فهرست مطالب
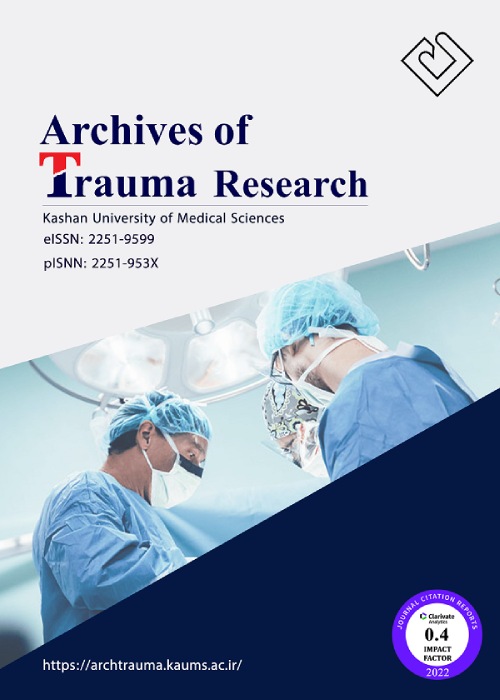
Archives of Trauma Research
Volume:12 Issue: 4, Oct-Dec 2023
- تاریخ انتشار: 1402/09/10
- تعداد عناوین: 7
-
-
Pages 171-178Background
Total Knee Arthroplasty (TKA), a proven and cost-effective orthopedic surgical technique, consistently reduces preoperative morbidity, enhances functional capacity, and alleviates pain in degenerative knee osteoarthritis.
ObjectivesThe aim of this study was to compare cell-based and traditional techniques in Osseointegration in Total Knee Arthroplasty.
MethodsThe literature search was conducted, across various electronic databases including Google Scholar, PubMed, Web of Science, Scopus, and the Cochrane Library. The search was conducted from 2001 to 2022, aiming to identify studies related to TKA and the various techniques employed in the procedure. Articles were screened based on the relevance to cell-based and traditional Osseointegration techniques, focusing on extracting information related to outcomes and complications.
ResultsWe included 11 studies in the study, employing the Cochrane 'Risk of Bias tool' to assess bias in randomized trials across five domains. The majority of articles demonstrated low risk (56.36%), signifying reliability, while 'Unclear' studies (32.73%) had some ambiguity without invalidating results. Studies classified as 'High risk' (10.91%) indicated substantial bias and potential errors. Our findings suggest that cell-based techniques are efficient for the treatment of TKA.
ConclusionOsseointegration presents significant advantages in terms of function and pain relief for patients willing to accept associated risks. Cell-based techniques have proven effective in preventing the need for revision in TKA, demonstrating long-term effects.
Keywords: Knee Arthroplasty, Osseointegration, Cell techniques, Metal implants, osteoarthritis -
Pages 179-185BackgroundTrauma is one of the important causes of disability, death and major health problem in the world. Various instruments are used to assess the clinical outcomes of trauma patients.ObjectivesThe aim of this study was to compare the Revised Trauma Score (RTS) with the MGAP score in determining the clinical outcomes.MethodsA retrospective cross-sectional analytical study was conducted on 1000 multiple trauma patients admitted to Poursina Hospital in Rasht. The data collection instruments included a three-part checklist of demographic and clinical characteristics, RTS and MGAP scores, and clinical outcomes (length of hospitalization and mortality). Data were analyzed using descriptive and non-parametric statistical tests by SPSS 21 software. To determine the predictive power of RTS and MGAP mortality using the ROC test, in addition to obtaining the area under the curve (AUC), the cut-off point, sensitivity, specificity, positive and negative predictive values (PPV and NPV) were obtained.ResultsThe findings showed that 3% of patients (CI: 95%) died and the length of hospitalization was 3.7±2 days. The mortality prediction level of RTS and MGAP instruments for trauma patients was significant (P<0.001) according to AUCs of 97.9% and 98.3%, respectively. Correlation between MGAP and RTS for the length of hospitalization were significant (r=-0.267and r=-0.274, p<0.001), but the intensity of correlation between MGAP and RTS was not significant. The best cut-off points for RTS and MGAP were equal to 7 and 22.5, respectively, with sensitivity rates of 98.1% and 92.3%., specificity rates of 96.7%, and 92.3%., PPV values of 97.7% and 92.3% and NPV values of 92.3% and 98.1%, respectively.ConclusionMAGP and RTS instruments can predict the clinical outcomes of trauma patients well, but they did not have a significant superiority over each other. Therefore, the preferred choice of one of these agents requires multicenter studies.Keywords: revised trauma score, MGAP, clinical outcomes, Trauma
-
Pages 186-194BackgroundDisasters occur as a result of the interaction between hazards and the societal conditions. These conditions that turn hazards into disasters are referred to as vulnerability. Vulnerability has different dimensions; one of its important dimensions is the social dimension.ObjectivesThis study was conducted to explore social factors influencing disaster risk and vulnerability in Islamic Republic of Iran's social context.MethodsThis study was conducted with a qualitative approach. Data were collected through in-depth semi-structured interviews with experts who were purposefully selected. Collected data were analyzed and coded manually using the conventional content analysis technique. The trustworthiness of the study findings was checked by Guba and Lincoln's criteria (credibility, conformability, dependability and transformability).ResultsIn this study, 16 disaster management experts were interviewed. The coding process of the interviews led to the formation of 243 codes, which were merged and 10 categories of social factors affecting disaster risks and vulnerability were identified: personal characteristics, community risk perception, employment, quality of residence, social capital, disaster/risk governance, religious beliefs, economical condition, communication/social isolation, and the existence of infrastructures.ConclusionMany social factors cause increased disaster risks and vulnerabilities. These factors are affected by the characteristics of societies and act differently in each society. This study has identified and introduced these factors from the point of view of experts, which can be used by policymakers in this field.Keywords: Social factors, disaster, vulnerability, Emergencies
-
Pages 195-200BackgroundWith severe situation in road traffic safety, there is an urgent need to study the risk factors that determine driving violations and the severity of road accidents.ObjectivesThe aim of the present study was to investigate the impact of driving violations and risky driving behaviors on the risk of accidents.MethodsIn this descriptive cross-sectional study, 320 professional drivers participated. Data of this study were collected from Occupational Medicine Center of Kashan, Truckers’ Cooperative, and Aran and Bidgol Kavir Steel Company using the Persian version of the Driver Behavior Questionnaire (DBQ). Data were analyzed using structural equation modelling by IBM SPSS Statistics 22 and IBM AMOS 19.ResultsAccording to the results, the fit indices of the final model showed a good fit (X2/df=3.07, RMSEA=0.083, CFI=0.977, NFI=0.967, TLI=0.957). All three research hypotheses were confirmed in the final model. There was a positive and significant relationship between violating traffic laws and risky driving behaviors (β=0.56), and the risk of driving accidents (β=0.41). Moreover, there was a positive and significant relationship between risky driving behaviors and the risk of driving accidents (β=0.52, P>0.01).ConclusionThe results of this study reveal that if the traffic violation rate could be reduced or controlled successfully by strict law enforcement and continuous monitoring, then the rate of serious injuries and fatalities would be reduced accordingly.Keywords: Traffic violation law, Traffic Accident, Dangerous driving behaviors, Heavy Vehicle, occupational driver
-
Pages 201-208BackgroundRoad traffic accidents (RTAs) are the leading cause of death for children and young adults aged 5 to 39 and the third-leading cause of death in Iran.ObjectivesThe aim of this study was to assess in-hospital costs of patients following RTAs and identify associated factors among the patients admitted to Sina Hospital, a collaborating hospital with the National Trauma Registry of Iran (NTRI).MethodsA hospital-based registry study was conducted between September 17, 2016, and April 30, 2022, involving 3245 patients affected by RTAs. Data collection utilized the NTRI minimum dataset. To assess the factors influencing in-hospital costs of RTAs, various statistical analyses were performed, including independent sample t-tests, one-way ANOVA, and multiple linear regression analysis.ResultsThe distribution of studied subjects based on sex, age, and road user group revealed that the majority were male (91%), within the working age range of 21-60 years (78.2%), and motorcyclists (55.2%). The mean in-hospital cost per patient following RTAs was 152.3 million Iranian Rial (IRR) ($609.35). In the multiple linear regression analysis, several factors were significantly associated with higher in-hospital costs (ps<0.05). These factors included undergoing surgery, admission to the intensive care unit (ICU), the year of admission, having a moderate injury severity, being a car occupant, being female, and having a longer length of stay (LOS) at the hospital. Patients who required surgery had an additional cost of 102 million IRR, while those admitted to the ICU had an additional charge of 43.4 million IRR (ps<0.001).ConclusionsThe study findings revealed that in-hospital costs resulting from RTAs were influenced by factors such as gender, injury severity, road user group, ICU admission, surgery operation, and LOS at the hospital. The mean in-hospital costs per patient were relatively high, amounting to 152.3 million IRR ($609.35). These findings highlight the need for increased efforts to mitigate the burden of RTAs in the country and implement measures to reduce their occurrence.Keywords: Hospital Costs, Traffic Accident, trauma registry
-
Pages 209-216BackgroundTrauma is one of the main causes of premature deaths worldwide. Improvements in hospital and pre-hospital care and procedures can reduce trauma-related deaths. Easy trauma scoring systems can help the doctors to adopt a specific and appropriate method of managing trauma patients.ObjectivesThis study aimed to compare the degree to which indicators of GCS, Age, and systolic blood pressure (GAP), New trauma score (NTS), Revised trauma score (RTS), and Kampala trauma score (KTS) predicted the hospital outcome of the multi-trauma patients.MethodsThis descriptive-analytical study was conducted on 385 multi-trauma patients referred to the emergency department of Imam Khomeini hospital in Urmia (Iran). The data related to GAP, NTS, RTS, and KTS were collected using a checklist and then were analyzed using descriptive and analytical statistics by SPSS 18.ResultsThe mean RTS, NTS, KTS, GAP and GCS values were 6.71±0.47, 6.06±0.34, 8.25±0.96, 77.77±0.96, 22.20±2 and 13.90±1.8, respectively. Moreover, the deceased patients’ averages of RTS, NTS and GAP were significantly lower than these averages in the discharged patients. Nonetheless, there was no significant difference between deceased and discharged patients in terms of their average KTS. Moreover, there was no statistically significant difference between the average RTS, NTS, KTS and GAP of the patients who needed surgery and the patients who did not need surgery. In addition, the averages RTS, NTS and GAP of the patients who needed ICU were significantly lower than these averages in patients who did not need ICU. However, there was no significant difference between the average KTS of the patients who needed ICU and the patients who did not need ICU.ConclusionThe findings of the study show that the deceased trauma patients’ RTS, NTS, KTS and GAP were lower than the recovered patients’ RTS, NTS, KTS and GAP. This problem can be a risk factor and shows that there is a need for faster treatment of these patients.Keywords: New trauma score (NTS), Revised trauma score (RTS), Kampala trauma score (KTS), GCS, age, and systolic blood pressure (GAP), Glasgow Coma Scale (GCS), Trauma
-
Pages 217-219
Dear Editor Traumatic brain injury (TBI) is a common cause of death and disability, with long-term consequences including cognitive impairment, emotional disturbances and physical disability. Prognosis prediction in TBI patients is critical for guiding treatment decisions and improving patient outcomes. The Glasgow coma scale (GCS) and Glasgow outcome scale (GOS) are useful tools to assess and monitor TBI patients, but other factors such as age, motor component of GCS, and type of injury also significantly influence clinical outcomes. With the advent of artificial intelligence (AI), there is growing interest in using machine learning algorithms to predict prognosis in TBI patients.[1] Here we discuss the harnessing of AI in prognosis prediction for TBI patients, as well as its potential benefits, disadvantages, challenges and predicted future directions. Current state of AI in prognosis prediction for TBI patientsReflecting on large bodies of data (clinical, radiographic and laboratory), AI has shown promise in predicting prognosis in TBI patients. Machine learning algorithms (MLA) can identify patterns in data that may not be immediately apparent to human clinicians, allowing for more accurate predictions of patient outcome. There are several studies that have used MLA to develop diagnostic systems for TBI and predict patient outcomes. These studies have used various clinical inputs such as vital signs, electroencephalography (EEG), hospital volume, Charlson comorbidity index and length of stay to develop machine learning (ML) models.[2] In a study conducted by Mawdsley et al, the effectiveness of ML models in predicting psychosocial aspects of TBI cases was systematically reviewed. The study included nine studies with eleven types of ML used to predict various outcomes of traumatic brain injury, concussion and psychosocial outcomes. The results indicated that while these models were able to develop predictive models successfully, there is insufficient evidence to consider ML algorithms as a dependable tool for clinical decision-making.[3] Alanazi et al., conducted a review in 2017 to assess the effectiveness of ML models in predicting patient outcomes for various disorders. The study revealed that AI has the potential to create several promising models that can predict outcomes using clinical, demographic, and imaging data. However, there are still limitations in applying these models in clinical settings. Therefore, further research is necessary to improve the reliability of these models in the future.[4] Potential benefits of AI in prognosis prediction for TBI patientsThe use of AI in prognosis prediction for TBI patients has several potential benefits. First and foremost, it can provide clinicians with more accurate and reliable predictions of patient outcomes, allowing for more informed treatment decisions. This can lead to improved patient outcomes and reduced healthcare costs. AI can help identify patients who are at high risk for poor outcomes, allowing for early interventions that may improve their prognosis, or early decision making around withdrawal of treatment. For example, if an algorithm predicts a patient is at high risk of adverse effects from an intracranial hemorrhage, clinicians can perform early surgical interventions or administer medications early to prevent further bleeding. In addition, AI can help identify patients who are likely to have favorable outcomes, or who do not require intervention, which allows for more efficient distribution of healthcare resources. For example, if an algorithm predicts a patient is likely to have a good outcome, they may not require intensive care unit (ICU) admission, or may not require prolonged rehabilitation services. Finally, AI can also help reduce the workload of medical professionals by performing repetitive tasks such as data entry and analysis. This can free up time for physicians and nurses to focus on providing high-quality care to patients.[1,5] Potential disadvantages of AI in prognosis prediction for TBI patientsThere are several potential disadvantages of using AI in predicting the clinical outcomes of patients with TBI. AI algorithms rely on large amounts of data to make accurate predictions. However, there may not be enough high-quality data available to train AI models effectively. Data entry relies on human factors and may be poorly or incorrectly entered, making errors possible. Also, AI algorithms may be biased if the data used to train them is not representative of the population being studied. For example, if the data used to train an AI model on TBI outcomes comes from a specific hospital or region, the model may not generalize well to other populations. AI models can be complex and difficult to interpret. Clinicians may struggle to understand how the model arrived at a particular prediction, which could lead to skepticism or mistrust of the technology. Finally, there are ethical considerations around the use of AI in healthcare. If an AI model predicts that a patient has a poor prognosis, this could lead to decisions about end-of-life care, which may go against the patient's wishes or values. Clinicians must carefully consider the potential risks and benefits of using AI in clinical decision-making.[1,5] Future DirectionsThe future of prognosis prediction in TBI patients using AI is promising. However, there are several challenges that must be addressed. One challenge is the lack of standardized data collection and reporting. To develop accurate algorithms, large amounts of high-quality data are required. However, there is currently no standardized way to collect and report TBI data, making it difficult to develop accurate, widely transferable algorithms. Another challenge is the need for validation studies. While initial research has shown promising results, further validation studies are needed to confirm the accuracy and reliability of AI algorithms in predicting TBI prognosis,[3,4] as well as ensuring safety in clinical practice.[1,6]With the increasing availability of electronic health records and the development of advanced AI algorithms, there is potential for more accurate and reliable predictions of TBI outcomes. AI can help identify patterns and correlations in patient data that may not be easily recognizable by human clinicians, leading to more personalized and effective treatment plans, however, there is the challenge of integrating AI into clinical practice. Clinicians must be trained on how to interpret and use AI predictions in their decision-making processes. This will require collaboration between clinicians and AI experts to develop user-friendly interfaces that can be integrated into clinical workflows. ConclusionThe reliability of prognosis prediction in TBI patients using AI depends on the quality and completeness of the data, the type of algorithm used and the level of validation testing performed. Conclusions surrounding the selection of optimal clinical or para-clinical features and the most precise machine learning model for predicting outcomes in TBI patients is still inconsistent. However, early studies have shown that AI algorithms can accurately predict mortality and functional outcomes in TBI patients by analyzing large amounts of patient data, including clinical, radiographic, and laboratory data. Therefore, further validation studies are needed to confirm the accuracy and reliability of AI algorithms in predicting TBI prognosis before they can be integrated into clinical practice. With continued research and collaboration between clinicians and AI experts, the use of AI in prognosis prediction for TBI patients has the potential to revolutionize TBI care and improve patient outcomes.
Keywords: Traumatic Brain Injury, Artificial intelligence, Machine Learning Algorithms