فهرست مطالب
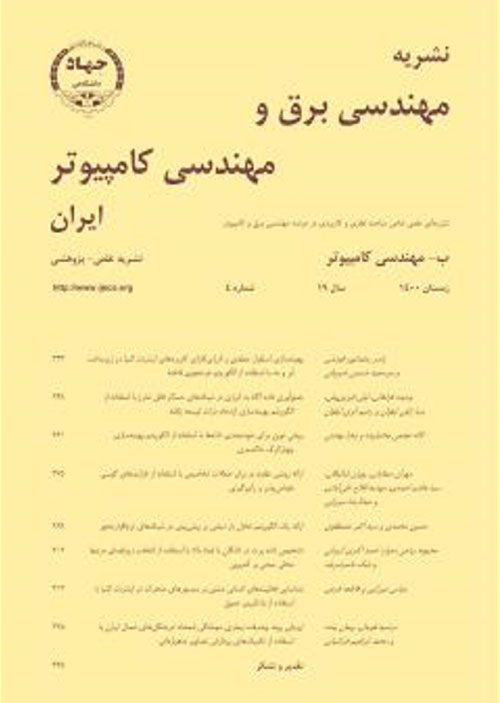
نشریه مهندسی برق و مهندسی کامپیوتر ایران
سال نوزدهم شماره 4 (پیاپی 67، زمستان 1400)
- ب - مهندسی کامپپوتر
- تاریخ انتشار: 1401/01/27
- تعداد عناوین: 8
-
-
صفحات 233-247
استقرار کاربردهای اینترنت اشیا در زیرساخت مه به عنوان مکمل ابر به طور موثری باعث صرفهجویی در استفاده از منابع محاسباتی در زیرساخت ابر میشود. تلاشهای تحقیقاتی اخیر در حال بررسی چگونگی بهرهبرداری بهتر از قابلیتهای مه برای اجرا و پشتیبانی از کاربردهای اینترنت اشیا است. استقرار ناکارامد مولفههای کاربردها در مه منجر به اتلاف منابع، پهنای باند و افزایش مصرف انرژی میشود. همچنین توزیع مولفههای یک کاربرد روی تعداد حداقل ممکن از گرههای مه به منظور کاهش مصرف انرژی منجر به کاهش سطح قابلیت اطمینان خدمات میشود. در این مقاله یک الگوریتم فراابتکاری ترکیبی بر مبنای الگوریتم جستجوی فاخته برای استقرار ایستای مولفههای کاربرد روی زیرساخت مه با هدف مصالحه بین مصرف بهینه انرژی و کاهش اثر نقطه تکی شکست و تقویت قابلیت اطمینان کاربرد در برابر خرابی ارایه میشود. نتایج شبیهسازی نشان میدهد که روش ارایهشده در این مقاله، مصرف انرژی در شبکه مه را کاهش داده و نیازمندیهای کیفیت خدمات کاربرد اینترنت اشیا را با قابلیت اطمینان بالا تامین میکند.
کلیدواژگان: استقرار مطمئن، انرژی کارا، رایانش مه و ابر، کاربردهای اینترنت اشیا، کاربردهای توزیع شده -
صفحات 248-260
یک چالش مهم در شبکههای حسگر، جمعآوری داده با توجه به انرژی محدود گرهها است. استفاده از حسگرهای قابل شارژ برای جمعآوری اطلاعات و انتقال آنها به چاهک، مشکل محدودیت انرژی را تا حدی مرتفع مینماید. با توجه به نرخ پایین برداشت انرژی در گرههای قابل شارژ، مدیریت مصرف انرژی در این شبکهها امری ضروری است. الگوریتمهای موجود، جنبههای مهم جمعآوری آگاه به انرژی- شامل زمانبندی خواب گرهها، خوشهبندی و مسیریابی- را به صورت جامع بررسی نکردهاند و همچنین اکثر آنها از روشهای حریصانه و با کارایی پایین استفاده نمودهاند. در این مقاله، یک روش کارای مبتنی بر الگوریتم بهینهسازی ازدحام ذرات توسعهیافته به نام EDGR برای جمعآوری داده در شبکههای قابل شارژ ارایه شده است. در الگوریتم پیشنهادی، مسئله مورد نظر به سه مرحله زمانبندی خواب گرهها، خوشهبندی و مسیریابی، تقسیم گردیده و مراحل به ترتیب حل شدهاند. بر اساس نتایج شبیهسازی، الگوریتم EDGR مقدار متوسط و انحراف از معیار انرژی ذخیرهشده در گرهها و همچنین نرخ گمشدن بستهها را به مقدار قابل توجهی نسبت به روشهای پیشین بهبود داده است.
کلیدواژگان: الگوریتم بهینه سازی ازدحام ذرات، خوشه بندی، زمان بندی خواب گره ها، شبکه حسگر قابل شارژ، مسیریابی -
صفحات 261-274
امروزه، خوشهبندی دادهها به دلیل حجم و تنوع دادهها بسیار مورد توجه قرار گرفته است. مشکل اصلی روشهای خوشهبندهای معمول این است که در دام بهینه محلی گرفتار میآیند. الگوریتمهای فراابتکاری به دلیل داشتن توانایی فرار از بهینههای محلی، نتایج موفقی را در خوشهبندی دادهها نشان دادهاند. الگوریتم بهینهسازی گرگ خاکستری از جمله این دسته الگوریتمها است که قابلیت بهرهبرداری خوبی دارد و در برخی از مسایل راه حل مناسبی ارایه داده است، اما اکتشاف آن ضعیف است و در بعضی از مسایل به بهینه محلی همگرا میشود. در این تحقیق برای بهبود خوشهبندی دادهها، نسخه بهبودیافتهای از الگوریتم بهینهسازی گرگ خاکستری به نام الگوریتم بهینهسازی چهارگرگ خاکستری ارایه شده که با استفاده از بهترین موقعیت دسته چهارم گرگها به نام گرگهای امگای پیشرو در تغییر موقعیت هر گرگ، قابلیت اکتشاف بهبود مییابد. با محاسبه امتیاز هر گرگ نسبت به بهترین راه حل، نحوه حرکت آن مشخص میشود. نتایج الگوریتم پیشنهادی چهارگرگ خاکستری با الگوریتمهای بهینهسازی گرگ خاکستری، بهینهسازی ازدحام ذرات، کلونی زنبور عسل مصنوعی، ارگانیسمهای همزیست و بهینهسازی ازدحام سالپ در مساله خوشهبندی روی چهارده مجموعه دادگان ارزیابی شده است. همچنین عملکرد الگوریتم پیشنهادی با چند نسخه بهبودیافته از الگوریتم گرگ خاکستری مقایسه شده است. نتایج به دست آمده عملکرد قابل توجه الگوریتم پیشنهادی را نسبت به سایر الگوریتمهای فراابتکاری مورد مقایسه در مساله خوشهبندی نشان میدهد. بر اساس میانگین معیار F روی تمام مجموعه دادگان، روش پیشنهادی 82/172% و الگوریتم بهینه ذرات 78/284% را نشان میدهد و در مقایسه با نسخههای بهبودیافته الگوریتم گرگ، الگوریتم EGWO که در رتبه بعدی است دارای میانگین معیار F برابر 80/656% میباشد.
کلیدواژگان: الگوریتم های فراابتکاری، الگوریتم بهینه سازی گرگ خاکستری، الگوریتم بهینه سازی چهارگرگ، خوشه بندی -
صفحات 275-288
در سالهای اخیر، مسیلهای تحت عنوان آسیبپذیری مدلهای مبتنی بر یادگیری ماشین مطرح گردیده است که نشان میدهد مدلهای یادگیری در مواجهه با آسیبپذیریها از مقاومت بالایی برخوردار نیستند. یکی از معروفترین آسیبها یا به بیان دیگر حملات، تزریق مثالهای تخاصمی به مدل میباشد که در این مورد، شبکههای عصبی و به ویژه شبکههای عصبی عمیق بیشترین میزان آسیبپذیری را دارند. مثالهای تخاصمی، از طریق افزودهشدن اندکی نویز هدفمند به مثالهای اصلی تولید میشوند، به طوری که از منظر کاربر انسانی تغییر محسوسی در دادهها مشاهده نمیشود اما مدلهای یادگیری ماشینی در دستهبندی دادهها به اشتباه میافتند. یکی از روشهای موفق جهت مدلکردن عدم قطعیت در دادهها، فرایندهای گوسی هستند که چندان در زمینه مثالهای تخاصمی مورد توجه قرار نگرفتهاند. یک دلیل این امر میتواند حجم محاسباتی بالای این روشها باشد که کاربردشان در مسایل واقعی را محدود میکند. در این مقاله از یک مدل فرایند گوسی مقیاسپذیر مبتنی بر ویژگیهای تصادفی بهره گرفته شده است. این مدل علاوه بر داشتن قابلیتهای فرایندهای گوسی از جهت مدلکردن مناسب عدم قطعیت در دادهها، از نظر حجم محاسبات هم مدل مطلوبی است. سپس یک فرایند مبتنی بر رایگیری جهت مقابله با مثالهای تخاصمی ارایه میگردد. همچنین روشی به نام تعیین ارتباط خودکار به منظور اعمال وزن بیشتر به نقاط دارای اهمیت تصاویر و اعمال آن در تابع هسته فرایند گوسی پیشنهاد میگردد. در بخش نتایج نشان داده شده که مدل پیشنهادشده عملکرد بسیار مطلوبی در مقابله با حمله علامت گرادیان سریع نسبت به روشهای رقیب دارد.
کلیدواژگان: شبکه های عصبی، فرایندهای گوسی، فرایندهای گوسی مقیاس پذیر، مثال های تخاصمی -
صفحات 289-301
شبکههای نرمافزارمحور یک معماری جدید در شبکه است که لایه کنترل را از لایه داده جدا میسازد. در این رویکرد مسیولیت لایه کنترل به نرمافزار کنترلر واگذار میشود تا رفتار کل شبکه را به طور پویا تعیین نماید. نتیجه این امر، ایجاد یک شبکه بسیار منعطف با مدیریت متمرکز است که در آن میتوان پارامترهای شبکه را به خوبی کنترل کرد. با توجه به افزایش روزافزون کاربران، ظهور فناوریهای جدید، رشد انفجاری ترافیک در شبکه، برآوردهسازی الزامات کیفیت خدمات و جلوگیری از کمباری یا پرباری منابع، تعادل بار در شبکههای نرمافزارمحور ضروری میباشد. عدم تعادل بار باعث بالارفتن هزینه، کاهش مقیاسپذیری، انعطافپذیری، بهرهوری و تاخیر در سرویسدهی شبکه میشود. تا کنون الگوریتمهای مختلفی برای بهبود عملکرد و تعادل بار در شبکه ارایه شدهاند که معیارهای متفاوتی مانند انرژی مصرفی و زمان پاسخ سرور را مد نظر قرار دادهاند، اما اغلب آنها از ورود سیستم به حالت عدم تعادل بار جلوگیری نمیکنند و خطرات ناشی از عدم تعادل بار را کاهش نمیدهند. در این مقاله، یک روش تعادل بار مبتنی بر پیشبینی برای جلوگیری از ورود سیستم به حالت عدم تعادل بار با بهرهگیری از الگوریتم ماشین یادگیری افراطی پیشنهاد میشود. نتایج ارزیابی روش پیشنهادی نشان میدهد که از نظر تاخیر پردازش کنترلکننده، میزان تعادل بار و زمان پاسخگویی به علت تعادل بار بهینه نسبت به روشهای CDAA و PSOAP عملکرد بهتری دارد.
کلیدواژگان: شبکه های نرم افزارمحور، توازن بار، الگوریتم های پیش بینی، ماشین یادگیری افراطی -
صفحات 302-312
یکی از چالشهای مسئله تشخیص داده پرت با ابعاد بالا، طلسم بعد است که در آن برخی ابعاد (ویژگیها) منجر به پنهانشدن دادههای پرت میگردند. برای حل این مسیله، ابعادی که حاوی اطلاعات ارزشمندی در دادگان با ابعاد بالا جهت تشخیص داده پرت هستند، جستجو میشوند تا با نگاشت دادگان به زیرفضای متشکل از این ابعاد مرتبط، دادههای پرت برجستهتر و قابل شناسایی شوند. این مقاله با معرفی یک روش جدید انتخاب زیرفضای مرتبط محلی و توسعه یک رویکرد امتیازدهی داده پرت مبتنی بر چگالی محلی، امکان تشخیص داده پرت در دادگان با ابعاد بالا را فراهم مینماید. در ابتدا، یک الگوریتم برای انتخاب زیرفضای مرتبط محلی بر اساس آنتروپی محلی ارایه میشود تا بتواند برای هر نقطه داده با توجه به دادههای همسایهاش یک زیرفضای مرتبط انتخاب کند. سپس هر نقطه داده در زیرفضای انتخابی متناظرش با یک روش امتیازدهی پرت محلی مبتنی بر چگالی امتیازدهی میشود، به طوری که با در نظر گرفتن یک پهنای باند تطبیقی جهت تخمین چگالی هسته سعی میشود که اختلاف جزیی بین چگالی یک نقطه داده نرمال با همسایههایش از بین رفته و به اشتباه به عنوان داده پرت تشخیص داده نشود و در عین حال، تخمین کمتر از مقدار واقعی چگالی در نقاط داده پرت، منجر به برجستهشدن این نقاط داده گردد. در پایان با آزمایشهای تجربی روی چندین دادگان دنیای واقعی، الگوریتم پیشنهادی تشخیص داده پرت زیرفضای مبتنی بر آنتروپی محلی با چند تکنیک تشخیص داده پرت بر حسب دقت تشخیص مقایسه شده است. نتایج تجربی نشان میدهد که الگوریتم پیشنهادی مبتنی بر معیار آنتروپی محلی و روش پیشنهادی امتیازدهی داده پرت توانسته است به دقت بالایی جهت تشخیص داده پرت دست یابند.
کلیدواژگان: تشخیص داده پرت، داده های با ابعاد بالا، انتخاب زیرفضای مرتبط محلی، آنتروپی محلی -
صفحات 313-324
کنترل محدودهها، اماکن و سنسورهای حرکتی در اینترنت اشیا نیازمند کنترل پیوسته و مستمر برای تشخیص فعالیتهای انسانی در شرایط مختلف است که این مهم، خود چالشی از جمله نیروی انسانی و خطای انسانی را نیز در بر دارد. کنترل همیشگی توسط انسان نیز بر سنسورهای حرکتی اینترنت اشیا غیر ممکن به نظر میرسد. اینترنت اشیا فراتر از برقراری یک ارتباط ساده بین دستگاهها و سیستمها میباشد. اطلاعات سنسورها و سیستمهای اینترنت اشیا به شرکتها کمک میکند تا دید بهتری نسبت به کارایی سیستم داشته باشند. در این پژوهش روشی مبتنی بر یادگیری عمیق و شبکه عصبی عمیق سیلایهای برای تشخیص فعالیتهای انسانی روی مجموعه داده تشخیص فعالیت دانشگاه فوردهام ارایه شده است. این مجموعه داده دارای بیش از یک میلیون سطر در شش کلاس برای تشخیص فعالیت در اینترنت اشیا است. بر اساس نتایج به دست آمده، مدل پیشنهادی ما در راستای تشخیص فعالیتهای انسانی در معیارهای ارزیابی مورد نظر کارایی 90 درصد و میزان خطای 2/2 درصد را داشت. نتایج به دست آمده نشان از عملکرد خوب و مناسب یادگیری عمیق در تشخیص فعالیت است.
کلیدواژگان: تشخیص فعالیت انسانی، یادگیری عمیق، یادگیری ماشین، شبکه عصبی عمیق، اینترنت اشیا -
صفحات 325-334
در چند سال اخیر، بیماری سوختگی شمشاد به یکی از مهمترین نگرانیهای مدیران منابع طبیعی کشور و دوستداران محیط زیست تبدیل شده است. به منظور کاهش خطر انقراض این گونه، نیاز به تشخیص زودهنگام و تهیه نقشه پراکنش بیماری است و در این راستا، دادههای سنجش از دور میتوانند نقش مهمی را ایفا کنند. در این پژوهش برای بررسی میزان تخریب از ادغام تصاویر پانکروماتیک با قدرت تفکیک مکانی بالا و چندطیفی با قدرت تفکیک مکانی پایین استفاده گردیده و همچنین به طور همزمان در تصاویر استخراجشده از ماهواره لندست 8، ویژگیهای طیفی و بافتی مورد توجه قرار گرفته و در نهایت با استخراج ویژگیهای موثر از فضای توصیف کاندیدا با کمک الگوریتم ژنتیک و به کارگیری طبقهبند مناسب در قالب به کارگیری همزمان خوشهبندی فازی و طبقهبندی بیشینه شباهت، کلاس پوشش منطقه با دقت مطلوبی بین سالهای 2014 تا 2018 استخراج نهایی شده است. نتایج ارزیابی و ضریب تبیین مدلها، اعتبارسنجی روش را در برآوردهای آینده مورد تایید قرار میدهد.
کلیدواژگان: پردازش تصاویر ماهواره ای، شاخص های گیاهی، لندست 8، سوختگی، شمشاد خزری
-
Pages 233-247
Deployment applications of internet of things (IoT) in fog infrastructure as cloud complementary leads effectively computing resource saving in cloud infrastructure. Recent research efforts are investigating on how to better exploit fog capabilities for execution and supporting IoT applications. Also, the distribution of an application’s components on the possible minimum number of fog nodes for the sake of reduction in power consumption leads degradation of the service reliability level. In this paper, a hybrid meta-heuristic algorithm based on cuckoo search algorithm is presented for static deployment the components of IoT applications on fog infrastructure in the aim of trade-off between efficient power usage, reduction in the effect of one point of failure and boosting the application reliability against failure. The results of simulations show that the proposed approach in this paper reduces the power consumption of fog network and meets the quality of service requirement of IoT application with the high reliability level.
Keywords: Reliable deployment, energy-efficient, fog, cloud computing, IoT applications, distributed applications -
Pages 248-260
This paper investigates the problem of data gathering in rechargeable Wireless Sensor Networks (WSNs). The low energy harvesting rate of rechargeable nodes necessitates effective energy management in these networks. The existing schemes did not comprehensively examine the important aspects of energy-aware data gathering including sleep scheduling, and energy-aware clustering and routing. Additionally, most of them proposed greedy algorithms with poor performance. As a result, nodes run out of energy intermittently and temporary disconnections occur throughout the network. In this paper, we propose an energy-efficient data gathering algorithm namely Energy-aware Data Gathering in Rechargeable wireless sensor networks (EDGR). The proposed algorithm divides the original problem into three phases namely sleep scheduling, clustering, and routing, and solves them successively using particle swarm optimization algorithm. As derived from the simulation results, the EDGR algorithm improves the average and standard deviation of the energy stored in the nodes by 17% and 5.6 times, respectively, compared to the previous methods. Also, the packet loss ratio and energy consumption for delivering data to the sink of this scheme is very small and almost zero
Keywords: Particle swarm optimization, clustering, sleep scheduling, rechargeable sensor network, routing -
Pages 261-274
Nowadays, clustering methods have received much attention because the volume and variety of data are increasing considerably.The main problem of classical clustering methods is that they easily fall into local optima. Meta-heuristic algorithms have shown good results in data clustering. They can search the problem space to find appropriate cluster centers. One of these algorithms is gray optimization wolf (GWO) algorithm. The GWO algorithm shows a good exploitation and obtains good solutions in some problems, but its disadvantage is poor exploration. As a result, the algorithm converges to local optima in some problems. In this study, an improved version of gray optimization wolf (GWO) algorithm called 4-gray wolf optimization (4GWO) algorithm is proposed for data clustering. In 4GWO, the exploration capability of GWO is improved, using the best position of the fourth group of wolves called scout omega wolves. The movement of each wolf is calculated based on its score. The better score is closer to the best solution and vice versa. The performance of 4GWO algorithm for the data clustering (4GWO-C) is compared with GWO, particle swarm optimization (PSO), artificial bee colony (ABC), symbiotic organisms search (SOS) and salp swarm algorithm (SSA) on fourteen datasets. Also, the efficiency of 4GWO-C is compared with several various GWO algorithms on these datasets. The results show a significant improvement of the proposed algorithm compared with other algorithms. Also, EGWO as an Improved GWO has the second rank among the different versions of GWO algorithms. The average of F-measure obtained by 4GWO-C is 82.172%; while, PSO-C as the second best algorithm provides 78.284% on all datasets.
Keywords: Data mining, data clustering, meta-heuristic algorithm, gray wolf optimization (GWO) algorithm, 4-gray wolf optimization (4GWO) algorithm, F-measure -
Pages 275-288
In recent years, the issue of vulnerability of machine learning-based models has been raised, which shows that learning models do not have high robustness in the face of vulnerabilities. One of the most well-known defects, or in other words attacks, is the injection of adversarial examples into the model, in which case, neural networks, especially deep neural networks, are the most vulnerable. Adversarial examples are generated by adding a little purposeful noise to the original examples so that from the human user's point of view there is no noticeable change in the data, but machine learning models make mistakes in categorizing the data. One of the most successful methods for modeling data uncertainty is Gaussian processes, which have not received much attention in the field of adversarial examples. One reason for this could be the high computational volume of these methods, which limits their used in the real issues. In this paper, a scalable Gaussian process model based on random features has been used. This model, in addition to having the capabilities of Gaussian processes for proper modeling of data uncertainty, is also a desirable model in terms of computational cost. A voting-based process is then presented to deal with adversarial examples. Also, a method called automatic relevant determination is proposed to weight the important points of the images and apply them to the kernel function of the Gaussian process. In the results section, it is shown that the proposed model has a very good performance against fast gradient sign attack compared to competing methods.
Keywords: Neural networks, Gaussian process, scalable Gaussian process, adversarial examples -
Pages 289-301
Software-defined networking is a new network architecture which separates the control layer from the data layer. In this approach, the responsibility of the control layer is delegated to the controller software to dynamically determine the behavior of the entire network. It results in a flexible network with centralized management in which network parameters can be well controlled. Due to the increasing number of users, the emergence of new technologies, the explosive growth of network traffic, meeting the requirements of quality of service and preventing underload or overload of resources, load balancing in software-based networks is of substantial importance. Load imbalance increases costs, reduces scalability, flexibility, efficiency, and delay in network service. So far, a number of solutions have been proposed to improve the performance and load balancing in the network, which take into account different criteria such as power consumption and server response time, but most of them do not prevent the system from entering the load imbalance mode and the risks of load imbalance. In this paper, a predictive load balancing method is proposed to prevent the system from entering the load imbalance mode using the Extreme Learning Machine (ELM) algorithm. The evaluation results of the proposed method show that in terms of controller processing delay, load balance and response time, it performs better than CDAA and PSOAP methods.
Keywords: Software-defined networking, load balancing, prediction algorithm, extreme learning machine (ELM) -
Pages 302-312
One of the challenges of high dimensional outlier detection problem is the curse of dimensionality which irrelevant dimensions (features) lead to hidden outliers. To solve this problem, some dimensions that contain valuable information to detect outliers are searched to make outliers more prominent and detectable by mapping the dataset into the subspace which is constituted of these relevant dimensions/features. This paper proposes an outlier detection method in high dimensional data by introducing a new locally relevant subspace selection and developing a local density-based outlier scoring. First, we present a locally relevant subspace selection method based on local entropy to select a relevant subspace for each data point due to its neighbors. Then, each data point is scored in its relevant subspace using a density-based local outlier scoring method. Our adaptive-bandwidth kernel density estimation method eliminates the slight difference between the density of a normal data point and its neighbors. Thus, normal data are not wrongly detected as outliers. At the same time, our method underestimates the actual density of outlier data points to make them more prominent. The experimental results on several real datasets show that our local entropy-based subspace selection algorithm and the proposed outlier scoring can achieve a high accuracy detection rate for the outlier data.
Keywords: Outlier detection, high dimensional data, locally relevant subspace selection, local entropy -
Pages 313-324
Control of areas and locations and motion sensors in the Internet of Things requires continuous control to detect human activities in different situations, which is an important challenge, including manpower and human error. Permanent human control of IoT motion sensors also seems impossible. The IoT is more than just a simple connection between devices and systems. IoT information sensors and systems help companies get a better view of system performance. This study presents a method based on deep learning and a 30-layer DNN neural network for detecting human activity on the Fordham University Activity Diagnostic Data Set. The data set contains more than 1 million lines in six classes to detect IoT activity. The proposed model had almost 90% and an error rate of 0.22 in the evaluation criteria, which indicates the good performance of deep learning in activity recognition.
Keywords: Human activity detection, deep learning, machine learning, DNN, IoT -
Pages 325-334
In recent years, boxwood dieback has become one of the essential concerns of practitioners and managers of the natural resources of the country. To control the expansion of the factors contributing to the dieback of box trees, the early detection and preparation of distribution maps are required. Assessment data can play an important role in this regard. The combination of high-resolution and low-spectrum panchromatic images with low resolution is used for evaluating the extent of destruction. Also, spectral and textural features are considered simultaneously in images extracted from Landsat 8 satellite. Finally, by extracting effective features from the candidate description space with the help of genetic algorithm and using the appropriate classification in the form of simultaneous application of fuzzy clustering and maximum similarity classification of area resulted in good accuracy in 2014-2018. The coefficients obtained from the models confirm their model validation for future estimates and the possibility it usage to assess the extent of the affected areas and the evolution of progress for all regions.
Keywords: Satellite image processing, vegetation indices, Landsat 8, dieback