فهرست مطالب
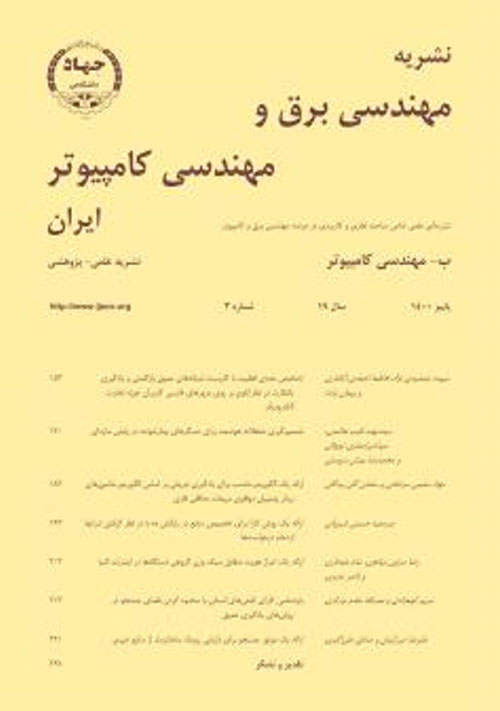
نشریه مهندسی برق و مهندسی کامپیوتر ایران
سال نوزدهم شماره 3 (پیاپی 65، پاییز 1400)
- ب - مهندسی کامپپوتر
- تاریخ انتشار: 1400/09/30
- تعداد عناوین: 7
-
-
صفحات 153-169
نظرکاوی، زیرشاخهای از دادهکاوی است که به حوزه پردازش زبان طبیعی وابسته بوده و با گسترش تجارت الکترونیکی، به یکی از زمینههای محبوب در بازیابی اطلاعات تبدیل شده است. این حوزه بر زیرمجموعههای مختلفی مانند تشخیص قطبیت، استخراج جنبه و تشخیص هرزنظر تمرکز دارد. اگرچه وابستگی نهانی بین این زیرمجموعهها وجود دارد اما طراحی یک چارچوب جامع شامل تمامی این موارد، بسیار چالشبرانگیز است. پژوهشهای موجود در این حوزه اکثرا بر روی زبان انگلیسی بوده و برای تحلیل احساس، بدون توجه به زیرمجموعههای تاثیرگذار، فقط بر روی حالت باینری تمرکز داشتهاند. همچنین استفاده از یادگیری ماشینی برای دستهبندی نظرات بسیار رایج است و در سالهای اخیر، اغلب پژوهشها از یادگیری عمیق با اهداف متفاوت استفاده کردهاند. از آنجا که در ادبیات پژوهشی به چارچوبی جامع با تمرکز بر زیرمجموعههای تاثیرگذار کمتر پرداخته شده است، از این رو در مقاله حاضر با استفاده از راهکارهای نظرکاوی و پردازش زبان طبیعی، چارچوب جامع مبتنی بر یادگیری عمیق با نام RSAD که پیشتر توسط نویسندگان این مقاله در حوزه نظرکاوی کاربران فارسی زبان توسعه داده شده بود برای تشخیص قطبیت در دو حالت باینری و غیر باینری جملات با تمرکز بر سطح جنبه بهبود داده شده که تمام زیرمجموعههای لازم برای تحلیل احساس را پوشش میدهد. مقایسه و ارزیابی RSAD با رویکردهای موجود، نشاندهنده استحکام آن است.
کلیدواژگان: پردازش زبان طبیعی، تحلیل احساس، تشخیص قطبیت جملات، تشخیص هرزنظر، شبکه های عصبی عمیق، نظرکاوی -
صفحات 170-182
امروزه از ساختمانهای اداری و مسکونی گرفته تا ابنیه تاریخی و ساختمانهای حساس و پراهمیت، نیاز به مراقبت و پایش ویژه دارند. بدیهی است چنین پایشی دارای هزینه، خطا و چالشهای بسیاری میباشد. شبکههای حسگر سیمی به دلایلی نظیر هزینه کمتر، کابردهای گستردهتر و نصب آسان در موارد زیادی در حال جایگزینی با شبکههای حسگر بیسیم هستند. در سازههای مختلف بسته به وضعیت و نوع سازه، مواردی نظیر مصرف انرژی، دقت و همچنین تحمل اشکال در از بین رفتن گرههای حسگر حایز اهمیت میباشند. بالاخص که با استفاده از شبکههای حسگر بیسیم، موارد یادشده، چالشهایی دایمی هستند که با وجود تحقیقات صورتگرفته، ظرفیت بهبودیافتن دارند. ایده اصلی مقاله پیش رو عبارت است از استفاده رویکردی نوآورانه در به کارگیری از فرایند تصمیمگیری مارکوف و حسگرهای بیدارشونده، تا به این وسیله هزینه و خطا در پایش سازههای پایا و نیمهپایا را نسبت به روشهای فعلی کاهش دهیم و بر اساس صورت مسیله، مزایایی را در پیادهسازی و اجرا به همراه داشته باشیم. بنابراین نوآوری روش پیشنهادی، استفاده از فرایند تصمیمگیری مارکوف و حسگرهای بیدارشونده به منظور ارایه روشی نوین و بهینهتر نسب به روشهای موجود به صورت اختصاصی برای پایش سلامت سازهای سازههای پایا و نیمهپایا است. این رویکرد در قالب شش گام تشریح شده است و در مقابل، روشهای پرکاربردی مورد مقایسه قرار گرفتهاند بدین گونه که در محیط شبیهسازی CupCarbon، با سنجههای مختلفی آزمایش و شبیهسازی شدهاند. نتایج نشان میدهد راهکار پیشنهادی در مقایسه با راهکارهای مشابه در موارد کاهش مصرفی انرژی از 11 تا 70 درصد، تحملپذیری اشکال در تبادل پیامها از 10 تا 80 درصد و همچنین در مبحث هزینه کل از 93 تا 97 درصد بهبود به دست آورده است.
کلیدواژگان: پایش سلامت سازه ای، شبکه های حسگر بی سیم، فرایند تصمیم گیری مارکوف، حسگرهای بیدارشونده -
صفحات 183-192
الگوریتم ماشین بردار پشتیبان یکی از الگوریتمهای مشهور و با کارایی بالا در یادگیری ماشین و کاربردهای مختلف است. از این الگوریتم تا کنون نسخههای متعددی ارایه شده که آخرین نسخه آن ماشینهای بردار پشتیبان دوقلوی مربعات حداقلی فازی میباشد. اغلب کاربردها در دنیای امروز دارای حجم انبوهی از اطلاعات هستند. از سویی دیگر یکی از جنبههای مهم دادههای حجیم، جریانیبودن آنها میباشد که باعث شده است بسیاری از الگوریتمهای سنتی، کارایی لازم را در مواجهه با آن نداشته باشند. در این مقاله برای نخستین بار نسخه افزایشی الگوریتم ماشینهای بردار پشتیبان دوقلوی مربعات حداقلی فازی، در دو حالت برخط و شبه برخط ارایه شده است. برای بررسی صحت و دقت الگوریتم ارایهشده دو کاربرد آن مورد ارزیابی قرار گرفته است. در یک کاربرد، این الگوریتم بر روی 6 دیتاست مخزن UCI اجرا شده که در مقایسه با سایر الگوریتمها از کارایی بالاتری برخوردار است. حتی این کارایی در مقایسه با نسخههای غیر افزایشی نیز کاملا قابل تشخیص است که در آزمایشها به آن پرداخته شده است. در کاربرد دوم، این الگوریتم در مبحث اینترنت اشیا و به طور خاص در دادههای مربوط به فعالیت روزانه به کار گرفته شده است. طبق نتایج آزمایشگاهی، الگوریتم ارایهشده بهترین کارایی را در مقایسه با سایر الگوریتمهای افزایشی دارد.
کلیدواژگان: شبکه های روی تراشه، مسیریابی، تحمل پذیری خطا، مسیریابی انطباقی، قابلیت اطمینان -
صفحات 193-202
امروزه با توسعه بسیار سریع فناوریهای نوین در حوزه اینترنت اشیا و شبکههای هوشمند، مفهوم شبکههای حسگر بیسیم بیش از هر زمان دیگری مورد توجه مراکز تحقیقاتی قرار گرفته است. در سالهای اخیر، پیدایش این شبکهها با ساختار متراکم، بر اهمیت به کارگیری فناوریهای مخابراتی از جمله فناوری فراپهن باند با قابلیت اطمینان بالا، کاربرد صنعتی و همچنین امنیت ارتباطی مناسب افزوده است. اما همچنان نگرانیهای بسیاری در ارتباط با میزان تداخل درون شبکهای به ویژه ناشی از خطوط گسسته طیفی نامطلوب در این فناوری مطرح هستند و بنابراین ارایه یک راهکار بهینه برای حذف تداخل درون شبکه و کنترل طیف توان و سپس تعریف ساختارهای فرستنده- گیرنده مطلوب البته با در نظر گرفتن حساسیتهای بالا نسبت به مساله سنکرونسازی در شبکههای حسگری بیسیم مبتنی بر تکنولوژی فراپهن باند ضروری است. این اهداف در تحقیق کنونی با اعمال استراتژی بهینه طیفی در مدل سیگنال، ساختار حسگر فرستنده و سپس ترسیم ساختارهای حسگر گیرنده بهینه و یا زیربهینه دنبال میشوند که نتایج به دست آمده بیانگر بهبود عملکرد ارتباطات در شبکههای حسگر بیسیم است.
کلیدواژگان: شبکه های حسگر بی سیم، اینترنت اشیا، تداخل درون شبکه، ساختار فرستنده- گیرنده -
صفحات 203-212
کارایی سیستمهای بازشناسی کنشهای انسانی به استخراج بازنمایی مناسب از دادههای ویدیویی وابسته است. در سالهای اخیر روشهای یادگیری عمیق به منظور استخراج بازنمایی فضایی- زمانی کارا از دادههای ویدیویی ارایه شده است، در حالی که روشهای یادگیری عمیق در توسعه بعد زمان، پیچیدگی محاسباتی بالایی دارند. همچنین پراکندگی و محدودبودن دادههای تمایزی و عوامل نویزی زیاد، مشکلات محاسباتی بازنمایی کنشها را شدیدتر ساخته و قدرت تمایز را محدود مینماید. در این مقاله، شبکههای یادگیری عمیق فضایی و زمانی با افزودن سازوکارهای انتخاب ویژگی مناسب جهت مقابله با عوامل نویزی و کوچکسازی فضای جستجو، ارتقا یافتهاند. در این راستا، سازوکارهای انتخاب ویژگی غیر برخط و برخط، برای بازشناسی کنشهای انسانی با پیچیدگی محاسباتی کمتر و قدرت تمایز بالاتر مورد بررسی قرار گرفته است. نتایج نشان داد که سازوکار انتخاب ویژگی غیر برخط، منجر به کاهش پیچیدگی محاسباتی قابل ملاحظه میگردد و سازوکار انتخاب ویژگی برخط، ضمن کنترل پیچیدگی محاسباتی، منجر به افزایش قدرت تمایز میشود.
کلیدواژگان: احراز هویت سبک وزن، احراز هویت گروهی، اینترنت اشیا، توافق کلید -
صفحات 213-220
کارایی سیستمهای بازشناسی کنشهای انسانی به استخراج بازنمایی مناسب از دادههای ویدیویی وابسته است. در سالهای اخیر روشهای یادگیری عمیق به منظور استخراج بازنمایی فضایی- زمانی کارا از دادههای ویدیویی ارایه شده است، در حالی که روشهای یادگیری عمیق در توسعه بعد زمان، پیچیدگی محاسباتی بالایی دارند. همچنین پراکندگی و محدودبودن دادههای تمایزی و عوامل نویزی زیاد، مشکلات محاسباتی بازنمایی کنشها را شدیدتر ساخته و قدرت تمایز را محدود مینماید. در این مقاله، شبکههای یادگیری عمیق فضایی و زمانی با افزودن سازوکارهای انتخاب ویژگی مناسب جهت مقابله با عوامل نویزی و کوچکسازی فضای جستجو، ارتقا یافتهاند. در این راستا، سازوکارهای انتخاب ویژگی غیر برخط و برخط، برای بازشناسی کنشهای انسانی با پیچیدگی محاسباتی کمتر و قدرت تمایز بالاتر مورد بررسی قرار گرفته است. نتایج نشان داد که سازوکار انتخاب ویژگی غیر برخط، منجر به کاهش پیچیدگی محاسباتی قابل ملاحظه میگردد و سازوکار انتخاب ویژگی برخط، ضمن کنترل پیچیدگی محاسباتی، منجر به افزایش قدرت تمایز میشود.
کلیدواژگان: بازشناسی کنش های انسانی، یادگیری عمیق، فضایی- زمانی، پیچیدگی محاسباتی، سازوکار انتخاب ویژگی -
صفحات 221-227
تحلیل محتوای اخبار منتشرشده، یکی از مسایل مهم در حوزه بازیابی اطلاعات است. امروزه تحقیقات زیادی برای تحلیل تکتک مقالات خبری انجام شده است، در حالی که اکثر رویدادهای خبری به شکل چندین مقاله مرتبط به هم به طور مکرر در رسانهها منتشر میشوند. تشخیص رویداد، وظیفه کشف و گروهبندی اسنادی را دارد که رویدادی یکسان را شرح میدهد و با ارایه یک ساختار قابل درک از گزارشهای خبری، هدایت بهتر کاربران در فضاهای خبری را تسهیل میکند. با رشد سریع و روزافزون اخبار برخط، نیاز به ایجاد موتورهای جستجو برای بازیابی رویدادهای خبری به منظور تسهیل جستجوی کاربران در این فضاهای خبری بیش از پیش احساس میشود. فرض اصلی تشخیص رویداد بر این است که به احتمال زیاد کلمات مرتبط به یک رویداد یکسان در دنیای واقعی، در اسناد و پنجرههای زمانی مشابه ظاهر میشوند. بر همین اساس ما در این تحقیق روشی گذشتهنگر و ویژگیمحور پیشنهاد میکنیم که کلمات را بر اساس ویژگیهای معنایی و زمانی گروهبندی میکند. سپس از این کلمات برای تولید یک بازه زمانی و توصیف متنی قابل درک برای انسان استفاده میکنیم. ارایه یک معماری مناسب و استفاده موثر از خوشهبندی جهت بازیابی رویدادها و همچنین تشخیص مناسب زمان رویداد، از نوآوریهای این پژوهش به شمار میروند. روش پیشنهادی روی مجموعه داده AllTheNews که تقریبا شامل دویست هزار مقاله از 15 منبع خبری در سال 2016 میباشد ارزیابی شده و با روشهای دیگر مقایسه گردیده است. ارزیابیها نشان میدهد که روش پیشنهادی در دو معیار دقت و یادآوری نسبت به روشهای پیشین عملکرد بهتری دارد.
کلیدواژگان: تشخیص رویداد، موتور جستجو، بازیابی اطلاعات، متن کاوی
-
Pages 153-169
Opinion mining as a sub domain of data mining is highly dependent on natural language processing filed. Due to the emerging role of e-commerce, opinion mining becomes one of the interesting fields of study in information retrieval scope. This domain focuses on various sub areas such as polarity detection, aspect elicitation and spam opinion detection. Although there is an internal dependency among these sub sets, but designing a thorough framework including all of the mentioned areas is a highly demanding and challenging task. Most of the literatures in this area have been conducted on English language and focused on one orbit with a binary outcome for polarity detection. Although the employment of supervised learning approaches is among the common utilizations in this area, but the application of deep neural networks has been concentrated with various objectives in recent years so far. Since the absence of a trustworthy and a complete framework with special focuses on each impacting sub domains is highly observed in opinion mining, hence this paper concentrates on this matter. So, through the usage of opinion mining and natural language processing approaches on Persian language, the deep neural network-based framework called RSAD that was previously suggested and developed by the authors of this paper is optimized here to include the binary and numeric polarity detection output of sentences on aspect level. Our evaluation on RSAD performance in comparison with other approaches proves its robustness.
Keywords: Deep neural networks, natural language processing, opinion mining, sentence polarity detection, sentiment Analysis, spam opinion detection -
Pages 170-182
Nowadays, office, residential, and historic buildings often require special monitoring. Obviously, such monitoring involves costs, errors and challenges. As a result of factors such as lower cost, broader application, and ease of installation, wireless sensor networks are frequently replacing wired sensor networks for structural health monitoring. Depending on the type and condition of a structure, factors such as energy consumption and accuracy, as well as fault tolerance are important. Particularly when wireless sensor networks are involved, these are ongoing challenges which, despite research, have the possibility of being improved. Using the Markov decision process and wake-up sensors, this paper proposes an innovative approach to monitoring stable and semi-stable structures, reducing the associated cost and error over existing methods, and according to the problem, we have advantages both in implementation and execution. Thus, the proposed method uses the Markov decision process and wake-up sensors to provide a new and more efficient technique than existing methods in order to monitor the health of stable and semi-stable structures. This approach is described in six steps and compared to widely used methods, which were tested and simulated in CupCarbon simulation environment with different metrics, and shows that the proposed solution is better than similar solutions in terms of a reduction of energy consumption from 11 to 70%, fault tolerance in the transferring of messages from 10 to 80%, and a reduction of cost from 93 to 97%.
Keywords: Structural health monitoring, wireless sensor networks, Markov decision making process, wake-up sensors -
Pages 183-192
Support Vector machine is one of the most popular and efficient algorithms in machine learning. There are several versions of this algorithm, the latest of which is the fuzzy least squares twin support vector machines. On the other hand, in many machine learning applications input data is continuously generated, which has made many traditional algorithms inefficient to deal with them. In this paper, for the first time, an incremental version of the fuzzy least squares twin support vector algorithm is presented. The proposed algorithmis represented in both online and quasi-online modes. To evaluate the accuracy and precision of the proposed algorithmfirst we run our algorithm on 6 datasets of the UCI repository. Results showthe proposed algorithm is more efficient than other algorithms (even non-incremental versions). In the second phase in the experiments, we consider an application of Internet of Things, and in particular in data related to daily activities which inherently are incremental. According to experimental results, the proposed algorithm has the best performance compared to other incremental algorithms.
Keywords: Incremental learning, SVM, fuzzy classification, FLSTSVM -
Pages 193-202
With the rapid development of new technologies in the field of internet of things (IoT) and intelligent networks, researchers are more interested than ever in the concept of wireless sensor networks (WSNs). The emergence of these densely structured networks in recent years has raised the importance of the use of telecommunications technologies, such as ultra-wideband (UWB) technology with high reliability, industrial applications, and appropriate communication security. However, there are still numerous concerns about the extent of inter-network interference, particularly owing to undesired spectral discrete lines in this technology. As a result, it is necessary to provide an optimal solution to eliminate interference and control the power spectrum, and then design the optimal transmitter-receiver structures while considering high sensitivities to the synchronization problem in WSNs based on UWB technology. These goals are pursued in the present study by employing the optimal spectral strategy in the signal model, the structure of the transmitter sensor, and then constructing the optimal or sub-optimal receiver sensor structures, the results of which indicate improved communication performance in WSNs.
Keywords: Sensor networks, Internet of things, inter-network interference, transmitter-receiver structure -
Pages 203-212
The efficiency of human action recognition systems depends on extracting appropriate representations from the video data. In recent years, deep learning methods have been proposed to extract efficient spatial-temporal representations. Deep learning methods, on the other hand, have a high computational complexity for development over temporal domain. Challenges such as the sparsity and limitation of discriminative data, and highly noise factors increase the computational complexity of representing human actions. Therefore, creating a high accurate representation requires a very high computational cost. In this paper, spatial and temporal deep learning networks have been enhanced by adding appropriate feature selection mechanisms to reduce the search space. In this regard, non-online and online feature selection mechanisms have been studied to identify human actions with less computational complexity and higher accuracy. The results showed that the non-linear feature selection mechanism leads to a significant reduction in computational complexity and the online feature selection mechanism increases the accuracy while controlling the computational complexity.
Keywords: Human action recognition, deep learning, spatial-temporal, computational complexity, feature selection mechanism -
Pages 213-220
The efficiency of human action recognition systems depends on extracting appropriate representations from the video data. In recent years, deep learning methods have been proposed to extract efficient spatial-temporal representations. Deep learning methods, on the other hand, have a high computational complexity for development over temporal domain. Challenges such as the sparsity and limitation of discriminative data, and highly noise factors increase the computational complexity of representing human actions. Therefore, creating a high accurate representation requires a very high computational cost. In this paper, spatial and temporal deep learning networks have been enhanced by adding appropriate feature selection mechanisms to reduce the search space. In this regard, non-online and online feature selection mechanisms have been studied to identify human actions with less computational complexity and higher accuracy. The results showed that the non-linear feature selection mechanism leads to a significant reduction in computational complexity and the online feature selection mechanism increases the accuracy while controlling the computational complexity.
Keywords: Human action recognition, deep learning, spatial-temporal, computational complexity, feature selection mechanism -
Pages 221-227
Analysis of published news content is one of the most important issues in information retrieval. Much research has been conducted to analyze individual news articles, while most news events in the media are published in the form of several related articles. Event detection is the task of discovering and grouping documents that describe the same event. It also facilitates better navigation of users in news spaces by presenting an understandable structure of news events. With rapid and increasing growth of online news, the need for search engines to retrieve news events is felt more than ever. The main assumption of event detection is that the words associated with an event appear in the same time windows and similar documents. Accordingly, in this research, we propose a retrospective and feature-pivot method that clusters words into groups according to semantic and temporal features. We then use these words to produce a time frame and a human readable text description. The proposed method is evaluated on the All The News dataset, which consists of two hundred thousand articles from 15 news sources in 2016 and compared to other methods. The evaluation shows that the proposed method outperforms previous methods in terms of precision and recall.
Keywords: Event detection, search engine, information retrieval, text mining