فهرست مطالب
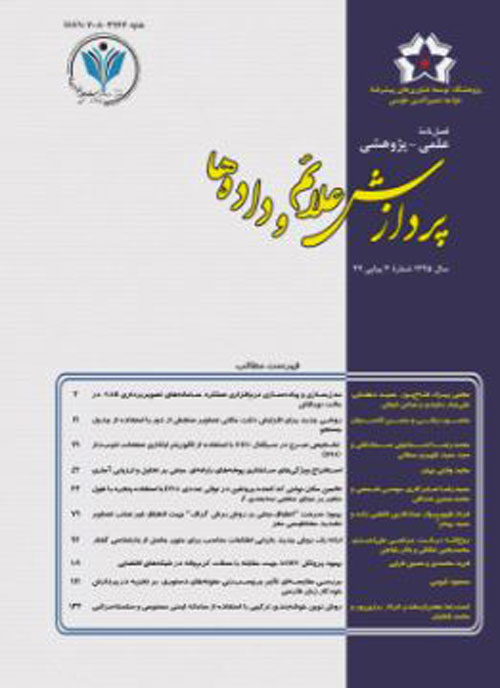
فصلنامه پردازش علائم و داده ها
سال هجدهم شماره 4 (پیاپی 50، زمستان 1400)
- تاریخ انتشار: 1401/02/07
- تعداد عناوین: 10
-
-
صفحات 3-22
به دلیل اهمیت بالای کیفیت داده ها در عملکرد سامانه های نرم افزاری، فرآیند پاکسازی داده به خصوص تشخیص رکوردهای تکراری، طی سالیان اخیر یکی از مهم ترین حوزه های علوم رایانه به حساب آمده است. در این مقاله روشی برای تشخیص رکوردهای تکراری ارایه شده است که با خوشه بندی سلسله مراتبی رکوردها بر اساس ویژگی های مناسب در هر سطح، میزان شباهت میان رکوردها تخمین زده می شود. این کار سبب می شود تا خوشه هایی در سطح آخر به دست آیند که رکوردهای درون آن ها بسیار مشابه یکدیگر باشند. برای کشف رکوردهای تکراری نیز مقایسه تنها بر روی رکوردهای درون یک خوشه از سطح آخر انجام می گیرد. همچنین در این مقاله برای مقایسه میان رکوردها، یک تابع تشابه نسبی بر پایه تابع فاصله ویرایشی ارایه شده که دقت بسیار بالایی به همراه دارد. مقایسه نتایج ارزیابی سامانه نشان می دهد که روش ارایه شده، در زمان کمتری، 90% تکراری های موجود را با دقت 97% کشف می کند و بهبود داشته است.
کلیدواژگان: تشخیص تکراری، پاک سازی داده، خوشه بندی سلسله مراتبی، تابع تشابه، انتخاب ویژگی -
صفحات 23-35
امروزه مساله مسیریابی وسایل نقلیه، یکی از موضوعات پرکاربرد در موضوعات صنعتی، نظامی و حتی امنیتی است و برای افزایش کارایی و بهره وری سامانه های حمل و نقل تعریف شده است. مساله مسیریابی وسیله نقیله با شرایط برداشت و تحویل هم زمان محموله از جمله این مسایل است. این مساله از نظر پیچیدگی محاسباتی در مجموعه مسایل سخت (NP-hard) قرار می گیرد؛ بنابراین محاسبه بهترین پاسخ برای این مساله، در زمان محاسباتی نمایی انجام خواهد شد و در مسایل اجرایی قابل استفاده نخواهد بود. استفاده از الگوریتم های فراابتکاری یکی از روش هایی است که به وسیله آنها می توان جواب هایی مناسب و در زمان محاسباتی قابل قبول به دست آورد. در روش های موجود، قیود موجود در مساله، با استفاده از روش جریمه به تابع هدف مساله اضافه شده و مساله بهینه سازی تک هدفه تعریف می شود. ضمن این که تعداد بهینه وسایل نقلیه مورد نیاز برای حل مساله در نظر گرفته نمی شود. در این مقاله، الگوریتم جست وجوی گرانشی بهبود یافته برای حل مسایل مقید معرفی شده است. همچنین به منظور کنترل قابلیت های الگوریتم نظیر کاوش و بهره وری از یک کنترلر فازی برای تعیین پارامترهای موجود در الگوریتم استفاده شده، سپس، با استفاده از این الگوریتم، روشی برای حل مساله مسیریابی وسایل نقلیه با شرایط برداشت و تحویل هم زمان ارایه شده است. با استفاده از این روش، علاوه بر محاسبه مسیرهای مناسب برای انجام خدمات، تعداد بهینه وسایل نقلیه برای فرآیند خدماتی نیز تعیین می شود. برای ارزیابی کارایی روش پیشنهادی در این مقاله، روش پیشنهادی شبیه سازی شده و روی مجموعه داده استانداردی که برای این دسته از مسایل تعریف شده، اجرا شده است. نتایج تجربی و شبیه سازی نشان می دهد که این روش، با وجود سادگی در روش پیاده سازی و اجرا، دارای کارایی بهتری نسبت به الگوریتم ها و روش های بررسی شده است.
کلیدواژگان: مسیریابی وسایل نقلیه، برداشت و تحویل هم زمان، الگوریتم های فراابتکاری، الگوریتم جست وجوی گرانشی مقید -
صفحات 37-47
از روش های خوشه بندی برای بازیابی ساختار نرم افزار جهت فهم درست آن و همچنین بازسازی نرم افزار استفاده می شود. در ادبیات موضوع، بیشتر الگوریتم های ارایه شده برای خوشه بندی سامانه های نرم افزاری به دو دسته الگوریتم های مبتنی بر جستجو و الگوریتم های سلسله مراتبی طبقه بندی می شوند و الگوریتمی از رده مبتنی بر افراز برای خوشه بندی یک سامانه نرم افزاری ارایه نشده است. این روش ها سعی دارند که گراف وابستگی موجودیت به دست آمده از کد منبع سامانه نرم افزاری را به چند مجموعه راسی افراز کنند. در سامانه های نرم افزاری، موجودیت می تواند رده، تابع و یا یک فایل باشد. با توجه به چندجمله ای غیر قطعی، سخت بودن مساله خوشه بندی، در سال های اخیر از روش های تکاملی و مبتنی بر جستجو مانند الگوریتم ژنتیک برای این حل این مساله، زیاد استفاده شده است. هر چند این الگوریتم ها در برخی موارد می توانند ساختار مناسبی از نرم افزار را به دست آورند، اما برای نرم افزار های با ابعاد بزرگ، با توجه به زمان اجرا و حافظه مصرفی زیاد، قابل اجرا نیستند؛ همچنین، این روش ها از اطلاعات و دانش گرافی موجود در گراف وابستگی موجودیت استفاده ی چندانی نمی کنند. در این مقاله یک الگوریتم مبتنی بر افراز ارایه شده است که بتوان از آن در خوشه بندی نرم افزار نیز استفاده کرد. همچنین، یک نوع فاصله جدید برای قیاس تشابه و عدم تشابه ارایه شده است. انتظار می رود روش پیشنهادی بتواند در قیاس با سایر روش های موجود، خوشه بندی هایی با کیفیت بالاتر و نزدیک به خوشه بندی فرد خبره، تولید کند. برای بررسی صحت اجرای الگوریتم، آن را بر روی نرم افزار موزیلا فایرفاکس اجرا کرده و نتایج را با الگوریتم های مطرح این حوزه، مقایسه کرده ایم.
کلیدواژگان: مهندسی نرم افزار، مهندسی معکوس، خوشه بندی نرم افزار، الگوریتم K-means -
صفحات 49-68
با افزایش روز افزون تصاویر، اندیس گذاری و جستجوی سریع آنها در پایگاه داده های بزرگ، یک امر ضروری است. یکی از راه کارهای موثر، نسبت دادن یک یا چند برچسب به هر تصویر با هدف توصیف محتوای درون آن است. با وجود کارایی روش های خودکار برچسب زنی، یکی از چالش های اساسی آنها مقیاس پذیری با افزایش تصاویر پایگاه داده است. در این مقاله، با هدف حل این چالش، ابتدا براساس توصیف گر بصری تصاویر که از شبکه های یادگیری عمیق استخراج می شوند، نمایندگان مناسبی به دست می آیند. سپس، با استفاده از رویه انتشار برچسب بر روی گراف، برچسب های معنایی از تصاویر آموزشی به نمایندگان منتشر می شوند. با این راه کار، به یک مجموعه نمایندگان برچسب دار دست خواهیم یافت که می توان عمل برچسب زنی هر تصویر آزمون را بر اساس این نمایندگان انجام داد. برای برچسب زنی، یک رویکرد مبتنی بر آستانه گذاری وفقی پیشنهاد شده است. با روش پیشنهادی، می توان اندازه مجموعه داده آموزشی را به 6/22 درصد اندازه اولیه کاهش داد که منجر به تسریع حداقل 2/4 برابری زمان برچسب زنی خواهد شد. همچنین، کارایی برچسب زنی بر روی مجموعه داده های مختلف برحسب سه معیار دقت، یادآوری و F1 در حد مطلوبی حفظ شده است.
کلیدواژگان: خلاصه سازی پایگاه داده، برچسب زنی تصویر، روش مبتنی برجستجو، مقیاس پذیری -
صفحات 69-80
با گسترش اینترنت و فضای وب، برقراری ارتباط و کسب اطلاعات در بین افراد از شکل سنتی و اولیه خود فاصله گرفته و به درون تارنماها کشیده شده است. همچنین فضای جهانی وب، فرصت بزرگی را برای کسب و کارها فراهم می کند تا ارتباط خود را با مشتری بهبود ببخشند و بازار خود را در دنیای برخط گسترش دهند. کسب و کارها برای بررسی میزان بازدید و محبوبیت سایت هایشان از معیاری به نام رتبه بندی ترافیکی استفاده می کنند. رتبه بندی ترافیکی میزان بازدیدکنندگان یک سایت را اندازه گرفته و براساس همین آمار، رتبه ای را به سایت اختصاص می دهد. یکی از مهم ترین چالش های موجود در رتبه بندی، ایجاد ترافیک جعلی تولید شده به وسیله برنامه های کاربردی به نام ربات است. ربات ها اجزای نرم افزاری مخرب مورد استفاده برای تولید هرزنامه ها، راه اندازی حملات مختل کننده سامانه، فیشینگ، سرقت هویت و خروج اطلاعات و دیگر فعالیت های غیر قانونی هستند تاکنون روش های مختلفی برای شناسایی و کشف ربات صورت گرفته است. در این پژوهش، شناسایی ربات ها از طریق تحلیل و پردازش لاگ دسترسی وب سرور و استفاده از روش های داده کاوی، انجام می شود. نتایج تجربی نشان می دهد که روش پیشنهادی در این پژوهش با کشف ویژگی های جدید و معرفی شرط جدید در برچسب گذاری نشست ها، باعث بهبود دقت در شناسایی ربات ها و در نتیجه ایجاد بهبود در رتبه بندی ترافیکی تارنماها نسبت به کارهای پیشین شده است.
کلیدواژگان: رتبه بندی ترافیکی، شناسایی ربات، برچسب گذاری نشست، لاگ دسترسی وب سرور، داده کاوی -
صفحات 81-88
هر فرد حدود یک سوم عمر خود را در حالت خواب می گذراند. نکته جالب این است که مغز یک فرد خوابیده به هیچ عنوان در حالت غیر فعال و ساکت نیست و به خصوص در شبکه عصبی هیپوکمپ امواج تیز ریپل مشاهده می شوند. در اینجا یک مدل پدیده شناختی که در آن تطبیق پذیزی برای نورون های تحریکی در نظر گرفته شده است، برای شبکه CA1-CA3 هیپوکمپ ارایه می دهیم. این مدل ساده در غیاب محرک خارجی نوساناتی با خواص مشابه امواج تیز ریپل که در تجربه به دست آمده است، تولید می کند؛ به خصوص نشان می دهیم در اثر کاهش تحریک در شبکه، دامنه ریپل ها افزایش می یابد و فرکانس ریپل ها کم می شود؛ به علاوه احتمال تشکیل دوتایی های ریپل در اثر کاهش تحریک افزایش می یابد. این نتایج با نتایج تجربی هم خوانی بسیار خوبی دارد.
کلیدواژگان: مدل جرم نورونی، امواج تیز ریپل، spike frequency adaptation -
صفحات 89-124
سامانه های پیشنهادگر در زمینه تجارت الکترونیک شناخته شده هستند. از این گونه سیستم ها انتظار می رود که کالاها و اقلام مهمی (از جمله موسیقی و فیلم) را به مشتریان پیشنهاد دهند. در سامانه های پیشنهادگر سنتی از جمله روش های پالایش محتوا پایه و پالایش مشارکتی، چالش ها و مشکلات مهمی از جمله شروع سرد، مقیاس پذیری و پراکندگی داده ها وجود دارد. اخیرا به کارگیری روش های ترکیبی توانسته با بهره گیری از مزایای این روش ها با هم، برخی از این چالش ها را تا حد قابل قبولی حل نمایند. در این مقاله سعی می شود روشی برای پیشنهاد ارایه شود که ترکیبی از دو روش پالایش محتوا پایه و پالایش مشارکتی (شامل دو رویکرد حافظه پایه و مدل پایه) باشد. روش پالایش مشارکتی حافظه پایه، دقت بالایی دارد، اما از مقیاس پذیری کمی برخوردار است. در مقابل، رویکرد مدل پایه دارای دقت کمی در ارایه پیشنهاد به کاربران بوده اما مقیاس پذیری بالایی از خود نشان می دهد. در این مقاله سامانه پیشنهادگر ترکیبی مبتنی بر هستان شناسی ارایه شده که از مزایای هر دو روش بهره برده و براساس رتبه بندی های واقعی، مورد ارزیابی قرار می گیرد. هستان شناسی، توصیفی واضح و رسمی برای تعریف یک پایگاه دانش شامل مفاهیم (کلاس ها) در حوزه موضوعی، نقش ها (رابط ها) بین نمونه های مفاهیم، محدودیت های مربوط به رابطه ها، همراه با یک مجموعه از عناصر و اعضا (یا نمونه ها) است که یک پایگاه دانش را تعریف می کند. هستان شناسی در بخش پالایش محتوا پایه مورد استفاده قرار می گیرد و ساختار هستان شناسی توسط تکنیک های پالایش مشارکتی بهبود می یابد. در روش ارایه شده در این پژوهش، عملکرد سیستم پیشنهادی بهتر از عملکرد پالایش محتوا پایه و مشارکتی است. روش پیشنهادی با استفاده از یک مجموعه داده واقعی ارزیابی شده است و نتایج آزمایش ها نشان می دهد روش مذکور کارایی بهتری دارد. همچنین با توجه به راه کارهای ارایه شده در مقاله حاضر، مشخص شد، روش پیشنهادی دقت و مقیاس پذیری مناسبی نسبت به سامانه های پیشنهادگری دارد که صرفا حافظه پایه (KNN) و یا مدل پایه هستند.
کلیدواژگان: سامانه پیشنهادگر، هستان شناسی، پالایش حافظه پایه، پالایش مدل پایه، خوشه بندی، KNN -
صفحات 125-152
پیش بینی قیمت سهام در بورس اوراق بهادار از جمله چالش برانگیزترین مباحث در مقوله پیش بینی است که توجهات بسیاری از جمله محققان را به خود جلب کرده است. عوامل مختلف درگیر در بورس اوراق بهادار سبب شده است تا بازار بورس همیشه از خود فرآیندی پویا و پیچیده داشته باشند. لذا پژوهش گران بر آن شده اند تا در پیش بینی رفتار بورس، به دنبال روش های نوینی باشند که دربرابر عدم ایستایی و پیچیده بودن مقاوم باشند. در این پژوهش یک مدل ترکیبی دوگانه متشکل از دو سامانه استنتاج فازی و یک الگوریتم رقابت استعماری به صورت ترکیبی استفاده شده است که یک سامانه فازی برای ایجاد مدلی برای پیش بینی قیمت سهام براساس 10 متغیر تاثیرگذار بر قیمت سهام استفاده می شود که قوانین فازی موتور استنتاج این سامانه فازی توسط نسخه بهبود یافته فازی جدید الگوریتم رقابت استعماری به دست می آید و پارامترهای الگوریتم رقابت استعماری نیز توسط یک سامانه فازی دیگر به نام تنظیم کننده پارامترها ، تعیین می شوند. به منظور ارزیابی عملکرد مدل پیشنهادی اطلاعات مرتبط با قیمت سهام شش شرکت فعال در بورس اوراق بهادار تهران در نظر گرفته شده و هشت مدل پیش بینی قیمت سهام در دو گروه الگوریتم به همراه مدل پیشنهادی پیاده سازی شدند. نتایج به دست آمده نشان از عملکرد بهتر مدل پیشنهادی از جهت کیفیت نتایج پیش بینی شده و انحراف کم نتایج فاز آزمون از فاز آموزش دارد.
کلیدواژگان: پیش بینی قیمت سهام، سامانه استنتاج فازی ممدانی، شبک عصبی، درخت تصمیم، جنگل تصادفی، ماشین بردار پشتیبان، الگوریتم رقابت استعماری -
صفحات 153-164
جریان داده به دنباله ای از داده ها گفته می شود که از منابع اطلاعاتی مختلف با سرعت زیاد و حجم بالا تولید می شوند. از مهم ترین چالش های موجود در تحلیل جریان داده وجود تغییر مفهوم در آن ها است. تغییر مفهوم به معنای تغییر ویژگی های آماری داده هاست. در بسیاری از پژوهش های موجود برای مقابله با چالش نامحدود بودن طول جریان داده و یا چالش تغییر مفهوم، از رویکردهایی با فرض موجود بودن برچسب درست برای همه داده ها استفاده می کنند؛ در حالی که با توجه به هزینه بر بودن فرآیند برچسب دهی جریان داده، به طورعمومی فرض می شود تنها بخشی از داده ها دارای برچسب هستند. در این مقاله یک روش یادگیری گروهی نیمه نظارتی ارایه شده که از تغییر آنتروپی برای تشخیص تغییر مفاهیم در رده بندی جریان داده استفاده می کند. مدل یادگیری گروهی پیشنهادی با تعداد محدودی داده برچسب دار اولیه آموزش می بیند؛ سپس در صورت مشاهده تغییر مفهوم، از داده های بدون برچسب برای به روزرسانی مدل رده بند گروهی استفاده می کند. روش پیشنهادی قادر است تغییرات موجود در مجموعه داده را تشخیص داده و با به روزرسانی مدل یادگیری، در بهبود دقت الگوریتم موثر باشد. نتایج آزمایش ها نشان می دهد که روش پیشنهادی از جنبه های مختلف نسبت به سایر روش ها کارایی بالاتری دارد.
کلیدواژگان: جریان داده، یادگیری گروهی، تغییر مفهوم، آنتروپی، رده بند نیمه نظارتی -
صفحات 165-179
نخستین گام در طبقه بندی تصاویر بافتی، توصیف بافت با استفاده از استخراج ویژگی های تصویری مختلف از آن است. تاکنون روش های متعددی برای این موضوع توسعه یافته اند که از جمله مشهورترین آن ها می توان به روش الگوی دودویی محلی اشاره کرد. به منظور استخراج اطلاعات بافتی در مقیاس های مختلف، روش الگوی باینری محلی را می توان در یک چهارچوب چندمقیاسه پیاده سازی کرد. در این حالت، بردارهای ویژگی به دست آمده در سطوح مقیاس مختلف به یکدیگر پیوست می شوند تا یک بردار ویژگی برآیند با طول بیشتر را تولید کند؛ اما چنین روشی دو عیب مهم دارد؛ نخست این که، روش الگوی دودویی محلی به شدت نسبت به نوفه حساس و با افزودن نوفه به تصویر بافتی، بردارهای ویژگی به دست آمده ممکن است به شدت تغییر کنند. دوم این که، با افزایش تعداد مقیاس ها، طول بردار ویژگی به دست آمده نیز افزایش می یابد که این امر ضمن کاهش سرعت فرآیند طبقه بندی بافت، ممکن است دقت طبقه بندی را نیز کاهش دهد. برای رفع و یا کاهش این دو عیب، در این مقاله، روشی مبتنی بر الگوی دودویی محلی چندمقیاسه پیشنهاد می شود که از مقاومت بهتری در مقابل نوفه سفید گوسی برخوردار و در عین حال، طول بردار ویژگی تولیدی به وسیله آن به طوردقیق برابر با طول بردار ویژگی تولیدی به وسیله روش اصلی الگوی دودویی محلی در حالت تک مقیاسه است. آزمایش ها بر روی چهار گروه از پایگاه داده Outex انجام شده که آزمایش های انجام گرفته نشان دهنده برتری روش پیشنهادی نسبت به روش های موجود مشابه است.
کلیدواژگان: استخراج ویژگی، الگوی دودویی محلی، بافت، طبقه بندی بافت، نوفه سفید گوسی
-
Pages 3-22
Accuracy and validity of data are prerequisites of appropriate operations of any software system. Always there is possibility of occurring errors in data due to human and system faults. One of these errors is existence of duplicate records in data sources. Duplicate records refer to the same real world entity. There must be one of them in a data source, but for some reasons like aggregation of data sources and human faults in data entry, it is possible to appear several copies of an entity in a data source. This problem leads to error occurrence in operations or output results of a system; also, it costs a lot for related organization or business. Therefore, data cleaning process especially duplicate record detection, became one of the most important area of computer science in recent years. Many solutions presented for detecting duplicates in different situations, but they almost are all time-consuming. Also, the volume of data is growing up every day. hence, previous methods don’t have enough performance anymore. Incorrect detection of two different records as duplicates, is another problem that recent works are being faced. This becomes important because duplicates will usually be deleted and some correct data will be lost. So it seems that presenting new methods is necessary. In this paper, a method has been proposed that reduces required volume of process using hierarchical clustering with appropriate features. In this method, similarity between records has been estimated in several levels. In each level, a different feature has been used for estimating similarity between records. As a result, clusters that contain very similar records will be created in the last level. The comparisons are done on these records for detecting duplicates. Also, in this paper, a relative similarity function has been proposed for comparing between records. This function has high precision in determining the similarity. Eventually, the evaluation results show that the proposed method detects 90% of duplicate records with 97% accuracy in less time and results have improved.
Keywords: Duplicate Record Detection, Data Cleaning, Hierarchical Clustering, Similarity Function, Feature Selection -
Pages 23-35
In the past decades, vehicle routing problem (VRP) has gained considerable attention for its applications in industry, military, and transportation applications. Vehicle routing problem with simultaneous pickup and delivery is an extension of the VRP. This problem is an NP-hard problem; hence finding the best solution for this problem which is using exact method, take inappropriate time, and these methods are not useful in real-world applications. Using meta-heuristic algorithms for calculating and computing the solutions for NP-hard problems is a common method to contrast this challenge. The objective function defined for this problem, is a constrained objective function. In previous algorithms, the penalty method was used as constraint handling technique to define the objective function. Determining the value of parameters and penalty coefficient is not easy in these methods. Moreover, the optimal number of vehicles was not considered in the previous algorithms. So, the user should guess number of vehicles and compare the result with other values for this variable. In this paper, a novel objective function is defined to solve the vehicle routing problem with simultaneous pickup and delivery. This method can find the vehicle routes such that increases the performance of the vehicles and decreases the processes’ costs of transportation. in addition, the optimal number of vehicle in this problem can be calculated using this objective function. Finding the best solution for this optimization problems is an NP-hard and meta-heuristic methods can be used to estimate good solutions for this problem. Then, a constrained version of gravitational search algorithm is proposed. In this method, a fuzzy logic controller is used to calculate the value of the parameters and control the abilities of the algorithm, automatically. Using this controller can balance the exploration and exploitation abilities in the gravitational search algorithm and improve the performance of the algorithm. This new version of gravitational search algorithm is used to find a good solution for the predefined objective function. The proposed method is evaluated on some standard benchmark test functions and problems. The experimental results show that the proposed method outperforms the state-of-the-art methods, despite the simplicity of implementation.
Keywords: Vehicle Routing Problem, Meta-heuristic algorithms, Constrained Gravitational Search Algorithm -
Pages 37-47
Clustering techniques are used to extract the structure of software for understanding, maintaining, and refactoring. In the literature, most of the proposed approaches for software clustering are divided into hierarchical algorithms and search-based techniques. In the former, clustering is a process of merging (splitting) similar (non-similar) clusters. These techniques suffered from the drawbacks such as finiteness criterion and arbitrary decisions occurred in the process. Because of the NP-hardness of clustering software systems, evolutionary and search-based algorithms are more commonly used algorithm than hierarchical ones. In evolutionary algorithms, the clustering of software systems is considered as a problem of searching over some possible clustering candidates. Although these algorithms are often able to achieve an appropriate structure of the software, they are not applicable in clustering large-scale software. Furthermore, these algorithms are unable to consider the knowledge in the artifact dependency graph, which extracted from the source code of the software. In software systems, an artifact can be everything like a class, a function, or a file. In this paper, a new partition-based clustering algorithm is presented. This algorithm attempts to partition the artifact dependency graph considering the knowledge therein. Moreover, a new distance criterion is presented to measure the similarity and dissimilarity of the artifacts. The proposed algorithm starts with the artifact dependency graph and creates the similarity matrices of the artifacts. So, it attempts to refine the partition candidate until a fixed point is reached. We expect that the proposed method compared with other methods could lead to achieve the clustering with high quality and similar to the expert's clustering based on MoJo-FM measure. To demonstrate the applicability and validity of the proposed algorithm, a large-scale case study, Mozilla Firefox, is employed. The results demonstrate that the proposed algorithm outperforms the commonly used evolutionary methods in the literature.
Keywords: Software Engineering, Reverse Engineering, Software Clustering, K-means algorithm -
Pages 49-68
By increasing the number of images, it is essential to provide fast search methods and intelligent filtering of images. To handle images in large datasets, some relevant tags are assigned to each image to for describing its content. Automatic Image Annotation (AIA) aims to automatically assign a group of keywords to an image based on visual content of the image. AIA frameworks have two main stages; Feature Extraction and Tag Assignment which are both important in order to reach a proper performance. In the first stage of our proposed method, we utilize deep models to obtain a visual representation of images. We apply different pre-trained architectures of Convolutional Neural Networks (CNN) to the input image including Vgg16, Dense169, and ResNet 101. After passing the image through the layers of CNN, we obtain a single feature vector from the layer before the last layer, resulting into a rich representation for the visual content of the image. One advantage of deep feature extractor is that it substitutes a single feature vector instead of multiple feature vectors and thus, there is no need for combining multiple features. In the second stage, some tags are assigned from training images to a test image which is called “Tag Assignment”. Our approach for image annotation belongs to the search-based methods which have high performance in spite of simple structure. Although it is even more time-consuming due to its method of comparing the test image to every training in order to find similar images. Despite the efficiency of automatic Image annotation methods, it is challenging to provide a scalable method for large-scale datasets. In this paper, to solve this challenge, we propose a novel approach to summarize training database (images and their relevant tags) into a small number of prototypes. To this end, we apply a clustering algorithm on the visual descriptors of training images to extract the visual part of prototypes. Since the number of clusters is much smaller than the number of images, a good level of summarization will be achieved using our approach. In the next step, we extract the labels of prototypes based on the labels of input images in the dataset. because of this, semantic labels are propagated from training images to the prototypes using a label propagation process on a graph. In this graph, there is one node for each input image and one node for each prototypes. This means that we have a graph with union of input images and prototypes. Then, to extract the edges of graph, the visual feature of each node on graph is coded using other nodes to obtain its K-nearest neighbors. This goal is achieved by using Locality-constraints Linear Coding algorithm. After construction the above graph, a label propagation algorithm is applied on the graph to extract the labels of prototypes. Based on this approach, we achieve a set of labeled prototypes which can be used for annotating every test image. To assign tags for an input image, we propose an adaptive thresholding method that finds the labels of a new image using a linear interpolation from the labels of learned prototypes. The proposed method can reduce the size of a training dataset to 22.6% of its original size. This issue will considerably reduce the annotation time such that, compared to the state-of-the-art search-based methods such as 2PKNN, the proposed method is at least 4.2 times faster than 2PKNN, while the performance of annotation process in terms of Precision, Recall and F1 will be maintained on different datasets.
Keywords: Database Summarization, Image Annotation, Search-Based method, Scalability -
Pages 69-80
With the expansion of the Internet and the Web, communication and information gathering between individual has distracted from its traditional form and into web sites. The World Wide Web also offers a great opportunity for businesses to improve their relationship with the client and expand their marketplace in online world. Businesses use a criterion called traffic ranking to determine their site's popularity and visibility. Traffic ranking measures the amount of visitors to a site and based on these statistics, allocates a ranking to the site. One of the most important challenges in the ranking is the creation of fake traffic that generated by applications called robots. Robots are malicious software components that used to generate spam, set up distributed denial of services attacks, fishing, identity theft, removal of information and other illegal activities .there are already several ways to identify and discover the robot. According to Doran et al., The identification methods are divided into two categories: offline and real-time. The offline detection method is divided into three categories: Syntactical Log Analysis, Traffic Pattern Analysis, and Analytical Learning Techniques. The real-time method is performed by the Turing test system. In this research, the identification of robots is done through the offline method by analysis and processing of access logs to the web server and the use of data mining techniques. In this method, first, the features of each session are extracted, then generally these sessions are labeled with three conditions into two categories of human and robot. Finally, by using data mining tool, web robots are detected. In all previous studies, the features are extracted from each sessions, for example in first studies, Tan&Kumar extracted 25 features of sessions. After that Bomhardt et al. used 34 features to identify the robots. In 2009 Stassopoulou et al. used 6 features that was extracted from sessions and so on. But in this research, features are extracted from sessions of a unique user. Experimental results show that the proposed method in this research, by discovering new features and introducing a new condition in session labeling, improves the accuracy of identifying robots and moreover, improves the ranking of web traffic from previous work.
Keywords: Traffic Ranking, Robot Detection, Session Labeling, Web Server Access Log, Data Mining -
Pages 81-88
We spend one third of our life in sleep. The interesting point about the sleep is that the neurons are not quiescent during sleeping and they show synchronous oscillations at different regions. Especially sharp wave ripples are observed in the hippocampus. Here, we propose a simple phenomenological neural mass model for the CA1-CA3 network of the hippocampus considering the spike frequency adaptation for excitatory neurons. The model consists of one group of identical CA1 excitatory neurons, one group of identical CA1 inhibitory neurons, one group of identical CA3 excitatory neurons, and one group of identical CA3 inhibitory neurons. All the recurrent connections between the neurons of CA3 network are considered. For CA1 neurons the excitatory to inhibitory, inhibitory to excitatory and inhibitory to inhibitory connections are considered. CA1 and CA3 neurons are connected by long-range connections from CA3 excitatory neurons to both CA1 excitatory and inhibitory neurons. We show that this simple model can spontaneously generate the oscillations similar to the sharp waves in the CA3 network. The duration of the sharp waves is determined by the slow dynamic of the adaptation process. The excitatory inputs from CA3 network to the CA1 network during these sharp waves induce ripples in the CA1 network due to the interaction of excitatory and inhibitory neurons. We next show that contrary to intuition and in a very good agreement with the recent experimental findings, reduction of the excitation increases the amplitude of the ripples while decreases the frequency of them. This model can also spontaneously generate ripple doublets. The decrease in the excitation is associated with the increase in the probability of observing ripple doublets. Our results shed light on our understanding of the mechanism underlying the generation of sharp wave ripples.
Keywords: Neural mass model, Sharp wave ripples, Spike frequency adaptation -
Pages 89-124
The recommender systems are models that are to predict the potential interests of users among a number of items. These systems are widespread and they have many applications in real-world. These systems are generally based on one of two structural types: collaborative filtering and content filtering. There are some systems which are based on both of them. These systems are named hybrid recommender systems. Recently, many researchers have proved that using content models along with these systems can improve the efficacy of hybrid recommender systems. In this paper, we propose to use a new hybrid recommender system where we use a WordNet to improve its performance. This WordNet is also automatically generated and improved during its generation. Our ontology creates a knowledge base of concepts and their relations. This WordNet is used in the content collaborator section in our hybrid recommender system. We improve our ontological structure via a content filtering technique. Our method also benefits from a clustering task in its collaborative section. Indeed, we use a passive clustering task to improve the time complexity of our hybrid recommender system. Although this is a hybrid method, it consists of two separate sections. These two sections work together during learning. Our hybrid recommender system incorporates a basic memory-based approach and a basic model-based approach in such a way that it is as accurate as a memory-based approach and as scalable as a model-based approach. Our hybrid recommender system is assessed by a well-known data set. The empirical results indicate that our hybrid recommender system is superior to the state of the art methods. Also, our hybrid recommender system is more accurate and scalable compared to the recommender systems, which are simply memory-based (KNN) or basic model-based. The empirical results also confirm that our hybrid recommender system is superior to the state of the art methods in terms of the consumed time. While this method is more accurate than model-based methods, it is also faster than memory-based methods. However, this method is not much weaker in terms of accuracy than memory-based methods, and not much weaker in terms of speed than model-based methods.
Keywords: Recommender System, Ontology, Memory-based Filtering, Model-based Filtering, Clustering, KNN -
Pages 125-152
Investing on the stock exchange, as one of the financial resources, has always been a favorite among many investors. Today, one of the areas, where the prediction is its particular importance issue, is financial area, especially stock exchanges. The main objective of the markets is the future trend prices prediction in order to adopt a suitable strategy for buying or selling. In general, an investor should be predicted the future status of the time, the amount and location of his assets in a way that increases the return on his assets. Stock price prediction is one of the most challenging topics in the field of forecasting, which has attracted many attentions from researchers. The various factors of the markets have caused the situation that they always have a dynamic and complex process. Therefore, researchers have been determined to look for new prediction methods of stock price, which will reduce the instability and complexity of the markets. In fact, the most of recent studies have shown that the stock market is a nonlinear, dynamic, and non-parametric system that is affected by various economic factors. The applications of artificial intelligence and machine learning techniques to identify the relationship between the factors and stock price exchanges can be organized in seven major groups such as neural networks and deep learning, support vector machine, decision tree and random forest, k nearest neighbor, regression, Bayesian networks and fuzzy inference-base methods. Due to the mentioned prediction methods have their own challenges, hydridizations of the meta-heuristic algorithms and the methods were applied to stock price prediction. In this paper, a new hybridization of Fuzzy Inference System and a novel modified Fuzzy Imperialist Competitive Algorithm (FICA+FIS) are proposed to stock price prediction. To achieve this aim, two Fuzzy Inference Systems are designed to tuing the ICA’s parameters based on three effective factors in search strategy and to predict stock price based on 10 effective economic factors. The candidate fuzzy rules set of the inference engine is obtained by the FICA for the second FIS and six fuzzy rules of the first FIS are designed based on the ICA’s behaviour. The FICA+FIS has 10 inputs of the stock price variables including the lowest stock price, the highest stock price, the initial stock price, the trading volume, the trading value, the first market index of the trading floor, the total market price index, the dollar exchange rate, the global price per ounce of gold, the global oil price, and its output is also the stock price. The inputs and output variables consist of three linguistic vairables such as Low, Medium, and High with triangular membership functions. Each country (search agent) of the FICA contains information on all the fuzzy rules of the inference engine attributed to the country and has r×12 elements, where r is the number of fuzzy rules. The FICA’s objective function is the mean square error (MSE) to evaluate the power of each country. A challenge of the ICA is the proper tuning paprameters such as the Revolution Probability (Prevolve), Assimilation Coefficient (Beta) and the Colonies Mean Cost Coefficient (zeta), which has a great impact on the efficiency of the algorithm (precision and time of access to solution). These parameters are usually constant and according to different problems, they have different values and are given experimentally. In this paper, the parameters are tuned based on the number of iterations that the best objective function value has not improved (UN), the number of imperialist (Ni) and the current number iteration (Iter). To this aim, a FIS is designed based on six fuzzy rules that UN, Ni and Iter are its input variables and Prevolve, Beta and zeta are its output variables. To analyze the efficiency of the FICA+FIS as a case study, six datasets are collocted from six companies which were active between 1389 to 1394 in Tehran Stock Exchange such as Pars Oil, Iran Khodro, Motogen, Ghadir, Tidewater and Mobarakeh. The information of around 2000 days are collected for each company and the data are divided to train and test data based on cross validation 10-fold. To compare the performance of the FICA+FIS, two groups of stock price prediction methods were implemented. In the first group, the fuzzy rules of the FIS’s engine to stock price prediction are obtained by the classic draft of the Imperialist Competitive Algorithm (ICA+FIS), the Genetic Algorithm (GA+FIS) and the Whale Optimization Algorithm (WOA+FIS), which are used to compare with the FICA. The second group includes classic stock price prediction methods such as multi-layered neural network (NN), support vector machine (SVM), CART decision tree (DT-CART), random forest (RF) and Gaussian process regression (GPR), which are used to compare with the FICA+FIS. The experimental results show that first, the improved fuzzy draft of the ICA performed better than its classic draft, the GA and the WOA, and second, the performance of the FICA FIS is better than other investigated algorithms in both training and testing phases, although the DT is a competitor in the training phase and the RF is a competitor in the test phase on some datasets.
Keywords: Stock Price Prediction, Fuzzy Inference Systems, Neural Networks, Decision Tree, Random Forest, Support Vector Machine, Imperialist Competitive Algorithm -
Pages 153-164
Data stream is a sequence of data generated from various information sources at a high speed and high volume. Classifying data streams faces the three challenges of unlimited length, online processing, and concept drift. In related research, to meet the challenge of unlimited stream length, commonly the stream is divided into fixed size windows or gradual forgetting is used. Concept drift refers to changes in the statistical properties of data, and is divided into four categories: sudden, gradual, incremental, and recurring. Concept drift is generally dealt with by periodically updating the classifier, or employing an explicit change detector to determine the update time. These approaches are based on the assumption that the true labels are available for all data samples. Nevertheless, due to the cost of labeling instances, access to a partial labeling is more realistic. In a number of studies that have used semi-supervisory learning, the labels are received from the user to update the models in form of active learning. The purpose of this study is to classify samples in an unlimited data stream in presence of concept drift, using only a limited set of initial labeled data. To this end, a semi-supervised ensemble learning algorithm for data stream is proposed, which uses entropy variation to detect concept drift and is applicable for sudden and gradual drifts. The proposed model is trained with a limited initial labeled set. In occurrence of concept drift, the unlabeled data is used to update the ensemble model. It does not require receiving the labels from the user. In contrast to many of the current studies, the proposed algorithm uses an ensemble of K-NN classifiers. It constructs a group of clustering-based classification models, each of which is trained on a batch of data. On receiving each new sample, first it is determined whether the data sample is an outlier or not. If the data is included in a cluster, the sample class is determined by majority voting. When a window of the stream is received, the possibility of concept drift is examined based on entropy variation, and the classifier is updated by a semi-supervised approach if necessary. The model itself determines the required data labels. The proposed method is capable of detecting concept drift in data, and improving its accuracy via updating the learning model with appropriate samples received from the stream. Therefore, the proposed method only requires a small initial labeled data. Experiments are performed using five real and synthetic datasets, and the model performance is compared to three other approaches. The results show that the proposed method is superior in terms of precision, recall and F1 score compared to other studies.
Keywords: data stream, ensemble learning, concept drift, entropy, semi-supervised classification -
Pages 165-179
In this paper we describe a novel noise-robust texture classification method using joint multiscale local binary pattern. The first step in texture classification is to describe the texture by extracting different features. So far, several methods have been developed for this topic, one of the most popular ones is Local Binary Pattern (LBP) method and its variants such as Completed Local Binary Pattern, Extended Local Binary Pattern, Local Temporary Pattern, Local Contrast Pattern, etc. In order to extract the features of a texture in different scales, the LBP method can be implemented in a multi-scale framework. For this purpose, the extracted feature vectors at different scales are usually concatenated together to produce the final feature vector with a longer length. But such a scheme has two main shortcomings. First, the LBP method is very sensitive to noise, hence by adding noise to a texture image, its feature vectors may change significantly. Second, by increasing the number of the scales, the length of the final feature vector is increased accordingly. This action increases the classification process time, and it may reduce the classification accuracy. To mitigate these shortcomings, this paper presents a method based on multiscale LBP, which has a better resistance against white Gaussian noise, while the length of its final feature vector is equal to the length of the final feature vector produced by the original LBP method. To implement the proposed method, we used 17 circular binary masks that contain 8 directed first-order masks, 8 directed second-order masks and 1 undirected mask. These masks have positive and negative weightes and each group of these masks have different radius which after convolution with input image extract features in different scales. Experiments were performed on four test groups of Outex database. Experimental results show that the proposed method is superior to the existing state-of-the-art methods. The complexity of proposed method is also analyzed. The results show that in this method, despite obtaining excellent classification accuracy, the complexity of the method has not changed much and even its complexity is less than some of the existing state-of-the-art methods.
Keywords: feature extraction, Local Binary Pattern, texture, texture classification, white gaussian noise