فهرست مطالب
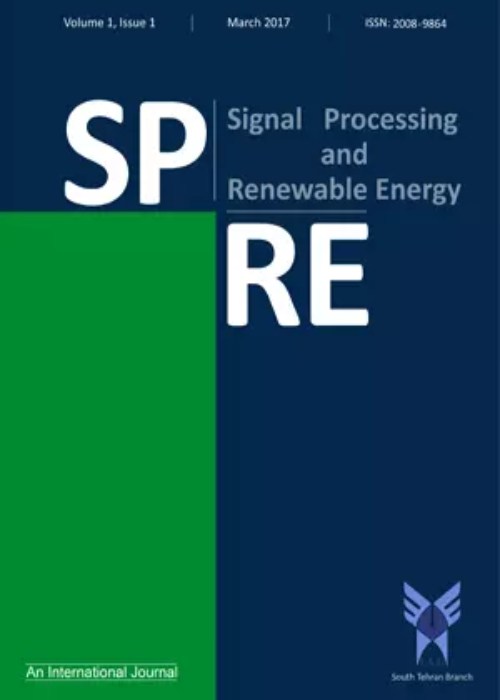
Signal Processing and Renewable Energy
Volume:7 Issue: 4, Autumn 2023
- تاریخ انتشار: 1402/09/10
- تعداد عناوین: 6
-
Pages 1-12Renewable energy sources offer geographical opportunities for enhanced energy efficien-cy. However, the substantial integration of renewable energy production into distribution systems introduces uncertainties and technical complexities by impacting the network per-formance and protection mechanisms. Distributed generation (DG) enhances power grid efficiency, yet its influence on protection systems raises concerns about fault detection and isolation within active distribution networks. This study introduces a method to coordinate protection devices by inverter current control of distributed production sources during faults. The paper compares the impact of dispersed resources on flow dynamics during fault conditions. ETAP software simulations validate the proposed approach, addressing challenges in coordinating and regulating protection devices when integrating diverse re-sources into the network. This method restores coordination and enhances the reliability of protection systems. In summary, this research emphasizes the challenges of integrating re-newable energy into distribution systems, highlighting the need for effective protection co-ordination. The proposed method contributes to mitigating these challenges, ensuring de-pendable and efficient active distribution networksKeywords: distributed generation, Distribution network, Power system protection, renewable energy sources, Resource impact
-
Pages 13-21Listening to the sound signals of the heart is considered one of the most non-invasive and easy ways to diagnose the irregularities of the human heart, the correct analysis of which requires the knowledge and experience of a specialist doctor. The purpose of this paper is to design and implement a computer-aided diagnosis (CAD) system for detecting and classifying normal and abnormal heart sounds from phonocardiogram (PCG) signals. To perform experiments, the PhysioNet database was used. In the pre-processing step, noise and environmental disturbances in the PCG signals are removed using band-pass Butterworth filters. Then, discrete wavelet transforms (DWT), group-based Sparse, and tensor decomposition are used to extract features from PCG signals. Finally, the support vector machine (SVM), the k-nearest neighbors (KNN), naive Bayes (NB), the classification and regression tree (CART), and multi-layer perceptron (MLP) were used for the classification step. The employment of DWT, group-based sparse, and tensor decomposition for detection features is the novelty of this paper. The proposed method demonstrated better performance compared to other methods used in different papers. The proposed DWT, group-based sparse and tensor decomposition-NB method had a high accuracy rate of 95.3%. Also, the combination of PCG feature extraction methods increases the accuracy of the CAD system in diagnosing abnormal heart sounds. The proposed method in this paper uses different methods for extracting features, and their classification has high accuracy for abnormal sound detection.Keywords: Classification of heart sounds, murmur, Group-based sparse, tensor decom-position, Discrete Wavelet transform
-
Pages 23-40The objective of this study is to strategize the allocation of resources within the distribution microgrid. The primary goals are to diminish active power loss and voltage discrepancies, enhance resilience, decrease the expense of procuring energy from the main network, augment the incorporation of distributed renewable energy sources, and optimize the profitability of integrating distributed generation and storage resources into the power supply. In this article, the microgrid design challenge is approached through the focus on a nonlinear optimization model. The Biogeography-based optimization algorithm is employed to tackle this complex problem. Moreover, the planning solution is crafted to address uncertainties, incorporating factors such as wind speed, solar radiation, and consumption load variability within the distribution network. To validate the efficacy of the proposed methodology, the standard 33-bus test system is scrutinized. The outcomes underscore the remarkable efficiency of the proposed solution, showcasing its potential for practical applications in optimizing microgrid performance.Keywords: independent microgrid, distributed wind generation resources, distributed solar generation resources, Energy storage, biogeography-based algorithm
-
Pages 41-57The fuel cell, especially its PEM type, is one of the most important sources of renewable energy, and considering the importance of energy in today's life, this paper presents a new solution to increase and optimize the output power of the PEM fuel cell. To extract the maximum power, the structure of PEM fuel cell equipped with boost converter is considered and this study presents the following innovations in this regard. To overcome the non-minimum phase and destabilizing nature of the boost converter, a hybrid robust control scheme is designed for the boost converter located at the output of the fuel cell stack. Two reset and adaptive sliding mode techniques in an innovative and hybrid design form the foundations of the planned scheme. Despite its simplicity, the reset technique has a high ability to eliminate the steady-state error, improve the transient and permanent dynamic response, and of course overcome the limitations of linear controllers. The planned sliding mode control technique is also an adaptive super twisting type, which is used to ensure the robustness and reliable performance of the controller against uncertainties and disturbances. The planned control scheme increases the power extracted from the PEM fuel cell by improving the performance of the boost converter and provides more efficiency than other techniques by stabilizing the output voltage from the PEM fuel cell and stack as well as ensuring stability. The outcomes of the implementation in the MATLAB environment and evaluation with the PI adaptive sliding mode method guarantee the efficiency and capability of the scheduled robust design.Keywords: PEM Fuel Cell, Boost converter, reset robust control, adaptive super twisting sliding mode control, Renewable Energy
-
Pages 59-67This study presents an innovative approach to ship tracking utilizing the analysis of propeller noise. It employs a hybrid technique that combines both time-domain and frequency-domain processing. In the frequency-domain aspect, the method utilizes matched field processing (MFP), which can be customized using diverse propagation models such as the normal modes, the image model, and the Lloyd-Mirror. Within this framework, an approximated time-domain frequency-limited covariance matrix is integrated, incorporating attributes of the Image propagation model, and coupled with the Multiple Signal Classification (MUSIC) algorithm. This integrated methodology leverages the strengths of both time-domain and frequency-domain strategies simultaneously. To validate the effectiveness of the proposed algorithm, actual data from a field trial conducted in collaboration with JASCO Applied Sciences Ltd at Duncan’s Cove, Canada, in September 2020 is employed. The trial involved deploying a hydrophone array in the outbound shipping lane, which collected broadband noise data from various types of vessels, enabling the estimation of vessel bearings. The results from this real-world trial demonstrate the practical performance of the proposed algorithm.Keywords: Matched Field Processing, MUSIC algorithm, Tracking ship noise, Underwater acoustic, Normal-mode model
-
Pages 69-86Advanced data processing methods are emerging on a daily basis and they can be used in many tasks. Short-term load forecasting (STLF) is an essential task for power distribution systems. Specially, for the electric vehicle (EV) charging stations and parking lots the STLF can give precise time tables in which the electrical power demand is at the lowest and the electric prices are low too. In this paper, we developed a STLF system that works based on wavelet transform of the load data collected from the previous 3 months. Next, we perform the feature selection using the Gram-Schmidt (GS) procedure. The selected features are then fed to the Radial basis function (RBF) network in order to predict the power usage one day ahead. The learning algorithm that is used for the RBF network is the hybrid "k-means, RLS" algorithm. It is indicated that the proposed RBF network works more accurately or at least equal to the previously presented support vector machine (SVM) predictor. Also, the computational complexity of the RBF network is much less than SVM and consequently, the time consumption of the presented system is far less than recently proposed methods. The numerical simulation results based on the real-world load data of the Urmia city showed that the proposed method based on the wavelet transform features and the hybrid RBF is more efficient than the previous SVM both in accuracy (more than 29%) and computational complexity (more than 30%).Keywords: RBF network, Short Term Load Forecasting, RLS Algorithm, hybrid learning, performance