فهرست مطالب
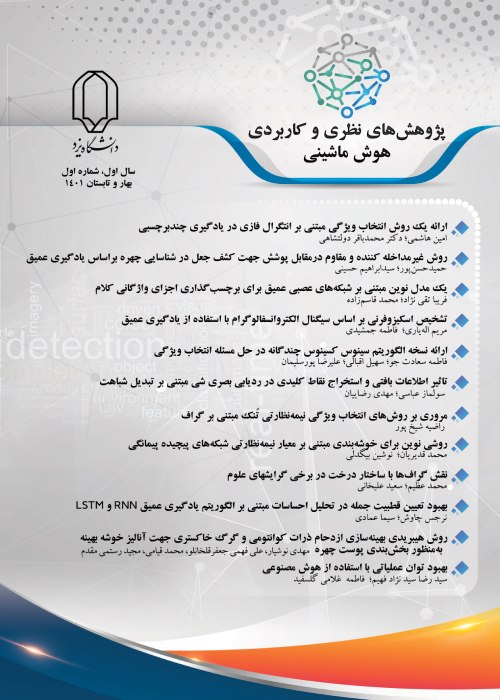
نشریه پژوهش های نظری و کاربردی هوش ماشینی
پیاپی 2 (پاییز و زمستان 1402)
- تاریخ انتشار: 1402/09/01
- تعداد عناوین: 12
-
صفحات 1-15
با رشد بسیار سریع سیستم های نظارت ویدیویی برای نظارت بر رفتارهای انسانی، تقاضا برای چنین سیستم هایی که قادر به تشخیص رخدادهای خشونت آمیز به صورت خودکار باشند در حال افزایش می باشد. تشخیص خشونت یکی از حوزه های تحقیقاتی فعال در یادگیری ماشین و پردازش تصویر برای جذب محققان جدید است. در واقع، روش های تشخیص خشونت به دو دسته عمده تقسیم می شوند که عبارتند از: روش های یادگیری ماشینی سنتی و روش های یادگیری عمیق. در این مقاله، روش های یادگیری عمیق ارایه می شوند و گوناگونی روش ها و ساختارهای شبکه های عصبی عمیق در این خصوص بررسی می شوند. درابتدا روش های سنتی و عمیق با یکدیگر مقایسه می شوند و برتری روش های عمیق بر روش های سنتی از جنبه های مختلف مورد بررسی قرار می گیرد. سپس ساختارهای مختلف شبکه های عمیق در خصوص تشخیص خشونت ارایه می گردد. علاوه بر این، مجموعه داده های موجود برای تحلیل خشونت در ویدیو ارایه می شوند. در نهایت، بحث در مورد تحقیقات انجام شده بیان می شود که می تواند برای گسترش کارهای آینده مفید باشند
کلیدواژگان: تشخیص خشونت، یادگیری عمیق، تشخیص رفتار خشونت آمیز، سیستم های نظارتی، یادگیری ماشین -
صفحات 16-28روش های انتخاب ویژگی ابزاری کارا در بهبود فرآیند یادگیری شناخته می شوند. هدف از یک روش انتخاب ویژگی، شناسایی ویژگی های مرتبط و حذف ویژگی های غیرمرتبط به منظور بدست آوردن یک زیرمجموعه مناسب از ویژگی ها است، بطوریکه افزونگی بین ویژگی های انتخاب شده کمینه گردد. در داده های چند-برچسبه، این امکان وجود دارد که در صورت وجود همبستگی بین ویژگی ها، مقدار افزونگی در مجموعه ویژگی ها افزایش یابد. وجود افزونگی بین ویژگی ها به همراه چالش ابعاد بالای داده های چند-برچسبه، می تواند باعث افزایش حجم محاسبات، کاهش دقت و در نهایت افزایش احتمال رخ دادن خطا در پیش بینی و طبقه بندی داده های چند-برچسبه شود. در این مقاله، با هدف کمینه کردن افزونگی ویژگی های انتخابی، یک الگوریتم انتخاب ویژگی چند-برچسبه با در نظر گرفتن مدل رگرسیون کمترین مربعات خطا و تنظیم تنکی پیشنهاد شده است. در انتها، با استفاده از تعدادی مجموعه داده چند-برچسبه مشهور، کارایی روش پیشنهادی بررسی می گردد و نتایج بدست آمده با چند روش انتخاب ویژگی چند-برچسبه متداول مقایسه می شودکلیدواژگان: انتخاب ویژگی چند-برچسبه، کمینه کردن افزونگی، رگرسیون، کمترین مربعات خطا، تنظیم تنکی
-
تلفیق روش های یادگیری شات محدود جهت بهبود عملکرد طبقه بندی تصاویر با مجموعه داده های کمصفحات 29-43در این پژوهش سعی بر آن شد تا شکل تغییریافته ای از رویکرد prototypical networks برای حل مسیله طبقه بندی شات محدود پیشنهاد شود. در این شبکه ها، طبقه بند سعی می کند تا با توجه به تنها تعداد کمی از نمونه های هر کلاس جدید نسبت به این کلاس ها تعمیم یابد. در رویکرد پیشنهادی به جای فاصله اقلیدسی، که در رویکرد مرجع استفاده شده است، از فاصله ماهالانوبیس برای اندازه گیری فاصله بین نمونه ها استفاده شد. به این ترتیب شبکه یک فضای متریک را یاد می گیرد که در آن می توان طبقه بندی را با محاسبه فواصل نسبت به بازنمایی های نمونه اولیه هر کلاس انجام داد. همچنین از یک معماری شبکه عصبی پنج لایه با فیلتر هایی با سایز پنج در پنج به جای بلوک های چهارتایی معرفی شده در رویکرد prototypical networks مرجع استفاده شد. این تغییرات موجب بهبود عملکرد این شبکه ها در طبقه بندی تصاویر omniglot و miniImageNet شد به طوری که شبکه پیشنهادی توانست به ترتیب به دقت های 1/99% و 5/68% بر روی این دو مجموعه داده دست یابد که نسبت به شبکه های نمونه های اولیه از دقت بهتری برخوردار است. نتایج نشان می دهد که برخی از تصمیمات ساده طراحی می توانند پیشرفت های قابل توجهی را در رویکردهای اخیر در این زمینه مانند انتخاب های معماری پیچیده و فرا یادگیری ایجاد کنند.کلیدواژگان: طبقه بندی، یادگیری شات محدود، فرا یادگیری، یادگیری متریک
-
صفحات 44-53
محبوبیت روزافزون اینترنت منجر به رشد چشمگیر تجارت الکترونیک شد. با این حال، چنین فعالیت هایی دارای چالش های امنیتی اساسی است که عمدتا ناشی از کلاهبرداری های سایبری و سرقت هویت افراد است. از این رو، بررسی مشروعیت صفحات وب بازدید شده یک کار بسیار مهم برای ایمن کردن هویت مشتریان و جلوگیری از حملات فیشینگ است. استفاده از روش های یادگیری ماشین، و یادگیری عمیق به طور گسترده به عنوان یک راه حل امیدوارکننده شناخته شده است. تحقیقات، مملو از مطالعاتی است که از روش های یادگیری ماشین و یادگیری عمیق برای تشخیص فیشینگ وب سایت استفاده می کنند. با توجه به این که در تحقیقات قبلی، تشخیص سایت-های فیشینگ با استفاده از ویژگی های مختلف توسط شبکه های عمیق بررسی شده است، در تحقیق جاری، ما قصد داریم تشخیص سایت های فیشینگ را با استفاده از چندین نوع ویژگی به صورت ترکیبی با کمک شبکه های عمیق انجام دهیم. بنابراین در این مقاله ابتدا ما به مرور روش های شناسایی سایت های فیشینگ از سایت های قانونی می پردازیم و در انتها روش پیشنهادی خود را ارایه می دهیم.
کلیدواژگان: شناسایی سایت های فیشینگ، یادگیری ماشین، یادگیری عمیق -
صفحات 54-66
پیام رسان تلگرام بستری مناسب برای کاربرانی است که به دنبال خرید محصول یا دریافت خدمات به صورت آنلاین هستند. در این پیام رسان، برای درخواست محصول،امکان دسترسی مستقیم به ارایه دهندگان کالا و خدمات وجود ندارد و باید ابتدا در گروه های تلگرامی مرتبط عضو شد و درخواست خود را در تک تک گروه ها ثبت کرد و منتظر پاسخ ماند. این امر ضمن زمان بر بودن، با مشکلاتی همراه است. هدف از این پژوهش تشخیص کاربران ارایه دهنده خدمات به منظور ارتباط مستقیم و موثر با مشتریان است. بدین منظور از ایده دسته بندی پیام های فارسی منتشر شده در تلگرام استفاده شد. یکی از مشکلات دسته بندی این پیام ها، ابعاد بزرگ فضای ویژگی است که سبب کاهش دقت و افزایش زمان دسته بندی می شود. برای حل این مسیله از روش های انتخاب ویژگی استفاده شد. روش پیشنهادی این پژوهش، بر مبنای ترکیب روش های انتخاب ویژگی مبتنی بر فیلتر محلی و سراسری است. نوآوری این پژوهش در استفاده از روش های ترکیبی انتخاب ویژگی جهت دسته بندی خودکار پیام های فارسی تلگرام، به منظور شناسایی کاربران ارایه دهنده خدمات است. روش پیشنهادی، ضمن کاهش تعداد ویژگی ها و انتخاب ویژگی های مرتبط، سبب بهبود عملکرد دسته بندی و تشخیص کاربران ارایه دهنده خدمات می شود.
کلیدواژگان: دسته بندی، کاربران ارائه دهنده خدمات، انتخاب ویژگی، کاهش ویژگی، یادگیری ماشین -
صفحات 67-80هنگامی که یک فرد تحت تاثیر یک محرک هیجانی قرار می گیرد، حالت هیجانی او تغییر می کند. این تغییرات در زمان گفتگو به خوبی در صدا و لحن گفتار نمود پیدا می کنند. این محتوای هیجانی در قالب مولفه های زبان شناختی از طریق پیام های گفتاری به افراد منتقل می شود. در سوی دیگر، شنونده ی سخن قادر است تا این محتوای هیجانی پیام دریافت شده را بازشناسی کند و همچنین رفتار هیجانی همبسته با آن را پیش بینی کند. سیستم عصبی و شناختی انسان قادر است این فرآیند را با وجود تمامی پیچیدگی ها به راحتی انجام دهد. به عبارت دیگر، محتوای هیجانی موجود در گفتار را بازشناسی کرده و متناسب با آن حالت هیجانی بازشناسی شده مجموعه ای از رفتارهای همبسته را برای گوینده ی گفتار، پیش بینی کند. این موضوع در مطالعات تعامل انسان و ماشین بسیار حایز اهمیت است. در اینجا، مدلی بر اساس حافظه انجمنی دو سویه ارایه شده است که قادر می باشد بر اساس حالت هیجانی، رفتار هیجانی مربوطه را پیش بینی کند. نتایج حاصل از شبیه سازی نشان می دهند، این مدل قادر است فرآیند نگاشت میان حالات هیجانی و مجموعه رفتارهای مربوطه را به صورت دو سویه انجام دهد و به صورت قدرتمندی در برابر درجاتی از ناکاملی اطلاعات و میزانی از نویز کارآمد باشد.کلیدواژگان: پیش بینی رفتار هیجانی، حافظه انجمنی دو سویه، رفتار هیجانی، حالت هیجانی، هیجان
-
صفحات 81-91
هدف این مقاله تشریح پیاده سازی دو قسمت اول از سه قسمت عمده زیر (1) منطق فازی، (2) استدلال جبری ومنطقی یکپارچه و (3) یاد گیری اتوماتیک عبارات فضایی است.پس از بحث و بررسی در خصوص لزوم استفاده از عبارات فازی در هندسه فضایی به چگونگی ادغام مختصات کمی و کیفی مرتبط با اشکال هندسی در موتور سیستم ارزیابی عبارات فضایی و اثبات قضایا، بنام Geomkr می پردازیم. استدلال یک پارچه بمعنی ادغام استدلال منطقی و جبری برای رضایتمندی عبارات توصیف صحنه یا قضایای هندسی است. بعد از توصیف نحوه ارزیابی عبارت فضایی به معرفی الگوریتم پیاده سازی می پردازیم. در خاتمه پس از نتیجه گیری به طرح مسایل جدید اشاره می کنیم. الگوریتم استدلال این سیستم نه تنها از سیستم یک پارچه روش جبری و روش منطقی مرتبه اول استفاده می کند بلکه با معرفیگزاره های فضایی فازی و نحوه ادغام آنها و همچنین گزاره های کلاسیک در این سیستم از توان بالایی نسبت به سیستم های کلاسیک برخوردار است.
کلیدواژگان: منطق فازی، ارزیابی عبارت هندسی، عبارات فازی هندسی، استدلال یکپارچه جبری و منطقی -
صفحات 92-104
این پژوهش برای افزایش دقت تخصیص اعتبار قوانین در سیستم دسته بند یادگیر با استفاده از یادگیری تقویتی مارکوف جهت پیش بینی ساختار دوم پروتیین است که یادگیری تقویتی مارکوف در سیستم دسته بند یادگیر، جایگزین الگوریتم Bucket Brigade شده است. برای آموزش سیستم از مجموعه دادگان Protein Data Bank استفاده می شود که شامل پروتیین 4L1W با تعداد نمونه 5741 است که 70 درصد برای آموزش و 30 درصد جهت آزمایش استفاده شده است. پس از آموزش سیستم، تعدادی دسته بند (قوانین) باارزش، تولید می شود که در مرحله آزمایش از این قوانین برای پیش بینی ساختار دوم پروتیین استفاده خواهد شد. نتایج آزمایش ها نشان می دهد دقت سیستم دسته بند یادگیر با یادگیری تقویتی مارکوف در نوع ساده و توسعه یافته آن، افزایش یافته است. با استفاده از یادگیری تقویتی مارکوف، ارزش گذاری به هر قانون بهبود داده می شود، به گونه ای که دقت سیستم دسته بند ساده %82.5 و سیستم دسته بند توسعه یافته %85 بهبود یافته است.
کلیدواژگان: بیوانفورماتیک، ساختار دوم پروتئین، سیستم دسته بند یادگیر، یادگیری تقویتی مارکوف -
صفحات 105-117تجزیه و تحلیل احساسات افراد از محتوای رسانه های اجتماعی از طریق متن، گفتار و تصاویر، در انواع مختلفی از برنامه ها و کاربردها مورد نیاز است. اکثر مطالعات تحقیقاتی اخیر در زمینه تجزیه و تحلیل احساسات، بر داده های متنی تمرکز داشته اند. با این حال، کاربران رسانه های اجتماعی، عکس ها و فیلم های مشابه بیشتری نسبت به متن به اشتراک می گذارند. به عبارت دیگر، تصاویر بهترین روش برای انتقال احساسات به دیگران هستند. از این رو، تمرکز بر توسعه یک مدل تحلیل احساسات بر اساس تصاویر در رسانه های اجتماعی اهمیت دارد. در این مقاله، از مدل یادگیری انتقال DenseNet-121 برای تحلیل احساسات بر اساس تصاویر استفاده خواهیم کرد. برای پیاده سازی این روش، از تصاویر موجود در مجموعه داده Image Sentiment استفاده خواهیم نمود. این مجموعه داده شامل آدرس های اینترنتی تصاویر به همراه قطبیت های احساسی آن ها است. بر اساس نتایج به دست آمده، دقت مدل پیشنهادی در این مقاله برابر با 89 % است که در مقایسه با کارهای پیشین در زمینه تجزیه و تحلیل احساسات بصری، مدل پیشنهادی، بهبود 5 تا 10 درصدی را نشان می دهد.کلیدواژگان: تجزیه و تحلیل احساسات تصویر، انتقال یادگیری، یادگیری عمیق، شبکه های اجتماعی
-
صفحات 118-130در حوزه شناخت مکاتب فلسفی، تفکر هر شخص با توجه به نوع نگرشی که در خصوص مکاتب مختلف می تواند داشته باشد متفاوت است. تشخیص نگرش نویسنده و میزان شباهت آن به هرکدام از مکاتب فلسفی همواره یکی از موضوعات مهم در حوزه علوم انسانی بوده است. در این مقاله، روشی مبتنی بر یادگیری عمیق برای تشخیص مکاتب فلسفی از روی متن پیشنهاد شده است. در روش پیشنهادی، ابتدا متن ها نرمال شده و کلمات اضافی و فاقد معنا حذف می شوند. بعد از مرحله نرمال سازی، متن به جملات و کلمات شکسته شده و سپس با استفاده از کتابخانه فست تکست هر کلمه به بردار عددی تبدیل می شوند، پس از آن با استفاده از شبکه طراحی شده ویژگی های متون استخراج شده و در نهایت سیستم، متون را یاد گرفته و آماده برای استخراج داده ها می باشد و با دادن یک جمله جدید میزان شباهت آن به هر مکتب بیان می شود. بر اساس ارزیابی صورت گرفته، میزان دقت در روش پیشنهادی 94 درصد می باشد.کلیدواژگان: مکاتب فلسفی، داده کاوی متن، تشخیص مکاتب، تبدیل متن به بردار عددی، میزان شباهت تفکر نویسنده با مکاتب
-
صفحات 131-144
ریخت شناسی بطن ها در مطالعات هیدروسفالی، اسکیزوفرنی، تومورها، تروما، بیماری آلزایمر، بیماری پارکینسون، پیری و آتروفی جهت تشخیص بیماری های عصبی مانند سکته مغزی، زوال عقل و بیماری هانتینگتون مورد بررسی قرار گرفته می شود. در این پژوهش، روشی تمام خودکار جهت بررسی مورفومتریک بطن های مغزی ارایه می شود. بخش بندی بطن های مغزی گامی مهم جهت آشکارسازی لندمارک های بطن های مغزی است. همچنین، تخمین اولیه ناحیه بطنی مغزی می تواند در بخش بندی دقیق مرز بطن های مغزی موثر باشد. برای این منظور، خوشه بند فازی (FCM) بهینه شده با الگوریتم شاهین هریس (HHO) و ویژگی های کانال تجمیعی (ACF) به کار گرفته می شوند. جهت اندازه گیری شاخص های خطی بطن های مغزی شامل ایوانز، دودمی، دودمی-قدامی، دودمی-گیجگاهی و شماره هاکمن نیازمند مکان یابی تعدادی لندمارک بر روی تصاویر MRI هستیم. این فرآیند براساس ویژگی های هندسی بطن های مغزی و به کارگیری تبدیل هاف انجام می شود. نتایج پیاده سازی نشان می دهند که الگوریتم پیشنهادی با دقت 90%، حساسیت 82% و ویژگی 99% بهترین عمل کرد را در بخش بندی بطن های مغزی نسبت به سایر روش های مقایسه شده دارد. همچنین، نتایج نشان می دهند که دقت اندازه گیری الگوریتم پیشنهادی در شاخص های ذکر شده به ترتیب 98%، 77%، 78%، 78% و 94% است.
کلیدواژگان: بهینه ساز شاهین هریس، تبدیل هاف، شاخص های بطن های مغزی، ناهنجاری های مایع مغزی-نخاعی، ویژگی های کانال تجمیعی -
صفحات 145-159
خلاصه سازی استخراجی متن یک تکنیک ضروری در پردازش زبان طبیعی است که با استخراج مهمترین جملات به تولید نسخه های فشرده از متن کمک می کند. در خلاصه سازی استخراجی جملاتی که حاوی اطلاعات مفید و مرتبط هستند برای خلاصه نهایی انتخاب می شوند. به منظور شناسایی این جملات الگوریتم های متفاوتی وجود دارند که عملکرد و خلاصه ایجاد شده از هرکدام بر اساس نوع متن و اندازه خلاصه مورد نیاز متفاوت است. در این مقاله روشی با نام Sa-TRB ارایه شده است، که برگرفته از دو الگوریتم TextRank و BERT بوده و علاوه بر استفاده از این دو روش از اشتراک جملات ایجاد شده سایر الگوریتم ها نیز بهره می برد تا دقت بالایی در انتخاب جملات خلاصه نهایی داشته باشد. مهمترین معیار برای ارزیابی عملکرد الگوریتم ها کیفیت خلاصه نهایی آنهاست، چنانکه هر چقدر خلاصه نهایی ایجاد شده توسط این الگوریتم ها به خلاصه ایجاد شده توسط انسان مشابه باشد، کیفیت خلاصه ایجاد شده بهتر است. برای به دست آوردن اندازه این تشابه از معیارهای روش ROUGE استفاده می شود. در نهایت با انجام آزمایش هایی روی دیتاست cnn-dailymail با اندازه خلاصه های مختلف نشان داده می شود که روش پیشنهادی با افزایش اندازه خلاصه مورد نیاز با وجود کاهش در معیار فراخوانی دارای دقت، امتیاز و در نتیجه کیفیت بالاتر خلاصه نهایی است، به طوری که در دو آزمایش آخر که نرخ فشردگی 20 و 25 درصد است، امتیاز روش پیشنهادی به 24.68 و 23.34 درصد رسیده است که تقریبا یک درصد از بهترین روش های آزمایش شده دیگر بهتر است.
-
Pages 1-15
With the rapid growth of video systems to monitor human behaviors, demands are increased on such systems which can detect violence events automatically. The violence detection is one of the active research area in machine learning and image processing to attract new researchers. The methods of violence detection are divided into two major categories which are traditional machine learning techniques and deep learning methods. In this article, deep learning methods have been reviewed and the variety of methods and structures of deep neural networks have been examined in this area. First, traditional and deep methods are compared with each other, and the superiority of deep methods over traditional methods is investigated from different aspects. Then, different structures of deep networks have been investigated regarding the detection of violence. Moreover, the available datasets for the analysis of violence in video are also introduced. Finally, it is discussed about the conducted research that can be useful for the development of future works.
Keywords: violence detection, Deep learning, violent behavior detection, surveillance systems, machine learning -
Pages 16-28Feature selection methods are known to be effective in improving the learning process. The purpose of a feature selection method is to identify relevant features and remove irrelevant features in order to obtain a suitable subset of features, so that the redundancy between the selected features is minimized. In multi-label data, if there is a correlation between features, it is possible that the amount of redundancy in the feature set is increased. The existence of redundancy between features along with the challenge of high dimensions of multi-label data can grow the computational calculations, decrease the accuracy and finally increase the probability of errors in the prediction and classification of multi-label data. In this article, with the aim of minimizing the redundancy of features, a multi-label feature selection algorithm is proposed considering the least squares regression model and sparse regularization. Finally, using a number of well-known multi-label data sets, the efficiency of the proposed method is verified and the results are compared with some common multi-label feature selection methods.Keywords: Multi-label feature selection, Redundancy minimization, regression, Least squared error, Sparsity regularization
-
Integration of few-shot learning methods to improve image classification performance with small data setsPages 29-43Despite the significant advancement of artificial intelligence methods in recent years, there is still a need for a lot of data to learn these methods. To meet this need, a new machine learning model called Few-shot learning has been proposed. One of the methods in this field is the prototypical networks approach, which is actually a combination of metric learning and meta-learning methods. In these networks, the classifier tries to generalize to these classes according to only a small number of samples of each new class. In this study, an attempt was made to propose a modified form of the prototype networks to solve the finite shot classification problem. Initially, in order to improve the performance of the prototype networks, instead of the Euclidean distance, the Mahalanobis distance was used to measure the distance between the samples. This improved the performance of these networks in classifying omniglot and miniImageNet images so that the proposed network was able to achieve 99.1% and 68.5% accuracy on these two datasets, respectively. The next section introduces a general approach that can automatically improve the architecture of convolutional neural networks using a genetic algorithm. In this research, this approach has been used specifically on omniglot datasets with the proposed primary architecture in prototype networks. Finally, by using this approach and replacing the proposed architecture with the main architecture of the prototype network, the network accuracy was improved and was able to achieve 99.5% accuracy.Keywords: Classification, few-shot learning, meta-learning, metric learning
-
Pages 44-53
The increasing popularity of the Internet has led to the dramatic growth of e-commerce. However, such activities have significant security challenges, mainly due to cyber fraud and identity theft. Therefore, checking the legitimacy of visited web pages is a very important task to secure the identity of customers and prevent phishing attacks. The use of machine learning methods, and deep learning is widely recognized as a promising solution. Research is full of studies that use machine learning and deep learning methods to detect website phishing. However, their findings depend on the data set and are far from generalizable. The two main reasons for the lack of generalization are impractical replication and lack of appropriate benchmark data sets for fair evaluation of systems. Furthermore, phishing methods are constantly evolving and the proposed models do not keep up with the rapid changes. In this article, we review the methods of identifying phishing sites from legal sites and finally reach the final conclusion.
Keywords: Phishing website detection, machine learning, Deep learning -
Pages 54-66
Telegram Messenger is a suitable platform for users who are looking to buy a product or receive services online. In these messengers, it is not possible to have direct access to the providers of goods and services, and in order to request the product, one must first become a member of the related groups and channels telegram. The purpose of this study is to directly identify users of service providers using the classification of Persian messages published in Telegram. One of the problems with categorizing these messages is the large size of the feature space, which reduces accuracy and increases classification time. Feature selection methods were used to solve this problem. The proposed method of this research is based on a combination of feature selection methods based on local and global filters. In this regard, in the first step, using the most widely used methods for selecting local and global filter feature, related features are selected. In the second step, a combination of local and global filtering methods is used to identify better features and increase classification accuracy. The innovation of this research is in using the combined methods of feature selection for automatic classification of Telegram Persian messages, in order to identify the users of the service provider. The proposed method, while reducing the number of features and selecting related features, improves the performance of classification and Identification of service providers.
Keywords: : classification, Service Provider Users, Feature selection, Feature Reduction, machine learning -
Pages 67-80A person's emotional state changes due to the emotional stimulus he is experiencing. During a conversation, these changes are noticeably reflected in his voice and tone of speaking as well as in the linguistic components of his speech. On the receiving side, the listener can recognize the emotional content of the received message and also, predict the emotional behavior associated with it. The human nervous and cognitive system is able to perform this process efficiently and effortlessly despite all the complexities involved in computationally defining a model for this process. In other words, it recognizes the emotional content in the speech and predict a set of related behaviors for the speaker according to that recognized emotional state. This issue is crucial in human-machine interaction studies. Here, a model based on Bidirectional Associative Memory is presented, which can predict the corresponding emotional behavior based on the emotional state. The results of the simulation show that this model is able to perform the process of mapping bidirectionally between emotional states and the set of behaviors related to emotional states and is robust against some degrees of information incompleteness and the presence of some level of noise.Keywords: Predicting Emotional Behavior, Bidirectional Associative Memory, Emotional Behavior, Emotional State, Emotion
-
Pages 81-91
This paper outlines the optimization of the two first parts of the three major components of the scene descriptions of the geometrical shapes, namely (1) fuzzy logic scheme, (2) an integrated algebraic and logical reasoning, and (3) the machine learning technique. After arguing the need for using fuzzy expressions in spatial reasoning, the integration of approximate references into spatial reasoning using absolute measurements is outlined. The integration here means that the satisfiability of a spatial expression including possibly fuzzy one is conducted by both logical and algebraic reasoning. Then, the implementation of spatial expression evaluation is briefly described. The paper ends by the conclusion and the problems to be studied. The reasoning algorithm of this system not only uses the one-piece system, algebraic method and first-order logical method, but by introducing fuzzy spatial propositions and how to integrate them, as well as classical propositions in this system, it has a high power compared to classical systems.
Keywords: Fuzzy logic, geometric expression evaluation, geometric fuzzy expressions, integrated algebraic, logical reasoning -
Pages 92-104
This research aims to enhance the accuracy of credit assignment for rules in a learning classifier system using Markov Reinforcement Learning for predicting the secondary structure of proteins. Markov Reinforcement Learning has replaced the Bucket Brigade algorithm in the learning classifier system. The Protein Data Bank dataset is utilized to train the system, specifically the protein 4L1W with 5741 samples, where 70% is used for training and 30% for testing purposes. Following the system's training, a set of valuable classifiers (rules) is generated, which will be employed in the testing phase to predict the protein's secondary structure. The experimental results demonstrate an improvement in the accuracy of the Markov Reinforcement Learning classifier system, both in the Learning classifier system and the eXtended classifier system. Through Markov Reinforcement Learning, the credit assignment to each rule is enhanced, resulting in an accuracy improvement of 82.5% for the Learning classifier system and 85% for the eXtended classifier system.
Keywords: Bioinformatics, Protein Secondary Structure, Learning Classifier System, Markov Reinforcement Learning -
Pages 105-117Analyzing individuals' emotions from the content of social media through text, speech, and images is necessary for various types of applications and purposes. Most recent research studies in the field of sentiment analysis have focused on textual data. However, social media users share more images and videos compared to text. In other words, images are the most effective way to convey emotions to others. Therefore, focusing on the development of a sentiment analysis model based on images in social media is important. In this article, we will use the DenseNet-121 transfer learning model to analyze emotions based on images. To implement this approach, we will utilize the images available in the Image Sentiment dataset. This dataset includes internet links to images along with their emotional polarities. Based on the obtained results, the accuracy of the proposed model in this article is 89%, which, compared to previous work in the field of visual sentiment analysis, shows a 5% to 10% improvement.Keywords: Visual Sentiment Analysis, Transfer Learning, Deep learning, Social networks
-
Pages 118-130In the field of knowledge of philosophical schools, each person's thinking is different according to the type of attitude he can have regarding different schools. Recognizing the author's attitude and its similarity to each of the philosophical schools has always been one of the important issues in the field of humanities. In this article, a method based on deep learning is proposed to distinguish philosophical schools from text. In the proposed method, first the texts are normalized and redundant and meaningless words are removed. After the normalization stage, the text is broken into sentences and words, and then using the fasttext library, each word is converted into a numerical vector, after that, the features of the texts are extracted using the designed network, and finally, the system has learned and is ready to extract data, and by giving a new sentence, its similarity to each school is expressed. Based on the evaluation, the accuracy of the proposed method is 94%.Keywords: Philosophical schools, data mining of text, recognition of schools, conversion of text into a numerical vector, degree of similarity of author', s thinking with schools
-
Pages 131-144
The morphology of the ventricles is used in studies of hydrocephalus, schizophrenia, tumors, trauma, Alzheimer's disease, Parkinson's disease, aging and atrophy to diagnose neurological diseases such as stroke, dementia and Huntington's disease. In this study, an automatic method for morphometric analysis of cerebral ventricles is proposed. Segmentation of cerebral ventricles is an important step to detect the landmarks of cerebral ventricles. Furthermore, the initial estimation of the cerebro-ventricular area can be effective in the proper segmentation of the ventricles. In this regard, Fuzzy C-Means Clustering (FCM) optimized with Harris-Hawk Optimization (HHO) and Aggregate Channel Features (ACF) are used. In order to measure the linear indices of cerebral ventricles, including Evans Index, Bicaudate Ratio, Bicaudate-Frontal Index, Bicaudate-Temporal Index, and Huckman Number, locating a number of landmarks on MRI images is required. This process is based on the geometric features of cerebral ventricles and the use of Hough transformation. The implementation results demonstrate that the proposed algorithm has the best performance with precision 90%, sensitivity 82%, specificity 99%, peak signal-to-noise ratio 77/11, dice similarity coefficient 86%, Jaccard index 75% and contour matching score 92% in the segmentation of cerebral ventricles among other compared methods. Additionally, the results show that the measurement accuracy of the proposed algorithm in the mentioned morphometric linear indices is 97%, 74%, 77%, 76% and 92% respectively.
Keywords: Aggregate Channel Features, Cerebrospinal Fluid Abnormalities, Harris-Hawks Optimizer, Hough Transformation, Indices of Cerebral Ventricles -
Pages 145-159
Extractive summarization of text is an essential technique in natural language processing, which helps to produce compact versions of text by extracting the most important sentences. Since the task of shortening and summarizing a text document is time-consuming and exhausting, an automatic system for creating these short versions of the text seems necessary. In extractive summarization, sentences that contain useful and relevant information are usually selected for the final summary. In order to identify these sentences, there are different algorithms, the performance and summary created by each one is different based on the type and scope of the text and the size of the required summary. In this article, a method called Sa-TRB is presented, which is derived from two algorithms, TextRank and BERT, and in addition to using these two methods, it also uses the common sentences created by other algorithms to achieve high accuracy in selection. Have final summary sentences. The most important criterion for evaluating the performance of algorithms is the quality of their final summary, so the more the final summary created by these algorithms is similar to the summary created by humans, the better the quality of the created summary is. ROUGE criteria have been used to obtain the size of this similarity. Finally, by conducting experiments on the cnn-dailymail dataset with different sizes of summaries, it is shown that the proposed method, by increasing the size of the required summaries, despite the decrease in the recall criterion, has accuracy, score and, as a result, higher quality of the final summaries. So, in the last two tests, the score of the proposed method has reached 24.68 and 23.34%, which is almost one percent better than the best tested methods.
Keywords: TextRank, BERT, LSA, Sa-TRB, ROUGE