فهرست مطالب
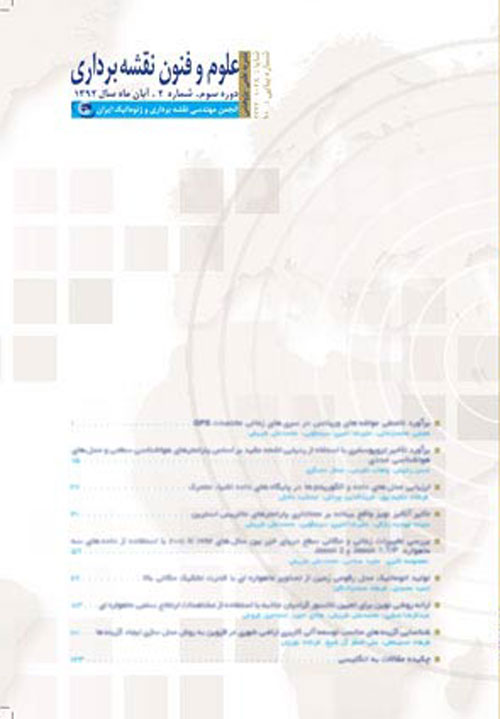
نشریه علوم و فنون نقشه برداری
سال یازدهم شماره 4 (پیاپی 44، بهار 1401)
- تاریخ انتشار: 1401/04/28
- تعداد عناوین: 11
-
-
صفحات 1-10
شمارش گل های ذرت اطلاعات ارزشمندی در مورد پیش بینی میزان محصول ارایه می دهد، اما شمارش گل های ذرت به روش سنتی و دستی امری گران و زمان بر می باشد. در این مقاله روش پیشنهادی مبتنی بر جداسازی مکانی آگاه از وظیفه (TSD) برای بهبود عملکرد تشخیص گل های ذرت اصلاح شده است. تصاویر رنگی با هم پوشانی، از مزرعه گل های ذرت توسط پهپاد اخذ شده است و سپس با اعمال پیش پردازش های لازم ارتوفوتو مزرعه تشکیل می گردد، در مرحله بعد آشکارساز TSD اصلاح شده جهت تعیین محل های گل های ذرت بر روی ارتوفوتو اعمال می گردد. سپس نتایج به دست آمده از کار نوین انجام شده با نتایج حاصل از کار تیم متخصص کشاورزی و آشکارسازهای معتبر در این زمینه مانند CenterNet و RepPoints مقایسه شده و دقت بالایی حاصل گردیده است.
کلیدواژگان: یادگیری عمیق، پهپاد، تشخیص و شمارش گل ذرت، اشیاء کوچک -
صفحات 11-23
تهیه نقشه پوشش اراضی شهری یکی از پیش شرط های اساسی بسیاری از برنامه های شهری محسوب می شود. صرف کمترین زمان و هزینه برای تهیه این نقشه ها از جمله چالش های مدیران شهری محسوب می شود. امروزه تصاویر ماهواره ای و طبقه بندی آن ها کاربرد گسترده ای در تهیه نقشه های پوشش اراضی شهری پیدا کرده اند. بر این اساس هدف از این تحقیق، تهیه نقشه پوشش اراضی شهری در شهر تبریز با استفاده از تصویر ماهواره ای سنتینل-2 می باشد. برای طبقه بندی تصویر ماهواره ای، از دو الگوریتم جنگل تصادفی و یادگیری عمیق مبتنی بر شبکه عصبی (شبکه عصبی عمیق) استفاده شد. کلاس های کاربری مورد نظر شامل پنج پوشش سطحی: اراضی بایر، مناطق ساخته شده، راه، پوشش گیاهی و آب بودند. تمام مراحل پردازش تصاویر ماهواره ای به صورت خودکار و در سامانه های پردازش ابری Google Earth Engine و Google Colab انجام شد. با توجه به نتایج به دست آمده، الگوریتم شبکه عصبی عمیق با صحت کلی 95/2 درصد عملکرد بهتری را نسبت به الگوریتم جنگل تصادفی با صحت کلی 93/1 درصد ارایه کرد. بررسی عملکرد این دو الگوریتم در استخراج هر یک از کلاس ها نشان داد که روش شبکه عصبی عمیق در استخراج کلاس های اراضی بایر و مناطق ساخته شده عملکرد بهتری داشته است، به طوری که مقادیر صحت کاربر و تولید کننده آن در کلاس اراضی بایر به ترتیب 9/6 و 1 درصد بیش تر از الگوریتم جنگل تصادفی بودند. این میزان در کلاس مناطق ساخته شده نیز به ترتیب 0/3 و 4/3 درصد بیش تر از الگوریتم جنگل تصادفی بودند. از طرفی الگوریتم جنگل تصادفی در استخراج کلاس راه عملکرد بهتری داشت و مقادیر صحت کاربر و تولید کننده آن به ترتیب 3/65 و 4/1 درصد بیش از روش شبکه عصبی عمیق بودند. بنابراین می توان گفت هر دو الگوریتم عملکرد مناسبی در تهیه نقشه پوشش شهری ارایه دادند، اما عملکرد کلی الگوریتم شبکه عصبی عمیق، مناسب تر بود.
کلیدواژگان: پوشش اراضی شهری، شبکه عصبی عمیق، جنگل تصادفی، سنتینل-2 -
صفحات 25-37
انتخاب مکان بهینه و مناسب برای اسکان موقت آسیب دیدگان ناشی از سوانح طبیعی نظیر سیل، از دیرباز مورد توجه برنامه ریزان متولیان مدیریت بحران بوده است. در صورت عدم مکانیابی صحیح این مراکز، ممکن است خسارت های سنگینی به دنبال داشته باشد. هدف از این پژوهش، تعیین مکان های مناسب به منظور استقرار مراکز اسکان موقت پس از وقوع سیل در استان مازندران می باشد. برای تحقق این هدف، ابتدا با استفاده از نظرات کارشناسان و تحقیقات گذشته 9 معیار موثر در مکانیابی اسکان موقت استخراج و نقشه های معیار تهیه و نرمال سازی شده است. برای وزن دهی به این معیارها نیز با استفاده از نظرات کارشناسان و تحقیقات گذشته، از روش فرآیند تحلیل سلسله مراتبی (AHP) استفاده شده است. در گام بعد، جهت تلفیق معیارها با توجه به وزن های محاسبه شده از روش AHP، از روش ترکیب خطی وزن دار (WLC) استفاده شده است و نقشهی نهایی در چهار کلاس "بسیار مناسب"، "مناسب"، "نامناسب" و "بسیار نامناسب" طبقه بندی شده است. در نهایت در دو کلاس "بسیار مناسب" و "مناسب" حاصل از مرحله قبل، با استفاده از تابع P_Median در الگوریتم ژنتیک، به مکانیابی مراکز اسکان موقت پس از سیلاب با توجه به نقاط جمعیتی پرداخته شده است. الگوریتم به جستجوی 15 مکان از میان نقاط کاندیدا برای اسکان موقت می پردازد که در نتیجه مراکز در مناطق با جمعیت بالا در دو کلاس مطلوب تر نسبت به سایر کلاس ها مکانیابی شده اند.
کلیدواژگان: اسکان موقت، سیلاب، سیستم اطلاعات جغرافیایی، تحلیل سلسله مراتبی، استان مازندران، p-median -
صفحات 39-53
امروزه با توجه به نیاز روز افزون پیش بینی پارامترهای حرکت قطبی از روش های مختلفی استفاده می شود. وجود مجموعه داده های بلند مدت پارامترهای x و y حرکت قطبی سبب بوجود آمدن منبع ارزشمندی برای پیش بینی این پدیده ی ژیوفیزیکی است. در این پژوهش از داده های بلند مدت حرکت قطبی برای پیش بینی دوره های 40 روزه به مدت 5 سال استفاده شده است. برای انجام پیش بینی ها از شبکه های عصبی کانولوشنی، شبکه حافظه طولانی کوتاه مدت، شبکه پرسپترون چندلایه و روش آنالیز هارمونیک کمترین مربعات استفاده شده است. در ادامه نتایج شبکه های عصبی با یکدیگر و روش آنالیز هارمونیک کمترین مربعات مقایسه گردیده است. در پایان مشخص شد که شبکه های حافظه طولانی کوتاه مدت در بیشتر روزها از شبکه های کانولوشنی و پرسپترون چندلایه نتایج بهتری دارند. همچنین مشخص شد که این روش ها دقت پیش بینی بسیار بهتری نسبت به روش آنالیز هارمونیک کمترین مربعات دارند. برای شبکه های ژرف برای پارامتر x بهترین مقادیر RMSE برای روز های اول و چهلم به ترتیب 49/0 و 05/15 میلی ثانیه کمانی است و برای پارامتر y بهترین مقادیر RMSE برای روز های اول و چهلم به ترتیب 68/0، 22/9 میلی ثانیه کمانی است. در روش آنالیز هارمونیک کمترین مربعات مقدار RMSE برای پارامتر x برای روزهای اول و چهلم به ترتیب برابر با 95/40 و 40/48 میلی ثانیه ی کمانی است و مقدار RMSE پارامتر y برای روزهای اول و چهلم به ترتیب برابر با 86/40 و 53/46 میلی ثانیه ی کمانی است.
کلیدواژگان: سری زمانی، حرکت قطبی، شبکه های عصبی ژرف، LSTM - CNN - MLP -
صفحات 55-65
آلودگی محیط زیست و بلایا با رشد جمعیت به تدریج افزایش یافته است . وجود منابع نفتی در دریاها و حوادث مربوط کشف ، استخراج و حمل و نقل آن ها باعث به وجود آمدن لکه های نفتی در سطح دریاها می شود که نشت این مواد نفتی به دریاها عوارض محیط زیستی جبران ناپذیری دارد . به همین دلیل نظارت بر اثرات این حوادث برای بهداشت عمومی بسیار مهم است . ماموریت های ماهواره ای ابزاری بسیار کارآمد برای شناسایی آلاینده هایی مانند نشت نفت است. سنسور رادار با دیافراگم مصنوعی (SAR) یک سیستم سنجش ماکروویو فعال است که می تواند برای تشخیص نشت نفت با سنسورهای نوری نصب شده روی سیستم های ماهواره ی لندست-8، سنتینل-2و استر با در نظر گرفتن پوشش ابر و زمان بازدید مجدد ماهواره در مکان مشابه استفاده شود. در این مطالعه منطقه نشت نفت ناشی از نشت لوله در جزیره خارک با تصاویر ماهواره ای لندست-8 و سنتینل-1 مورد مطالعه قرار گرفت. تکنیک های مختلف پردازش تصویر به منظور برجسته سازی نشت نفت در رابطه با حادثه رخ داده ، مانند فیلتر مورفولوژی و کانولوشن بر روی باندهای لندست-8 اعمال شد. ما از تصاویر لندست-8 به منظور پشتیبانی از نتایج به دست آمده از سنتینل-1 استفاده کردیم . لکه های نفتی با تجزیه و تحلیل داده SAR و نتایج لندست-8 با موفقیت تشخیص داده شدند و با تفسیر بصری نتایج ، روش های انتخاب شده از نظر نمایش مناطق نشت نفت با یکدیگر تطابق دارند.
کلیدواژگان: لکه نفتی، سنتینل-1، لندست-8، پردازش تصویر -
صفحات 67-82
در سال های اخیر آشکارسازی و استخراج عارضه راه از تصاویر ماهواره ای با پیشرفت و توسعه ی الگوریتم های یادگیری عمیق در بخش تقسیم بندی معنایی بیش ازپیش موردتوجه پژوهشگران قرارگرفته است. در این راستا بیشتر مطالعات انجام شده درزمینه آشکارسازی و شناسایی عارضه راه با استفاده از تصاویر اپتیک بوده و در این میان مطالعات معدودی با استفاده از تصاویر راداری در سطح جهان انجام شده است. لذا هدف این مقاله بهره گیری از یک شبکه عصبی پیچشی باقیمانده عمیق پالایش شده (RDRCNN) به منظور ارزیابی و مقایسه دقت آشکارسازی عارضه راه حاصل از تصاویر راداری سنتینل 1 در کلان شهرهای تهران و شیراز در شرایط برابر ازنظر تعداد نمونه های آموزشی، اعتبارسنجی و معماری یکسان می باشد. در این تحقیق جهت آشکارسازی عارضه راه با استفاده از شبکه های عصبی عمیق (DNN)، از ترکیب رنگی VV-VH تصاویر راداری سنتینل 1 از 8 شهر مختلف (تهران، مشهد، اصفهان، شیراز، تبریز،، ارومیه، بغداد و پکن) بهره گرفته شد. درنهایت برای آموزش و آشکارسازی عارضه راه از مدل RDRCNN با داشتن یک واحد متصل باقی مانده (RCU) و یک واحد ادراک متسع شده (DPU) استفاده شد. یافته های پژوهش حاکی از آن است که مدل RDRCNN در فرایند شناسایی و آشکارسازی راه در دو شهر تهران و شیراز تقریبا یکسان عمل کرده و به طورکلی مدل فوق در شهر شیراز اندکی بهتر عمل نموده است؛ به صورتی که ازنظر متریک های ارزیابی صحت برای تصاویر شهر تهران معیارهای کامل بودن 57.66%، صحت 51.29%، امتیاز F1 54.43% و دقت کلی 92.78% و برای تصاویر شهر شیراز معیارهای کامل بودن 60.77%، صحت 54.71%، امتیاز F1 57.40% و دقت کلی 95.63% به دست آمد. یافته های این پژوهش دقت پایین آموزش و آشکارسازی عارضه راه از تصاویر راداری سنتینل 1 برای دو مورد از کلان شهرهای ایران را نشان می دهد. به طورکلی با مقایسه نتایج حاصل از این پژوهش و مطالعات قبلی می توان به این مورد پی برد که یکی از مهم ترین علل دقت پایین نتایج، کم عرض بودن راه ها در شهرهای ایران می باشد؛ اما به علت فقدان مطالعات لازم در زمینه ی آشکارسازی عارضه راه با تصاویر راداری سنتینل 1، نمی توان با قاطعیت در مورد نتایج آن اظهارنظر کرد و پیشنهاد می شود مطالعات بیشتری در این زمینه انجام گیرد.
کلیدواژگان: یادگیری عمیق، RDRCNN، سنتینل 1، آشکارسازی راه، تهران، شیراز -
صفحات 83-96
شهرهای امروزی مملو از سازه های بزرگ اند که پایش آن ها به روش های سنتی نیازمند هزینه و زمان بسیار بالایی است. سازه های مهندسی موجود بدلیل عوامل مختلف از جمله بارگذاری های پیش بینی نشده و نشست زمین دچار تغییر شکل شده و مشکلاتی را به وجود می آورند. یکی از این ساز ه های مهم که نیازمند پایش مداوم است، سد می باشد؛ چراکه ناپایداری سدهای بزرگ و گسیختگی آن ها عاملی تهدیدکننده برای جان و مال انسان ها به حساب می آید و اثرات زیست محیطی قابل ملاحظه ای به دنبال خواهد داشت. مسیله اصلی در این تحقیق، ارایه روشی کم هزینه، سریع و دقیق برای محاسبه جابجایی و تغییرشکل بدنه سدهای خاکی می باشد. روش های مختلفی جهت اندازه گیری جابجایی بدنه سازه سد وجود دارد که در میان آن ها استفاده از تکنیک های سنجش ازدوری از جمله تداخل سنجی راداری به دلیل فراهم نمودن مشاهدات گسترده و منظم از سطح زمین می تواند در امر اندازه گیری جابجایی و نشست سازه سد مفید واقع شود. منطقه مورد مطالعه در این تحقیق، سد خاکی ماملو واقع در استان تهران می باشد. روش استفاده شده برای برآورد جابجایی بدنه این سد، تکنیک پراکنشگر دایمی (PSI) می باشد، داده های مورد نیاز در این تحقیق، داده های باند C و X سنجنده های Sentinel-1A و CosmoSkyMed-X می باشد. در پردازش سری زمانی این تصاویر از گراف ستاره ای و مثلث بندی دلونی استفاده شده و دو مدل خطی و غیرپارامتریک برای برآورد جابجایی در نظر گرفته شد. در پردازش داده های سنجنده S-1A سد داریان مدل مناسب برای جابجایی ها مدل غیرپارامتریک و برای داده های سنجنده CSK همین سد مدل خطی به عنوان مناسب ترین مدل انتخاب گردید. به دلیل عدم برداشت داده های زمینی از سد ماملو در بازه زمانی مشترک با داده های راداری، به منظور ارزیابی دقت و اثبات درستی نتایج بدست آمده، نتایج دو دسته داده ی راداری CSK و S-1A مربوط به سد ماملو با یکدیگر مقایسه شده و همخوانی بسیار بالایی بین نتایج این دسته داده ملاحظه شد که میزان RMSE محاسبه شده برای داده های ASC این دو سنجنده برابر با 0.7703 میلیمتر و برای داده های DSC برابر با 0.9551 میلیمتر محاسبه گردید که این همخوانی بسیار بالای نتایج، امریست که می تواند دلیلی بر درستی نتایج استخراج شده باشد.
کلیدواژگان: تداخل سنجی راداری، تکنیک پراکنشگر دائم، سدخاکی، Sentinel-1A، CosmoSkyMed، X -
صفحات 107-117
زیرساخت داده مکانی (SDI) Spatial Data Infrastructure مجموعه ای از سیاست ها، استانداردها، شبکه های دسترسی، داده های مکانی، سازمان ها و مردم است که امور مختلف تولید، جمع آوری، ذخیره سازی، دسترسی و استفاده بهینه از داده های مکانی را در حوزه مشخصی تسهیل و هماهنگ می سازد. در راستای پیاده سازی زیرساخت داده مکانی استانی، سازمان مدیریت و برنامه ریزی استان، مسیولیت راهبری و هماهنگی بین دستگاه های اجرایی استان و استقرار ژیوپورتال استانی را بر عهده خواهد داشت. با عنایت به اینکه تاکنون زیرساخت داده مکانی در سطح استانی مورد مطالعه قرار نگرفته، لذا به منظور سنجش و ارزیابی میزان موفقیت پیاده سازی زیرساخت داده مکانی در استان ایلام، ابتدا عوامل و شاخص های موثر در پیاده سازی موفق SDI استانی شناسایی و سپس با استفاده از روش ترکیبی تکنیک دیمتل و فرایند تحلیل شبکه (DANP) وزن دهی شدند، که به ترتیب شاخص های ساختار، منابع مالی، تخصص و آموزش و فرهنگ سازی، بیشترین اهمیت را به خود اختصاص دادند. سپس میزان اجرای هر کدام از معیارها در استان مورد بررسی قرار گرفت. در نهایت میزان موفقیت پیاده سازی زیرساخت داده های مکانی استان ایلام محاسبه گردید. نتایج نشان داد که میزان تحقق SDI در استان ایلام 63 درصد می باشد که با توجه به شرایط موجود می توان عملکرد این استان را در سطح خوب قرار دارد. درنهایت نقاط ضعف در پیاده سازی SDI در استان ایلام، شناسایی و راهکارهای برطرف نمودن آنها و افزایش میزان موفقیت تحقق SDI در این استان بیان گردید.
کلیدواژگان: زیرساخت داده مکانی (SDI)، تکنیک دیمتل و فرآیند تحلیل شبکه سلسله مراتبی (DANP)، استان ایلام -
صفحات 119-130
در سال های اخیر جمعیت شهرنشین در ایران رو به افزایش بوده است. این افزایش جمعیت در شهرهای بزرگ باعث افزایش تقاضا برای زمین می شود. افزایش تقاضا به نوبه خود موجب بلندمرتبه سازی و افزایش تراکم و ایجاد نقشه های ثبتی پرتعداد و پیچیده در این ساختمان ها شده است. در حال حاضر، روش فعلی برای ثبت و ذخیره سازی انواع مرزهای حقوقی در ایران، استفاده از خطوط و نقشه های دوبعدی است. این روش دارای محدودیت هایی برای نمایش مرزهای ثبتی مختلف که فضاهای مالکیتی را مشخص می کنند، است. به همین دلیل ایجاد یک سازوکار جهت ثبت مالکیت های موجود در ساختمان ها به شکلی دقیق و کارا بیش ازپیش موردنیاز است. با پیشرفت درزمینه مدل های سه بعدی مخصوصا مدل سازی اطلاعات ساختمانی[1]، تحقیقات زیادی جهت ثبت سه بعدی مرزهای حقوقی با استفاده از این مدل ها در کشورهای مختلف در حال انجام است. در این مقاله برای اولین بار از مدل سازی اطلاعات ساختمانی جهت ثبت سه بعدی انواع مرزهای حقوقی در حوزه قضایی ایران استفاده شد. برای این کار ابتدا انواع مختلف مرزهای ثبتی در ایران طبق مقررات اداره ثبت اسناد و املاک کشور و دستورالعمل تفکیکی ساختمان ها در ایران مشخص شدند. سپس جهت ثبت این مرزها، استاندارد کلاس های بنیاد صنعت[2] توسعه یافت و بر روی یک نمونه اولیه که ساختمانی در تهران است پیاده سازی شد. در مرحله ارزیابی، مرزهای ثبتی در دو روش پیشنهادی و روش فعلی با استفاده از روش پرسشنامه مقایسه شدند. نتایج این ارزیابی در معیارهای مختلف، قابلیت بالای روش پیشنهادی در ثبت و نمایش مرزهای حقوقی مصوب سازمان ثبت اسناد و املاک کشور را نشان داد، به طوری که بیش از 80 درصد از جامعه نخبگان شرکت کننده در پرسشنامه این روش را نسبت به روش فعلی مناسب تر دانستند.
کلیدواژگان: ثبت املاک، کاداستر سه بعدی، مدل سازی اطلاعات ساختمانی، IFC، مدل داده سه بعدی -
صفحات 131-140
فقدان ابزارهای کارآمد برای اندازه گیری درختان، چالشی اساسی در آماربرداری جنگل است. فن آوری مدت زمان پرواز (TOF) با استفاده از تکنیک های فتوگرامتری و توانایی سنجش عمق، امکان سه بعدی سازی و اندازه گیری اجسام مختلف از جمله تنه درختان را فراهم می کند. در تحقیق حاضر به بررسی دقت اندازه گیری قطر با استفاده از فن آوری TOF نسبت به کالیپر، برای شش گونه راش، بلندمازو، افرا پلت، ممرز، توسکا و انجیلی (که به لحاظ فرم تنه و ضخامت پوست متفاوت هستند) پرداخته شد. بدین منظور ابتدا 20 اصله از هر گونه، در جنگل آموزشی-پژوهشی دارابکلا مازندران به صورت تصادفی انتخاب گردید. سپس قطر در ارتفاع 1 و 30/1 متری تنه درختان با استفاده از کالیپر اندازه گیری شد. همچنین ابرنقاط تنه درختان توسط گوشی هوشمند Phab2Pro تولید و قطر های هدف با استفاده از نرم افزارCloudCompare اندازه گیری شدند. نتایج RMSE حاکی از عدم وجود اختلاف معنی دار بین آماربرداری زمینی و اندازه گیری با ابزار TOF در گونه های مختلف است. با این وجود گونه های افرا، راش و بلندمازو خطای اندازه گیری کمتری نسبت به ممرز، توسکا و انجیلی داشته اند. همچنین مقدار RMSE کل برای قطر در ارتفاع 1 و 30/1 متر به ترتیب 01/1 و 87/0 سانتی متر به دست آمد. شاخص Bias نشان داد، مقادیر اندازه گیری شده با فن آوری TOF برای اغلب گونه ها بیش از مقادیر کالیپر بوده است. با توجه به نتایج به دست آمده و نظر به قابلیت های فراوان فن آوری TOF از جمله توان اندازه گیری قطر در ارتفاعات مختلف، تولید تصاویر سه بعدی رنگی، سرعت و دقت اسکن بالا، پیچیدگی کم، سبکی ابزار و هزینه کمتر نسبت به سایر فن آوری های سه بعدی سازی، گزینه مناسبی جهت جایگزینی با ابزارهای رایج در اندازه گیری جنگل می باشد.
کلیدواژگان: آماربرداری جنگل، مدت زمان پرواز، RGB-D SLAM، ابر نقاط، کالیپر
-
Pages 1-10
Panicle counts (PC) provide valuable information about yield prediction in sorghum but are expensive and time-consuming to acquire via traditional manual approaches. In this thesis, high-resolution RGB imagery acquired by UAVs has been used. The proposed method based on task-aware spatial disentanglement (TSD) has been modified to improve the performance of panicle detection. TSDPC has high accuracy in comparison to state-of-the-art techniques such as CenterNet and RepPoints.
Keywords: Deep Learning, UAV Images, Panicle Counting, Small Objects -
Pages 11-23
Rapid urban growth, especially in developing countries, is causing a large number of urban planning problems. Although only three percent of the global land surface is covered by urban areas, approximately 54% of the world’s population lives in urban centers; according the latest estimates, by 2050 it will increase to nearly 65%. Accurate information on Urban Land Cover (ULC) types and their spatial distribution are of paramount importance for urban planning and management. To date, many studies have been conducted in the context of ULC mapping, and several methodologies and datasets have been used (e.g. land surveying and satellite data) in this regard. Under this background, generating ULC maps using land surveying method is considered as the most accurate technique, however, it is a costly and time-consuming task. Spending the least time and cost to produce these maps is one of the main challenges for city managers. To address this issue, the integration of satellite images and state-of-art classification methods has been received considerable attention in recent years. This study seeks to produce a 10 m resolution ULC map for Tabriz city, locating North East of Iran, using Sentinel-2 satellite data. The present study also aims to compare the potential of two advanced classifiers including Random Forest (RF) and Deep Neural Network (DNN) in ULC mapping. Five ULC classes including bare land, built-up areas, road, vegetation, and water were considered in this regard. As the number of trees (ntree) and the number of variables (mtry) are two main criteria applying the RF algorithm. In this study, ntree was set to 100 and the mtry was set to the square root of the total number of input features. In the case of DNN, a DNN model with six layers, including one input layer with 10 neurons (bands 2-8A and 11-13 of sentinel-2), four hidden layers with 200 neurons per layer, and one output layer (five ULC classes). In this study, the ReLU activation function was used for the hidden layers, softmax activation function was used for classifying information in the output layer. Our findings illustrated that the DNN algorithm by providing 95.2% overall accuracy outperformed RF (overall accuracy = 93.1%). Analyzing the performance of two algorithms regarding ULC classes showed that the DNN algorithm provided better results in bare land and built-up classes; the user’s accuracy and producer’s accuracy of bare land class were respectively 9.6% and 1% higher than those of RF. Regarding the built-up class, these metrics were also higher than RF (user’s accuracy = + 0.3% and producer’s accuracy = + 4.3%). In contrast, the RF algorithm performed better in extracting the road class; the user’s accuracy and producer’s accuracy of road class were 3.65% and 4.1% more than those of DNN, respectively. RF and DNN showed the same performances in classifying vegetation and water classes. In general, both algorithms provided good performances in ULC classification, however, the overall performance obtained by the DNN algorithm was substantially higher than RF. Because the performance of the DNN algorithm is better than the RF algorithm, we concluded that DNN is a valid alternative tool that should be considered for ULC mapping.
Keywords: Urban Land Cover, DNN, Random Forest, Sentinel-2 -
Pages 25-37
The selection of an optimal and appropriate place for temporary accommodation of people affected by natural disasters, such as flood, has long been of concern to Crisis Management Planners. Failure to properly locate these centers may result in heavy damage. The objective of the present study is to determine appropriate places for establishing temporary post-flood settlement centers in Mazandaran province. In order to achieve this objective, firstly, effective criteria for locating temporary accommodation were determined and the standard maps were prepared and normalized. In the present study, nine criteria for locating temporary accommodation centers have been applied. The Analytic Hierarchy Process (AHP) method has been applied to weigh the criteria by availing experts’ opinions and studying related articles. In the next step, in order to incorporate the criteria according to the weights calculated by the method of AHP, the method Weighted Linear Combination (WLC) was applied and the final map was categorized into four classes of “very appropriate”, “appropriate”, “not appropriate” and “very inappropriate”. In conclusion, the location of temporary post-flood settlement centers with respect to demographic points was considered in the two classes of “very appropriate” and “appropriate” from the previous stage using the P_Median function in the genetic algorithm. The algorithm searches for 15 locations among the candidate points for temporary accommodation. The centers are located in higher populated areas in two classes more desirable than other classes.
Keywords: Temporary Sheltering, Flood, GIS, AHP, GA, P-Median -
Pages 39-53
There are many instabilities in the earth's rotation due to celestial bodies, gravitational forces, and earth internal dynamics which make the calculation of Earth Orientation Parameters (EOP) and Polar Motion (PM) parameters a challenging task. In today's world due to the increasing requests for predicting EOP and PM parameters in a wide range of fields such as Astronomy, Geodesy, Oceanography, and Hydrography various methods are used. These days it is possible to calculate accurate values of EOP and PM parameters by means of global positioning system (GPS), very-long baseline interferometry (VLBI) and satellite laser ranging (SLR). The core reason for the short-term prediction of these parameters is the impossibility of calculating these parameters in real-time due to heavy preprocessing procedures. Hence, researchers are seeking to employ different methods for accurate short-term prediction of EOP and PM parameters. In recent years, non-parametric methods such as least square (LS) with autoregressive moving average (ARMA) and also singular spectrum analysis (SSA) have been used to estimate these parameters. Another method for the prediction of the aforementioned parameters was conventional artificial neural network (ANN). Currently, Deep Learning has become a popular field that attracts many researchers. Deep learning is a subset of artificial intelligence (AI) and machine learning that uses multiple layers and parameters in order to extract complex features from the inputs. It is widely used in computer vision and time series prediction applications. In this paper, we used three deep learning methods namely LSTM, CNN, and MLP in order to predict PM parameters (x and y parameters). Furthermore, we have used Least square harmonic estimation (LSHE) method in order to compare the final results with different networks. LSTM equipped with a short-term recursive memory. This recursive mechanism prepares LSTM for handling time series data. CNN extracts important features of input data by convolution multiplication and each convolution layer within CNN architecture produces feature maps as output. These Feature maps contain recognized patterns which will be used as input of next layer. CNN networks, which were primarily designed for the computer vision, are being used more and more in timeseries prediction applications. MLP networks are similar to conventional back propagation feedforward networks, however, new activation functions and optimizers could be used in MLP networks. For sufficient training of different architectures, we used 35 years of daily PM parameters from 1st January 1980 to 31 December 2015 and we predicted 40 days periods ahead for the future 5 years. For comparison of predicted values by different networks, we used mean absolute error (MAE) as a criterion and illustrate results in two tables. Also, we depicted different figures to show how networks are working. In addition, we used two LSTM, four CNN, and two MLP Networks with ADAM optimizer, ReLU activation function, and learning rate of 0.001- 0.0001 in order to select the best network with the lowest errors. Moreover, the figures of predicted values vs actual values and plots of MAE for 40 days are shown on four figures for better comprehension of ultimate results. In the end, it turned out that LSTM networks outperformed CNN and MLP networks and in this network the final results are better than the others in most days. For the x parameter in the first, twentieth and fortieth days, the best MAE values are 0.41 mas, 5.58 mas, and 12.45 mas and the best values for RMSE are 0.49 mas, 6.69 mas, 15.05 mas, respectively. For the y parameter in the first, twentieth and fortieth days, the best values of MAE are 0.54 mas, 3.24 mas and 7.56 mas and the best values for RMSE are 0.68 mas, 4.72 mas, 9.22 mas, respectively. The final results show that the neural networks outperformed LSHE method and the accuracies of the deep learning networks are satisfying and LSTM and CNN networks are capable to predict values accurately.
Keywords: Timeseries, Polar Motion, Deep Learning, LSTM, CNN, MLP -
Pages 55-65
Environmental pollution and disasters have gradually increased with population growth. The presence of oil resources in the seas and the incidents related to their discovery, extraction and transportation cause the formation of oil slicks on the sea surface, and the leakage of these petroleum products into the seas has irreparable environmental consequences. That is why monitoring the effects of these accidents is very important for public health. Satellite missions are a very effective tool for detecting pollutants such as oil spills. Artificial Aperture Radar Sensor (SAR) is an active microwave detection system that can be used to detect oil leaks with optical sensors installed on Landsat-8, Sentinel-2 and Ester satellite systems, taking into account cloud cover and satellite re-visit time at the same location. Be. In this study, the oil spill area due to pipe leakage in Khark Island was studied with Landsat-8 and Sentinel-1 satellite images. Various image processing techniques were applied to Landsat-8 bands to highlight oil spills in connection with the accident, such as morphology and convolution filters. We used Landsat-8 images to support the Sentinel-1 results. Oil spills were successfully detected by analyzing SAR data and Landsat-8 results, and by visually interpreting the results, the selected methods are consistent in terms of displaying oil spill areas.
Keywords: Oil spill, Sentinel-1, Landsat-8, image processing -
Pages 67-82
In recent years, Road detection and road extraction from satellite images with the advancement and development of deep learning algorithms in the field of semantic segmentation has received more and more attention of researchers. In this regard, most of the studies have been done in the field of Road detection and road extraction using optical images and in these studies, few studies have been performed using radar images worldwide. Therefore, the aim of this study was to use a deeply Refined Deep Residual Convolutional Neural Network (RDRCNN) to evaluate and compare the accuracy of road extraction from Sentinel 1 radar images in Tehran and Shiraz metropolitan areas in equal conditions in terms of number of educational samples, validation and architecture. It is the same. In this study, to extract the road using DNN, the VV-VH color combination of Sentinel 1 radar images from 8 different cities (Tehran, Mashhad, Isfahan, Shiraz, Tabriz, Urmia, Baghdad and Beijing) was used. Finally, the RDRCNN model with a residual connected unit (RCU) and a dilated perception unit (DPU) was used for road training and extraction. The research findings indicate that the RDRCNN model has performed almost the same in the process of identifying and extracting roads in the two cities of Tehran and Shiraz, and in general, the above model has performed slightly better in the city of Shiraz. In terms of accuracy evaluation metrics, for Tehran images, the criteria were Recall 57.66%, accuracy 51.29%, F1 score 54.43% and overall accuracy 92.78%, and for Shiraz images Recall criteria 60.77%, accuracy 54.71%, F1 score 57.40% and overall accuracy of 95.63% were obtained. The findings of this study show the low accuracy of road training and extraction from Sentinel 1 radar images for two metropolitan areas of Iran. In general, by comparing the results of this study with previous studies, it can be seen that one of the most important reasons for the low accuracy of the results is the low width of roads in Iranian cities; However, due to the lack of necessary studies in the field of road extraction with Sentinel 1 radar images, it is not possible to comment definitively on the results and it is suggested that more studies be done in this field.
Keywords: Deep Learning, RDRCNN, Sentinel 1, Road Extraction, Tehran, Shiraz -
Pages 83-96
With increasing the number of large engineering structures in cities, experts are looking for a good solution for monitoring these structures to avoid great financial and human damages. From the past, leveling and ground surveying were carried out to measure the deformation of structures and ground displacements along the vertical direction; but these measurements are time-consuming and costly. Also, the using of precision instruments and deformation sensors are not suitable because of their high cost, time-consuming and complexity. Due to the ability of Radar images and Radar interferometry techniques in the field of monitoring the ground displacement, in this research, we are looking for evaluating the potential of this method for monitoring the dam deformation and displacements. To achieve this goal, we used two sets of radar data which are CosmoSkyMed-X and Sentinel-1A. In the time series processing of these images, the PSI method was selected then the star graph and Deloney triangulation were used. In the next step, we used both linear and nonparametric models for displacement estimation. The results were evaluated by applying two different displacement models and finally, the model with higher temporal coherence was selected as the appropriate model and the other model was discarded. In processing the Mamlu dam images, the appropriate model for monitoring the displacements with S-1A Radar data was the nonparametric model and for CSK data was linear model. Due to the lack of ground data collection from Mamlu dam in the same period of time with radar data, to evaluate the accuracy and proof the obtained results, the results of two radar data sets (CSK and S-1A) were compared with each other and a very high agreement was observed between the results of these data sets that the amount of RMSE calculated for ASC data of these two sensors is equal to 0.7703 mm and for DSC data was calculated 0.9551 mm, which is a very high consistency of the results, which can be a reason for the accuracy of the extracted results.
Keywords: Radar interferometry, Persistent scatterer technique, Earth-fill dam, CosmoSkyMed-X images, Sentinel-1A images -
Pages 97-105
The Differential Interferometric Synthetic Aperture Radar (DInSAR) can be considered an efficient and cost-effective method for monitoring ground subsidence because of its extensive spatial coverage and high precision. Because of orderly observations from a broad-range product, the new commissioning of the first Sentinel-1 satellite offers better support to operational scrutinies via DInSAR. In the present paper, the results of a Small Baseline Subset Interferometric Synthetic Aperture Radar (SBAS-InSAR) time-series analysis of 42 Interferometric Wide swath (IW) products of Urmia Lake Bridge in northwestern Iran acquired between November 2014 to April 2021 for both descending and ascending pass using the Sentinel-1A observation with Progressive Scans in azimuth (TOPS) imaging mode is studied. The SBAS processing was based upon the analysis of 111 small-baseline differential interferograms. The results demonstrate that the majority of regional ground subsidence rates in the research area ranged from 10 to 210 mm during the study period. Also, the maximum subsidence rate exceeded 210 mm/year. The Line of Sight (LOS) direction for descending pass is 91 mm/year for ascending the pass. The board view displays that ground subsidence is intense on the bridge. The largest subsidence center is located at the central points of the bridge. GPS data verified the SBAS-InSAR-derived result. The results include displacement in the horizontal and vertical directions for ascending and descending passes.
Keywords: InSAR, Sentinel 1, Subsidence, SBAS, SARscape, Interferogram -
Pages 107-117
Spatial data infrastructure is a set of policies, standards, access networks, spatial data, organizations and people that facilitate and coordinate the various tasks of production, collection, storage, access and optimal use of spatial data in a specific area. In order to implement the provincial spatial data infrastructure, the provincial management and planning organization will be responsible for leading and coordinating between the executive organs of the province and the establishment of the provincial geoportal. In order to measure the success of spatial data infrastructure implementation in Ilam province, first the effective factors and indicators in the successful implementation of provincial SDI were identified and then weighed using the combined method of Dematel technique and network analysis process (DANP), which the indicators of structure, financial resources, specialization, education and culture, respectively were the most important. Then the implementation of each of the criteria in the province was examined. Finally, the success rate of spatial data infrastructure implementation in Ilam province was calculated. The results showed that the realization rate of SDI in Ilam province is 63%, which according to the existing conditions, the performance of this province can be at a good level. Finally, the weaknesses in the implementation of SDI in Ilam province identification and solutions to eliminate them and increase the success rate of SDI in this province were expressed.
Keywords: Spatial Data Infrastructure (SDI), Demetel, Network Analysis Process (DANP), Ilam Province -
Pages 119-130
In recent years, the population in Iran has been increasing. This growth in the population of large cities multiplies the demand for dwellings. The increase in demand, in turn, has led to the creation of high-rise buildings and numerous complex registration maps in these buildings. The current method for registering and storing various legal boundaries in Iran is based on two-dimensional maps. This method has its limitations for visualizing different registration boundaries that define legal spaces. For this reason, a new method to register the ownership of buildings accurately and efficiently is more than ever needed. With the advancement in the field of 3D modeling, especially Building Information Modeling, a lot of research is being done to record the three-dimensional legal boundaries using these models in different countries. In this article, for the first time, Building Information Modeling was used to record three-dimensional legal boundaries in the Iranian jurisdiction. To do this, first different types of registration boundaries in Iran were identified according to the regulations of the Real Estate Registration Organization of Iran and the building subdivision instructions in Iran. Then, to record these boundaries, Industry Foundation Classes (IFC) was developed and implemented on a prototype, which is a building in Tehran. In the evaluation stage, the registration boundaries in the two proposed methods and the current method were compared using a questionnaire. The results of this evaluation in different criteria showed the high capability of the proposed method in registering and visualization legal boundaries approved by the Real Estate Registration Organization so that more than 80% of the elite community participating in the questionnaire considered this method more appropriate than the current method.
Keywords: property registration, 3D cadastre, Building Information Modeling, IFC, 3D data model -
Pages 131-140
The lack of efficient inventory tools is an old and well-known problem related to in situ forest measurements. Time of flight technology (TOF), using photogrammetric techniques and the ability to detect depth, allows the creation of 3D point clouds and the measurement of various objects, including tree stems. Therefore, this paper presents the accuracy of diameter measurement with TOF technology compared to calipers for six tree species: (Fagus orientalis Lipsky), (Quercus castanaefulia C.A.M. subsp), (Acer velutinum Boiss), (Carpinus betulus L.), (Alnus subcordata C.A.M.), (Parrotia persica C.A.Mey.), differing in stem shape and bark (20 of each species) were studied in the Darabkola Research Forest. Then, the diameters at a height of 1 and 1.30 metres of the tree trunks were measured with a vernier calliper and a point cloud was created using a Phab 2 Pro smartphone. The target diameters were measured using the CloudCompare software. The results showed that there was not much difference between the field recordings and the TOF measurement. However, Acer velutinum, Fagus orientalis and Quercus castanaefulia had fewer measurement errors than Parrotia persica, Alnus glutinosa and Carpinus betulus. Overall, the diameter RMSE at a height of 1 m and 1.30 m was 1.01 cm and 0.87 cm, respectively. According to the Bias index, the values measured with the TOF technology were higher than those measured with the caliper for most species were. Due to the results obtained and the many possibilities of TOF technology, such as the possibility to measure the diameter at different heights, the creation of RGB-3D, the high scanning speed and accuracy, low complexity, the brightness and the lower cost than other technologies, three-dimensional surveying is a good option for forest inventory verification.
Keywords: Forest inventory, RGB-D SLAM, Time of Flight, Point cloud, Caliper