهادی در مقایسه با روش های پایه، عملکرد بهتری دارد. همچنین، با توجه به اهمیت کاربر توییت منبع در مکالمه ها، این کاربر از جنبه های مختلفی موردبررسی و آنالیز قرار گرفت.
کلیدواژگان: مکالمه، تشخیص شایعه، توییتر، درخت پاسخ، گراف کاربران -
صفحات 65-76
مساله دریافت فشرده در همین اواخر توجه زیادی در پردازش سیگنال به خود جلب کرده به طوری که بخش اعظمی از پژوهش ها در این حوزه به این مساله معطوف شده است. از جمله حوزه کاربردی دریافت فشرده، کاربرد آن در شبکه های حس گری بی سیم است. ساختمان این شبکه ها که متشکل از حس گرهای بی سیم با توان محدود است، ایجاب می کند تا الگوریتم هایی که برای این کاربرد ارتقا داده می شوند، به لحاظ مصرف انرژی بهینه باشند. به عبارتی، الگوریتم های طراحی شده برای این زمینه می بایست پیچیدگی های محاسباتی کمتری داشته و نیازمند کمترین تبادلات بین حس گرها باشند. بر همین اساس، در این مقاله الگوریتم بازسازی دریافت فشرده توزیع شده ای برحسب مد مشارکتی افزایشی دوجهته پیشنهاد شده است؛ در حقیقت، نخست یک چهارچوب جامع توزیع شده برای بازسازی سیگنال های تنک در شبکه های حس گری ارایه شده و سپس این چهارچوب برای مسایل بهینه سازی متفاوتی پیاده شده است. پیچیدگی پایین محاسباتی و عملکرد حالت دایم بهتر مهم ترین مشخصه الگوریتم های پیشنهادی است.
کلیدواژگان: شبکه های حس گری بی سیم، سیگنال تنک، توپولوژی افزایشی دوجهته، دریافت فشرده، الگوریتم بازسازی -
صفحات 77-90
یک سامانه تشخیص پلاک خودرو شامل سه بخش: شناسایی پلاک (های) تصویر، استخراج کاراکترها و تشخیص نویسه ها است. اولین و مهم ترین مرحله در یک سامانه تشخیص پلاک، شناسایی ناحیه پلاک در تصویر است. در این مقاله یک روش کارا و مبتنی بر طبقه بندی کننده سلسله مراتبی برای شناسایی پلاک (های) خودروهای ایرانی در تصویر پیشنهاد شده و رویکردهایی جهت بهبود کارایی سامانه پیشنهاد شده است. در ابتدا با بازخورد گرفتن از نمونه های منفی (نواحی فاقد پلاک)، یک رویکرد آموزشی دو مرحله ای و سپس برای کاهش تعداد هشدار غلط و افزایش دقت شناسایی ناحیه، یک رویکرد آزمایش دو مرحله ای ارایه شده است. در نهایت روشی ترکیبی با ادغام دو رویکرد آموزش و آزمایش دو مرحله ای معرفی شده است. این سامانه بر روی تصاویر رنگی و خاکستری قابل اعمال است و توانایی شناسایی چند پلاک در تصویر، از نمای جلو و عقب خودرو، در شرایط نوری مختلف، در محیط های شلوغ و پلاک ها با مقیاس های مختلف را دارد و به محل نصب پلاک و یا ناحیه خاصی از پلاک و همچنین رنگ پس زمینه پلاک حساس نیست. برای ارزیابی رویکردهای ارایه شده، مجموعه داده هایی از تصاویر پلاک های ایرانی در شرایط مختلف جمع آوری شده است. نتایج ارزیابی ها نشان می دهد که رویکردهای ارایه شده به خوبی توانسته است، نرخ بازیابی نواحی پلاک و دقت ناحیه یافته شده را بهبود داده و نرخ هشدار غلط را نیز به خوبی کاهش دهد.
کلیدواژگان: پلاک های ایرانی، شناسایی پلاک، طبقه بندی کننده سلسله مراتبی، آموزش، آزمایش دو مرحله ای -
صفحات 91-108
فیلتر منطبق در گیرنده رادار فقط با نسخه سیگنال ارسالی تطبیق دارد و به دلیل عدم تطبیق با سیگنال دریافتی از محیط، خروجی آن دچار تلف می شود. دامنه گلبرگ های جانبی خروجی فیلتر منطبق در رادارهای مجهز به فشرده سازی پالس به شکل موج کدشده ارسالی وابسته است که به اندازه طول کد در دو طرف موقعیت هدف گسترده می شوند. برای آشکارسازی یک هدف ضعیف در مجاورت یک هدف قوی، گلبرگ های جانبی خروجی فیلتر منطبق ناشی از هدف قوی سبب پوشانندگی هدف ضعیف و عدم آشکارسازی آن می شود. به طورمعمول گستره پویایی رادار براساس نسبت بیشینه توان دریافتی به کمینه توان قابل آشکارسازی تعریف می شود که به سطح آستانه و گلبرگ های جانبی وابسته هستند. الگوریتم های وفقی با شرط حفظ تفکیک پذیری برد موجب کاهش سطح گلبرگ های جانبی تا سطح نوفه شده و در نتیجه گستره پویایی را افزایش می دهند. در این مقاله یک الگوریتم وفقی بهبودیافته (از نظر بارمحاسباتی و مقاومت در برابر دوپلر) مبتنی بر تخمین گر کمینه میانگین مربعات خطا (MMSE) به نام الگوریتم مرمت فشرده سازی پالس وفقی با طول فیلتر منعطف (FFL-APCR) پیشنهاد می شود، که طول فیلتر در آن وابسته به طول کد ارسالی است. همچنین نشان داده می شود که طول کد ارسالی در تعیین حد مجانبی پیک گلبرگ های جانبی و گستره پویایی تاثیرگذار است؛ به علاوه تاثیر سرعت زیاد هدف بر پهن شدگی گلبرگ اصلی و تنزل عملکرد فیلترهای وفقی بررسی می شود؛ در نهایت افزایش گستره پویایی رادار با الگوریتم پیشنهادی FFL-APCR در شرایط مختلف نشان داده و عملکرد آن با معیار میانگین مجذور خطا (MSE) ارزیابی می شود.
کلیدواژگان: گستره پویایی، فیلتر منطبق، فشرده سازی پالس وفقی، حداقل میانگین مربعات خطا تکراری، الگوریتم مرمت فشرده سازی پالس وفقی با طول فیلتر منعطف -
صفحات 109-126
با توجه به گسترش روزافزون پژوهش های علوم شناختی، کدگشایی مغز انسان یک موضوع داغ در حوزه علوم عصبشناسی محاسباتی است. در این راستا پژوهش های متعددی جهت ارایه روشی کارا و موثر برای کدگشایی فعالیت مغز انسان با پردازش دادگان fMRI در حال انجام است. خروجی این روشها به طورعمومی معطوف به ارایه یک مدل محاسباتی تعمیمیافته است که امکان تشخیص سیگنال مغزی و تعلق آن به عامل محرک (شیء دیداری) را ارایه میدهد. دادگان مغزی دارای ابعاد زمانی و فضایی زیادی هستند که سبب افزایش تعداد ویژگی ها نیز می شود. همچنین استخراج ویژگیهای مفید از تصاویر مغزی مانند fMRI کاری پیچیده است. این امر سبب طولانی شدن عمل هم گرایی در الگوریتمهای یادگیری برای ایجاد مدل مناسب می شود؛ با توجه به چالش های یادشده، روش های یادگیری گروهی چندوجهی یک پیشنهاد مناسب برای حل مساله کدگشایی مغز محسوب می شود که تلفیقی مناسب بین ویژگیهای عملکردی متفاوت در دادگان مغزی ایجاد می کند. در روش پیشنهادی داده های آموزشی بر اساس اطلاعات متقابل در فضای ویژگی خوشه بندی می شوند، به صورتی که فضای ویژگی به چند وجه تفکیک می شود؛ سپس روی هر وجه ویژگی یک مدل ماشین بردار پشتیبان به صورت موازی آموزش داده می شود. در مرحله آزمون، فضای ویژگی دادگان آزمون نیز به صورت مشابه دادگان آموزش تقسیم می شود؛ و هر بردار ویژگی به مدل مربوطه تخصیص داده خواهد شد. از هر مدل یک بردار احتمالاتی تولید می شود و با هم جوشی این بردارها ماتریس پروفایل تصمیم گیری ساخته خواهد شد؛ درنهایت عملگرهای وزن دار مرتب شده اعمال می شود. جهت بررسی کارایی رویکرد پیشنهادی از رویکرد اعتبار سنجی متقابل با سناریوی درون فردی استفاده شده است. معیارهایی مانند صحت و ماتریس درهم ریختگی برای ارزیابی مدل، به کار برده شده است. با بررسی عملکرد مدل بر روی هر وجه ویژگی، می توان دقت دستهبندی با میانگین بیش از 50% را به دست آورد؛ اما در مدل گروهی نظارتی متوسط صحت تشخیص به بیش از 90 درصد می رسد.
کلیدواژگان: اطلاعات متقابل، تصویربرداری تشدید مغناطیسی عملکردی fMRI، کدگشایی مغز، همجوشی تصمیم ها، یادگیری گروهی -
صفحات 127-146
تاکنون روش های مختلفی برای بهینه سازی ارایه شده است و یکی از معروف ترین روش های بهینه سازی، الگوریتم های هوش جمعی هستند. بسیاری از مسایل بهینه سازی اخیر در دنیای واقعی طبیعت پویا دارند؛ بنابراین، الگوریتم بهینه سازی برای حل مسایل در محیط های پویا مورد نیاز است. الگوریتم دسته والد-فرزند مبتنی بر حافظه و خوشه بندی (CMPCS)، گونه ای از الگوریتم های هوش جمعی و برگرفته شده از طبیعت است، که در این مقاله ارایه شده است. این روش به رفتار فردی و گروهی وابسته است، در این الگوریتم برای افزایش کارآیی از یک حافظه با خوشه بندی و دافعه استفاده شده است. روش CMPCS پیشنهاد شده بر روی محک قله های متحرک (MPB) آزمایش شده است. MPB یک محک خوب برای ارزیابی کارایی الگوریتم های بهینه سازی در محیط های پویا است. نتایج تجربی در MPB نشان می دهد که روش پیشنهادی CMPCS کارایی مناسب تری نسبت به روش های دیگر حل مسایل بهینه سازی پویا دارد.
کلیدواژگان: بهینه سازی پویا، محیط های پویا، حافظه، محک قله های متحرک -
صفحات 147-160
سامانه های حمل و نقل دریایی بخش مهمی از ترابری جهانی را شامل می شوند. سامانه های نظارتی در صنایع دریانوردی و کشف اهداف دریایی از اهمیت به سزایی در کاربردهای نظامی و تجاری برخوردار است. افزایش روزافزون ترابری دریایی موجب علاقه پژوهش گران به توسعه روش های نظارتی هوشمند در زمینه ترابری دریایی شده است. به دلیل وجود کلاترها، مه و گرد و غبار در دریا، تصاویر حرارتی نسبت به تصاویر مریی در این زمینه، از کارایی و دقت تشخیص بالاتری برخوردار هستند. در این مقاله، یک روش برای کشف اهداف دریایی در تصاویر نوفه ای حرارتی ارایه شده است. روش پیشنهاد شده شامل دو مرحله آشکارسازی خط افق در تصویر و سپس کشف اهداف است. ابتدا خط افق با استفاده از روش بیشینه گیری از تصویر گرادیان و برازش خط آشکار، سپس یک ناحیه مشخص برای جستجوی اهداف دریایی حول خط افق انتخاب می شود. محدود کردن ناحیه جستجو باعث افزایش سرعت روش پیشنهادی و کاهش هشدارهای کاذب می شود. در مرحله دوم، ناحیه انتخابی بلوک بندی می شود و از هر بلوک تعدادی ویژگی استخراج می شود. این ویژگی ها به چندین دسته بند متعارف داده و نتایج آنها به یک تصمیم ساز فازی نوع دوم بازه ای داده می شود تا با ترکیب این نتایج در مورد تعلق بلوک به ناحیه هدف یا پس زمینه تصمیم گیری نهایی را انجام دهد؛ در نهایت اهداف مورد نظر از تجمیع این بلوک ها و حذف موارد ناخواسته کشف می شوند. مقادیر شاخصه های ارزیابی دقت، صحت و فراخوان سیستم پیشنهادی روی پایگاه داده به ترتیب 59/97%، 19/96% و 92/97% بوده که نسبت به سایر روش های مقایسه شده، مقادیر بالاتری را گزارش داده است. نتایج به دست آمده نشان می دهد که در روش پیشنهادی، خط افق با حجم محاسباتی کم و با دقت خوبی آشکار شده و در نهایت اهداف دریایی مورد نظر با دقت بالایی کشف می شوند.
کلیدواژگان: کشف اهداف، آشکارسازی خط افق، استخراج ویژگی، دسته بند، سیستم استنتاج فازی
-
Pages 3-18
Digital transformers are considered as one of the digital circuits being widely used in signal and data processing systems, audio and video processing, medical signal processing as well as telecommunication systems. Transforms such as Discrete Cosine Transform (DCT), Discrete Wavelet Transform (DWT) and Fast Fourier Transform (FFT) are among the ones being commonly used in this area. As an illustration, the DCT is employed in compressing the images. Moreover, the FFT can be utilized in separating the signal spectrum in signal processing systems as fast as possible. The DWT is used in separating the signal spectrum in a variety of applications from signal processing to telecommunication systems, as well. In order to build a VLSI circuit, several steps have to be taken from chip design to final construction. The first step in the synthesis of the integrated circuits is called high-level synthesis (HLS), in which a structural characteristic is obtained from a behavioral or algorithmic description. The resulting structural characteristic is equivalent to the one being considered in the behavioral description and it somehow represents the method for implementing the behavioral description as a result several structural descriptions could be implementable for each behavioral description. Therefore, depending on the intended use, the characteristic will be selected that outperforms the others. The main purpose of the HLS is to optimize the power consumption, the chip occupied area and delayed and is fulfilled by selecting the appropriate number of operating units and how they are implemented to the operators. This is generally accomplished through a graph analysis called the data flow graph (DFG) which is a graphical representation of the type and how the operators connect. In the DFG, each node is equivalent to an operator while the edges represent the relationship between these operators. Experience has proved that if the level of design optimization is high, in addition to higher efficiency, the design time will be lower, which is why the researchers are far more interested in optimization at higher levels of design than the lower levels. The complex, extensive, and discrete nature of the HLS problems have been ranked them among the most complex problems in VLSI circuits engineering. Bearing this mind, using meta-heuristic and Swarm intelligence methods to solve high-level synthesis projects seems to be a favored option. In this paper, a heuristic method called Moth-Flame Optimization (MFO) has been used to solve the HLS problem in the design of digital transformer to find the optimal response. The MFO is a population-based heuristic algorithm that optimizes the problems using the laws of nature. The leading notion behind the MFO algorithm inspired from the moths’ movements and their instinctive navigation during the night. In the MFO algorithm, the moths are like chromosomes in the GA and like the particles in the PSO algorithm. In order to compare and prove the efficiency of the proposed method, it was applied on the test data with the GA-based method separately but with the same initial conditions. The comparative results along with the results of the GA-based method demonstrated that the proposed method exhibits a higher ability to provide the appropriate hardware structure and high-level synthesis of various types of transformers. Another outstanding feature of the proposed method is its high speed of finding an optimal response with an average of more than 20% greater than the GA based method.
Keywords: High-Level Synthesis, Datapath, Digital Transformers, Meta-heuristic Algorithm, MFO Algorithm -
Pages 19-28
Stochastic gradient-based adaptation algorithms have received a great attention in various applications. The most well-known algorithm in this category is the Least Mean Squares (LMS) algorithm that tries to minimize the second-order criterion of mean squares of the error signal. On the other hand, it has been shown that higher-order adaptive filtering algorithms based on higher-order statistics can perform better in many applications, particularly in the presence of intense noises. However, these algorithms are more prone to instability and also their convergence rates decline in the vicinity of their optimum solutions. In attempt to make use of the useful aspects of these algorithms, it has been proposed to combine the second-order criterion with higher-order ones, e.g. that of the Least Mean Fourth (LMF) algorithm. The Least Mean Mixed-Norm (LMMN) algorithm is a stochastic gradient-based algorithm which aim is to minimize an affine combination of the cost functions of the LMS and LMF algorithms. This algorithm has inherited many properties and advantages of the LMS and the LMF algorithms and mitigated their weaknesses in some ways. These advantages are achieved at the cost of the additional computation burden of just one addition and four multiplications per iteration. The main issue of the LMMN algorithm is the lack of an analytical model for predicting its behaviour, the fact that has restricted its practical application. To address this issue, an analytical model is presented in the current paper that is able to predict the mean-square-error and the mean-weights-error behaviour with a high accuracy. This model is derived using the Isserlis’ theorem, based on two mild and practically valid assumptions; namely the input signal is stationary, zero-mean Gaussian and the measurement noise are additive zero-mean with an even probability distribution function (pdf). The accuracy of the derived model is verified using several simulation tests. These results show that the model is of a high accuracy in various settings for the noise’s power level and distribution as well as the unknown filter characteristics. Furthermore, since the LMF and the LMS algorithms are special cases of the more general LMMN algorithm, the proposed model can also be used for predicting the behaviour of these algorithms.
Keywords: Adaptive Algorithms, LMMN Algorithm, Analytical Model -
Pages 29-44
Nowadays, crypto-ransomware is considered as one of the most threats in cybersecurity. Crypto ransomware removes data access by encrypting valuable data and requests a ransom payment to allow data decryption. The number of Crypto ransomware variants has increased rapidly every year, and ransomware needs to be distinguished from the goodware types and other types of ransomware to protect users' machines from ransomware-based attacks. Most published works considered System File and process behavior to identify ransomware which depend on how quickly and accurately system logs can be obtained and mined to detect abnormalities. Due to the severity of irreparable damage of ransomware attacks, timely detection of ransomware is of great importance. This paper focuses on the early detection of ransomware samples by analyzing behavioral logs of programs executing on the operating system before the malicious program destroy all the files. Sequential Pattern Mining is utilized to find Maximal Sequential Patterns of activities within different ransomware families as candidate features for classification. First, we prepare our test environment to execute and collect activity logs of 572 TeslaCrypt samples, 535 Cerber ransomware, and 517 Locky ransomware samples. Our testbed has the capability to be used in other projects where the automatic execution of malware samples is essential. Then, we extracted valuable features from the output of the Sequence Mining technique to train a classification algorithm for detecting ransomware samples. 99% accuracy in detecting ransomware instances from benign samples and 96.5% accuracy in detecting family of a given ransomware sample proves the usefulness and practicality of our proposed methods in detecting ransomware samples.
Keywords: malware, ransomware, crypto ransomware, ransomware detection, ransomware family detection -
Pages 45-64
Today, online social media with numerous users from ordinary citizens to top government officials, organizations, artists and celebrities, etc. is one of the most important platforms for sharing information and communication. These media provide users with quick and easy access to information so that the content of shared posts has the potential to reach millions of users in a matter of seconds. Twitter is one of the most popular and practical/used online social networks for spreading information, which, while being reliable, can also, be a source for spreading unrealistic and deceptive rumors as a result can have irreversible effects on individuals and society. Recently, several studies have been conducted in the field of rumor detection and verify using models based on deep learning and machine learning methods. Previous research into rumor detection has focused more on linguistic, user, and structural features. Concerning structural features, they examined the retweet propagation graph. However, in this study, unlike the previous studies, new structural features of the reply tree and user graph in extracting rumored conversations were extracted and analyzed from different aspects. In this study, the effectiveness of new structural features related to reply tree and user graph in detecting rumored conversations in Twitter events were evaluated from different aspects. First, the structural features of the reply tree and user graph were extracted at different time intervals, and important features in these intervals were identified using the Sequential Forward Selection approach. To evaluate the usefulness of valuable new structural features, these features have been compared with consideration of linguistic and user-specific features. Experiments have shown that combining new structural features with linguistic and user-specific features increases the accuracy of the rumor detection classification. Therefore, a rumor classification algorithm based on new structural, linguistic, and user-specific features in rumor conversation detection was proposed. This algorithm performs better than the basic methods and detects rumored conversations with greater accuracy. In addition, due to the importance of the source tweet user in conversations, this user was examined and analyzed from different aspects. The results showed that most rumored conversations were started by a small number of users. Rumors can be prevented by early identification of these users on Twitter events.
Keywords: Conversion, Rumor detection, Twitter, Reply tree, User graph -
Pages 65-76
Recently, the problem of compressive sensing (CS) has attracted lots of attention in the area of signal processing. So, much of the research in this field is being carried out in this issue. One of the applications where CS could be used is wireless sensor networks (WSNs). The structure of WSNs consists of many low power wireless sensors. This requires that any improved algorithm for this application must be optimized in terms of energy consumption. In other words, the computational complexity of algorithms must be as low as possible and should require minimal interaction between the sensors. For such networks, CS has been used in data gathering and data persistence scenario, in order to minimize the total number of transmissions and consequently minimize the network energy consumption and to save the storage by distributing the traffic load and storage throughout the network. In these applications, the compression stage of CS is performed in sensor nodes, whereas the recovering duty is done in the fusion center (FC) unit in a centralized manner. In some applications, there is no FC unit and the recovering duty must be performed in sensor nodes in a cooperative and distributed manner which we have focused on in this paper. Indeed, the notable algorithm for this purpose is distributed least absolute shrinkage and selection operation (D-LASSO) algorithm which is based on diffusion cooperation structure. This algorithm that compete to the state-of-the-art CS algorithms has a major disadvantage; it involves matrix inversion that may be computationally demanding for sufficiently large matrices. On this basis, in this paper, we have proposed a distributed CS recovery algorithm for the WSNs with a bi-directional incremental mode of cooperation. Actually, we have proposed a comprehensive distributed framework for the recovery of sparse signals in WSNs. Here, we applied this comprehensive structure to three problems with different constraints which results in three completely distributed solutions named as distributed bi-directional incremental basis pursuit (DBIBP), distributed bi-directional incremental noise-aware basis pursuit (DBINBP) and distributed bi-directional incremental regularized least squares (DBIRLS). The proposed algorithms solely involve linear combinations of vectors and soft thresholding operations. Hence, the computational load is significantly reduced in each sensor. In the proposed method each iteration consists of two phases; clockwise and anti-clockwise phases. At each iteration, in anti-clockwise phase, each node receives the local estimate from its previous neighbor and updates an auxiliary variable. Then in the clockwise phase, each node receives the updated auxiliary variable from its next neighbors to update the local estimate. On the other hand, information exchange in two directions in an incremental manner which we called it bi-directional incremental structure. In an incremental strategy, information flows in a sequential manner from one node to the adjacent node. Unlike the diffusion structure (like as D-LASSO) where each node communicates with all of their neighbors, the incremental mode of cooperation requires the least amount of communication and power. The low computational complexity and better steady state performance are the important features of the proposed methods.
Keywords: Wireless sensor networks, Sparse signal, Bi-directional incremental topology, Compressive sensing, Recovery algorithm -
Pages 77-90
Automatic license plate recognition is widely used in intelligent transport systems to automatically and quickly read license plates of vehicles. A license plate recognition is a computer vision system containing three main steps: plate detection, character segmentation, and character recognition. The first and foremost step of this system is the plate detection stage where the plate is located from the input image. This step has many challenges, including low-resolution images, illumination change, complex background, multiple plates, and different plate sizes. The plate detection methods can be categorized into connected component-based, color-based, and classifier-based methods. The two first approaches are not reliable in real-world environments, and they are too sensitive to illumination change and plate sizes compared to the classifier-baed techniques. In this context, the Cascade classifier has successfully been applied to various object detection problems. This classifier sequentially combines several weak classifiers based on the AdaBoost Algorithm. In this paper, an effective Iranian vehicle license plate detection approach is developed based on a cascade classifier. A two-phase training approach is proposed to enhance the cascade classifier by getting feedback from the negative data. Then, a new testing approach is suggested to reduce false-positive detection and improve detection precision. We also collected an Iranian license plate dataset which is publicly available for research purposes. The dataset covers images in different real-world conditions. The proposed system evaluated on this dataset is able to be applied on gray as well as color images, in a way that can detect multiple plates at front or rear of the cars in different illuminations. Moreover, the proposed method is invariant to the size, position or where the plates are located in the image. The experimental results provided in different conditions show that the proposed approach can improve the precision and recall rate while reducing the false-positive rate.
Keywords: Iranian license Plate, Plate detection, Cascade classifier, Two-phase training, testing -
Pages 91-108
The matched filter in the radar receiver is only adapted to the transmitted signal version and its output will be wasted due to non-matching with the received signal from the environment. The sidelobes amplitude of the matched filter output in pulse compression radars are depended on the transmitted coded waveforms that extended as much as the length of the code on both sides of the target location. In order to detect a weak target in vicinity of strong target, the sidelobes of the matched filter output resulting from the strong target masked the weak target and didn’t detect its. Generally, the radar dynamic range is defined by the maximum power ratio to the minimum detectable power that is depended on the level of the threshold and the sidelobe levels. Adaptive algorithms suppress the sidelobe levels to noise level with condition of maintain the range resolution and therefore increase the dynamic range. In this paper, an improved algorithm (in terms of computational cost and Doppler robustness) is proposed based on the minimum mean square error (MMSE) estimator denoted as Flexible Filter Length-Adaptive Pulse Compression Repair (FFL-APCR), which filter length depends on the length of transmitted code. It is also shown that the length of the code is influenced by determining the asymptotic peak sidelobe level and the dynamics range. In addition, the influence of the high-speed target on main lobe broadening and the performance degradation of adaptive filters is investigated. Finally, extending of radar dynamic range with the proposed FFL-APCR algorithm is shown in various conditions and its performance evaluated by mean square error criteria.Where return signals coincide with the transmission of a pulse, pulse eclipsing can occur which results in detection performance loss. The mismatches (Doppler phase shift and pulse eclipsing) degrades performance of sidelobes suppression algorithms. The FFL-APCR algorithm suppresses range sidelobes by using a smaller filter length and reduces the computational cost. Consequently, this algorithm should be computationally efficient (real-time) to enable the practical application of RMMSE.
Keywords: Dynamic range, Matched Filter, Adaptive Pulse Compression, Minimum Mean Square Error (MMSE), Flexible Filter Length-Adaptive Pulse Compression Repair (FFL-APCR) algorithm -
Pages 109-126
In the past two decades, the applications of computational neuroscience have been increasingly growing. Breaking the neural code is a crucial open problem in computational neuroscience. Various research groups attempt to provide an efficient method to decode human brain activity using fMRI data. The output of these methods is a computational model that can assign brain signals to an external stimulus; in this study, visual object recognition has been investigated. The brain decoders are used in many applications, such as the brain-computer interface or detecting specific mental illnesses. In general, brain fMRI data have a high spatial and temporal resolution that increases the number of features of the problem. Proper feature extraction from brain images is a challenging and time-consuming process. Consequently, the convergence of learning algorithms takes a long time to create an appropriate model. So, breaking down the feature space is highly recommended. We proposed new multi-view learning to solve the brain decoding problem. This approach splits the feature space based on mutual information and finds an appropriate ensemble classification model that detects the related visual object to neural activities in the brain.The proposed method clusters the feature space based on mutual information and splits it into coherent sub-spaces, views. For each feature view, a support vector machine model is learned in parallel; the used SVM version can generate a vector of probabilities for each class. At the test phase, the feature space of test data is divided similarly to the training data, and each model generates a probabilistic vector for the test instances. Then, these vectors are combined in the decision profile matrix. The decision fusion is employed by the ordered weighted averaging (OWA) approach. The proposed multi-view learning methods achieved higher accuracy rates than the single view model. The main advantage of the MV model is that it can run in parallel, making it counterproductive to deal with the high-dimensional problems based on the divide and conquer strategy. The optimization phase to detect the most acceptable parameters for each model is obtained using the simulated annealing, SA, algorithm. We have employed three real fMRI datasets of the human brain to assess the proposed method, obtained from the Openneuro website. Also, the leave-one-run-out cross-validation approach has been carried out to evaluate the proposed method in the intra-subject scenario. Criteria such as accuracy rate and confusion matrix have been undertaken to analyze the results. The single feature view obtains an accuracy rate of more than 50%. While in the ensemble model, the accuracy rate in most subjects is more than 90%.
Keywords: Brain Decoding, Decision Fusion, Ensemble learning, fMRI, Mutual Information -
Pages 127-146
In the real world, we face some complex and important problems that should be optimized, most of the real-world problems are dynamic. Solving dynamic optimization problems are very difficult due to possible changes in the location of the optimal solution. In dynamic environments, we are faced challenges when the environment changes. To respond to these changes in the environment, any change can be considered as the input of a new optimization problem that should be solved from the beginning, which is not suitable because it is time consuming. One technique for improving optimization and learning in dynamic environments is by using information from the past. By using solutions from previous environments, it is often easier to find promising solutions in a new environment. A common way to maintain and exploit information from the past is the use of memory, where solutions are stored periodically and can be retrieved and refined at the time that the environment changes. Memory can help search respond quickly and efficiently to change in a dynamic problem. Given that a memory has a finite size, if one wishes to store new information in the memory, one of the existing entries must be discarded. The mechanism used to decide whether the candidate entry should be included in the memory or not, and if so, which of the old entries should be replaced it, is called the replacement strategy. This paper explores ways to improve memory for optimization and learning in dynamic environments. In this paper, a memory with clustering and new replacement strategy for storing and restoring memory solutions has been used to enhance memory performance. The evolutionary algorithms that have been presented so far have the problem of rebuilding populations when multiple populations converge to an optimum. For this reason, we proposed algorithm with exclution mechanism that have the ability to explore the environment (Exploration) and extraction (Explitation). Thus, an optimization algorithm is required to solve the problems in dynamic environments well. In this paper, a novel collective optimization algorithm, namely the Clustering and Memory-based Parent-Child Swarm Algorithm (CMPCS), is presented. This method relies on both individual and group behavior. The proposed CMPCS method has been tested on the moving peaks benchmark (MPB). The MPB is a good Benchmark to evaluate the efficiency of the optimization algorithms in dynamic environments. The experimental results on the MPB reveal the appropriate efficiency of the proposed CMPCS method compared to the other state-of-the-art methods in solving the dynamic optimization problems.
Keywords: Dynamic Optimization, Dynamic Environments, Memory, Moving Peaks Benchmark -
Pages 147-160
Maritime transportation system is a vital part of the world conveyance. The surveillance in maritime industry and detecting marine targets have a great impact on military and commercial applications. Daily increase in maritime zone encourages the researchers to develop intelligent surveillance approaches in the maritime transportation. The sensing methods generally include visual and infrared cameras, sensors, and radars. Cameras are widely used since they capture high resolution images than sensors and traditional radars. Also, applying complex pattern recognition techniques and decision-making processes to the camera images provides more accurate detection results. Due to the clutters, dust, and weather changes in the sea including the rainfall, snowfall, and heavy fog, the image quality taken by the visual cameras is drastically deteriorated. Also, detecting the sea targets -specially the small ones- and similarly the sea-sky horizon line becomes more challenging. In such situations, the infrared images reveal higher performance and accuracy in comparison with visible images. The sea-sky horizon line detection of noisy infrared images in small target detection algorithms with high intensity and low SNR is of great importance in maritime surveillance. Determining the horizon line simplifies the target detection by restricting the search area for the targets in the image. This task decreases the computation time and mistakes in the detection. This paper presents a method for detecting marine targets in noisy infrared images. The proposed method includes two steps of detecting the sea-sky horizon line and finding the targets. In the first step, the two-dimensional gradient of the image is computed, from which it is observed that the most variations are appeared at the edge points. With respect to this remark, the maximum of each column of the gradient image is found and the obtained values for all columns and corresponding rows’ numbers are kept in a set, namely the maximum pixels set. Then, to find the sea-sky horizon line, on the first and the last 75 pixels in the mentioned set, a straight line is fitted along the image width. Afterwards, to search for the objects, a region of interest is selected around the detected line. Restricting the search region increases the speed of the proposed method and decreases the number of false alarms. In the second step, this region is partitioned into some separate blocks; from each, multiple features are extracted. These features are fed into multiple classifiers whose outputs are given to a decision-making algorithm based on the interval type-II fuzzy fusion system. This system decides to which class (target or background) that block belongs. Finally, the objects are found by integrating the target blocks and removing the unwanted ones. To evaluate the proposed method, first an image dataset was generated using an infrared camera with medium wavelength in different situations. This was done due to no access to a complete infrared sea image bank. Sea infrared images were commonly corrupted by a combination of noises including the salt-and-pepper, Gaussian noise or electronic noises due to the detector of camera image supply. In order to attenuate these noises, a 3×3 median filter was applied to the raw image. Afterwards, to increase the image contrast, the histogram equalization method was performed. Finally, the proposed approach was run to find the marine targets in the enhanced image. The results demonstrated that the sea-sky horizon line was detected with low computational complexity and high accuracy while targets were also found with desirable detection rates.
Keywords: Targets detection, Sea-sky horizon line detection, Feature extraction, Classifier, fuzzy inference system