فهرست مطالب
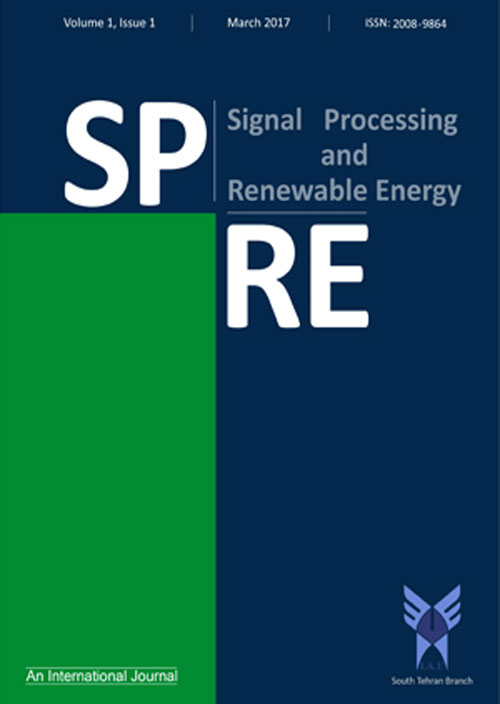
Signal Processing and Renewable Energy
Volume:5 Issue: 2, Spring 2021
- تاریخ انتشار: 1400/03/17
- تعداد عناوین: 6
-
Pages 1-13Solving medical image diagnosis and image analysis problems has long been thought of in many research types in the last decades. Mostly the interpretations of medical data are being made by a medical expert. In terms of image interpretation by a human expert, it is entirely limited due to its subjectivity, complexity, extensive variations across different interpreters, and fatigue. After the success of deep learning in other real-world applications, it provides exciting solutions with reasonable accuracy for medical imaging and is seen as a critical method for future applications in the health sector. This study proposed the deep learning approach based on soft-max activation function to obtain more reliable medical image diagnosis results properly. The proposed model evaluated a brain tumor (MRI) medical dataset, alongside an assessment in terms of accuracy, MSE, and RMSE, as a qualitative analysis of the learned features and practical commendations. This research should help improve the application and refinement of the evaluated approaches in the future.Keywords: deep learning, Medical Image Analysis, Machine Intelligence
-
Pages 15-34
This paper proposes a robust method for frequency control in an islanded microgrid facing solar unit uncertainties. Here, microgrids with photovoltaic panels, battery energy storage systems, diesel generators, and fuel cells are considered. This paper focuses on the frequency constancy of stand-alone microgrids for reaction to these uncertainties. There are so many ways to control the frequency of microgrids, such as the PI control method, but these methods can't be useful in the case of uncertain systems. Due to the small scale of energy generation, the microgrid inertia changes with these uncertainties. Robust control is a method for frequency control that can be used in a situation of uncertainties, perturbations and frequency instability. In this article H_∞ frequency control is used for a microgrid with a dual peak load profile in the case of different coefficient penetrations of the solar panel. The results demonstrated that the battery energy storage system's coefficient penetration and the solar cell significantly affect microgrid frequency. A smart algorithm is proposed to determine the best coefficient penetration value for battery energy storage system available electricity and solar panels.
Keywords: Battery energy storage system, islanded microgrid, Robust frequency control, Solar cell penetration, Uncertainty parameters -
Pages 35-51Due to the growing future of blockchain applications such as smart contracts and the widespread use of smart contracts in the Internet of Things (IoT) and the lack of Persian resources in this field, it is necessary to conduct comprehensive and integrated researches for a systematic study of past studies and provide interpretive perspectives and create new knowledge. In this research, using mixed research method (first, using the Meta-Synthesis Research Method, by combining qualitative findings of previous studies and combining different perspectives, a model in the process of smart contracts on the blockchain platform is presented and then for evaluation and analysis of the modeled, structural equations quantitative method and confirmatory factor analysis with Smart PLS3 software have been used. The lack of a standard framework for analyzing and comparing smart contract-based systems identifies the need to design a smart contract model that allows smart contract developers to identify bottlenecks and improve system-based smart contracts.Keywords: Smart Contract Model, Smart Contract Process, design Smart Contract, Smart Contract Architecture, Smart Contract platform
-
Pages 53-60Sleep stages classification using the signal analysis includes EEG, EOG, EMG, PPG, and ECG. In this study, the proposed method using transfer learning to sleep stages classification. First, we have used the two PPG signals for this method It is important to use a less complex signal. The PPG signal has the least complexity, and in this article, we used this signal for transitional learning. In this study, we extracted 52 features from two signals and prepared them for the classification stage. This method includes two steps, (a) Train data PPG1 and Test data PPG2, (b) Train data PPG2 and Test data PPG1. Results proved that our method has acceptable reliability for classification. The accuracy of 94.26% and 96.49% has been reached.Keywords: PPG signal, sleep stages classification, deep transfer learning
-
Pages 61-82The emergency demand response program (EDRP) is a type of program that can be utilized as a tool for controlling the price of electricity when there is a lack of reliability in the distribution system. In this study, a formulation is proposed for determining the optimum amount of demands in the EDRP according to the viewpoints of the regional market manager (RMM) aimed at reducing the EDRP costs and smoothening the load curve based on the logarithmic model and the matrix of demand elasticity. The probability that the aggregators should present their available reserves to the RMM in response to the received incentives has also been included in the proposed formulations. The market manager then prioritizes the available reserves using the reserve-margin factor (RMF). Three algorithms including co-evolutionary particle swarm optimization (C-PSO), co-evolutionary teaching learning-based optimization (C-TLBO) and co-evolutionary improved teaching learning-based optimization (C-ITLBO) are used for reducing the EDRP costs. The results show that the proposed formulations are effective in improving the economic performance of the regional market and the load curve. Furthermore, the results indicate the superiority of the C-ITLBO algorithm in terms of the total cost, incentive rate and peak shaving in comparison with C-PSO and C-TLBO algorithms.Keywords: Emergency demand response program, Electricity market, logarithmic model, Reserve margin factor, Co-evolutionary improved teaching learning-based optimization
-
Pages 83-97One of the trends in modern management is the consideration of the principle of customer orientation and customer satisfaction. Following this approach, online shopping, as one of the goods and services distribution channels, is also inclined to maintain and expand relations with customers. Nonetheless, what has become even more highlighted in the competition arena is going beyond customer satisfaction by predicting the customers’ behavior in order to properly respond to their needs and ultimately, establish loyalty. One of the methods used to know the customers is the clustering approach. Clustering is a data mining technique that takes a number of items and places them in clusters based on their attributes. One of the problems of the k-means clustering is that it has no specific method for primary determination or calculation of the cluster centers. Therefore, in order to optimize the clusters, we use the particle swarm optimization (PSO) algorithm. In the end, we analyze these clusters using the RFM model to analyze the customers’ behavior. What is achieved by analysis of each cluster is finding the cluster of the most loyal customers. In this study, we specify the number of optimized clusters using the k-means clustering algorithm. Then, we use the obtained number of clusters for the primary adjustment in the particle swarm optimization algorithm. Finally, we score the achieved clusters by the RFM method so that we can identify the most loyal customers that are placed in the cluster with the highest score.Keywords: Commercial Websites, Clustering, Customer Behavior Analysis, k-means clustering, Particle Swarm Optimization (PSO)