فهرست مطالب
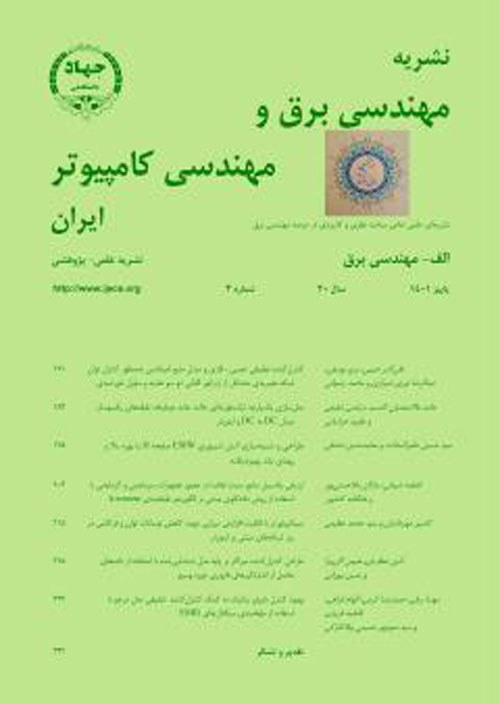
نشریه مهندسی برق و مهندسی کامپیوتر ایران
سال بیستم شماره 3 (پیاپی 73، پاییز 1401)
- تاریخ انتشار: 1401/09/13
- تعداد عناوین: 7
-
-
صفحات 185-195
امروزه با افزایش حجم تولید داده، توجه به الگوریتمهای یادگیری ماشین جهت استخراج دانش از دادههای خام افزایش یافته است. داده خام معمولا دارای ویژگیهای اضافی یا تکراری است که بر روی عملکرد الگوریتمهای یادگیری تاثیر میگذارد. جهت افزایش کارایی و کاهش هزینه محاسباتی الگوریتمهای یادگیری ماشین، از الگوریتمهای انتخاب ویژگی استفاده میشود که روشهای متنوعی برای انتخاب ویژگی ارایه شده است. از جمله روشهای انتخاب ویژگی، الگوریتمهای تکاملی هستند که به دلیل قدرت بهینهسازی سراسری خود مورد توجه قرار گرفتهاند. الگوریتمهای تکاملی بسیاری برای حل مسیله انتخاب ویژگی ارایه شده که بیشتر آنها روی فضای هدف تمرکز داشتهاند. فضای مسیله نیز میتواند اطلاعات مهمی برای حل مسیله انتخاب ویژگی ارایه دهد. از آنجایی که الگوریتمهای تکاملی از مشکل عدم خروج از نقطه بهینه محلی رنج میبرند، ارایه یک مکانیزم موثر برای خروج از نقطه بهینه محلی ضروری است. در این مقاله از الگوریتم تکاملی PSO با تابع چندهدفه برای انتخاب ویژگی استفاده شده که در آن یک روش جدید جهش که از امتیاز ویژگیهای ذرات استفاده میکند، به همراه نخبهگرایی برای خروج از نقاط بهینه محلی ارایه گردیده است. الگوریتم ارایهشده بر روی مجموعه دادههای مختلف تست و با الگوریتمهای موجود بررسی شده است. نتایج شبیهسازیها نشان میدهند که روش پیشنهادی در مقایسه با روش جدید RFPSOFS بهبود خطای 20%، 11%، 85% و 7% به ترتیب در دیتاستهای Isolet، Musk، Madelon و Arrhythmia دارد.
کلیدواژگان: انتخاب ویژگی، بهینه سازی چندهدفه، الگوریتم PSO، مجموع وزن دار تطبیق پذیر، جهش هوشمند، نخبه گرایی -
صفحات 196-206
با توجه به سرعت روزافزون تولید اطلاعات و نیاز تبدیل اطلاعات به دانش، روشهای یادگیری ماشین قدیمی دیگر پاسخگو نیستند. هنگام استفاده از طبقهبندیها با روشهای یادگیری ماشین قدیمی، به ویژه استفاده از طبقهبندیهای ذاتا تنبل مانند روش k- نزدیکترین همسایگی (KNN)، عملیات طبقهبندی دادههای حجیم بسیار کند است. نزدیکترین همسایگی به دلیل سادگی و دقت عملی که ارایه میدهد یک روش محبوب در زمینه طبقهبندی دادهها میباشد. روش پیشنهادی مبتنی بر مرتبسازی بردارهای ویژگی دادههای آموزشی در یک درخت جستجوی دودویی است تا طبقهبندی دادههای بزرگ را با استفاده از روش نزدیکترین همسایگی تسریع بخشد. این کار با استفاده از یافتن تقریبی دو دورترین داده محلی در هر گره درخت انجام میشود. این دو داده به عنوان معیار برای تقسیم دادههای موجود در گره فعلی بین دو گروه، مورد استفاده قرار میگیرند. مجموعه دادههای موجود در هر گره بر اساس شباهت آنها به این دو داده، به فرزند چپ یا راست گره فعلی تخصیص داده میشوند. نتایج آزمایشهای متعدد انجامشده بر روی مجموعه دادههای مختلف از مخزن UCI، میزان دقت خوب با توجه به زمان اجرای کم روش پیشنهادی را نشان میدهد.
کلیدواژگان: بافر همپوشانی، داده های حجیم، درخت تصمیم دودویی، طبقه بندی نزدیک ترین همسایگی -
صفحات 207-216
محاسبات کوانتومی، روش جدیدی از پردازش اطلاعات است که بر مبنای مفاهیم مکانیک کوانتومی بنا شده و منجر به رخدادهای عجیب و قدرتمندی در حوزه کوانتوم میشود. سنتز منطقی مدارهای کوانتومی به فرایند تبدیل یک گیت دادهشده کوانتومی به مجموعهای از گیتها با قابلیت پیادهسازی در تکنولوژیهای کوانتومی اطلاق میشود. از معروفترین روشهای سنتز منطقی CSD و QSD هستند. هدف اصلی این مقاله، ارایه یک روش سنتز منطقی چندهدفه ترکیبی از دو روش فوق در مدل مداری محاسباتی با هدف بهینهسازی معیارهای ارزیابی است. در این روش پیشنهادی، فضای جوابی از ترکیبهای مختلف روشهای تجزیه CSD و QSD ایجاد میشود. فضای جواب ایجادشده، یک فضا با اندازه نمایی بسیار بزرگ است. سپس با استفاده از یک رهیافت پایین به بالا از روش حل برنامهریزی پویای چندهدفه، روشی ارایه میشود تا تنها بخشی از کل فضای جواب، برای یافتن مدارهایی با هزینههای بهینه پرتو جستجو شوند. نتایج به دست آمده نشان میدهند که این روش، موازنهای بین معیارهای ارزیابی ایجاد میکند و پاسخهای بهینه پرتو متعددی تولید کرده که با توجه به تکنولوژیهای مختلف کوانتومی میتوانند انتخاب شوند.
کلیدواژگان: محاسبات کوانتومی، مدل مداری کوانتومی، سنتز منطقی، بهینه سازی چندهدفه، برنامه ریزی پویا -
صفحات 217-226
بهکارگیری دادههای بدون برچسب در خودآموزی نیمهنظارتی میتواند به طور قابل توجهی دقت طبقهبند نظارتشده را بهبود بخشد، اما در برخی موارد ممکن است دقت طبقهبندی را به مقدار چشمگیری کاهش دهد. یکی از دلایل چنین تنزلی، برچسبگذاری اشتباه به دادههای بدون برچسب میباشد. در این مقاله، روشی را برای برچسبگذاری با قابلیت اطمینان بالا به دادههای بدون برچسب پیشنهاد میکنیم. طبقهبند پایه در الگوریتم پیشنهادی، ماشین بردار پشتیبان است. در این روش، برچسبگذاری فقط به مجموعهای از دادههای بدون برچسب که از مقدار مشخصی به مرز تصمیم نزدیکتر هستند انجام میشود. به این دادهها، دادههای دارای اطلاعات میگویند. اضافهشدن دادههای دارای اطلاعات به مجموعه آموزشی در صورتی که برچسب آنها به درستی پیشبینی شود در دستیابی به مرز تصمیم بهینه تاثیر بهسزایی دارد. برای کشف ساختار برچسبزنی در فضای داده از الگوریتم اپسیلون- همسایگی (DBSCAN) استفاده شده است. آزمایشهای مقایسهای روی مجموعه دادههای UCI نشان میدهند که روش پیشنهادی برای دستیابی به دقت بیشتر طبقهبند نیمهنظارتی خودآموز به نسبت برخی از کارهای قبلی عملکرد بهتری دارد.
کلیدواژگان: الگوریتم اپسیلون- همسایگی (DBSCAN)، الگوریتم خودآموزی، طبقه بندی نیمه نظارتی، ماشین بردار پشتیبان -
صفحات 227-235
در سالهای اخیر، شبکههای عصبی نوری به علت سرعت بالا و توان مصرفی پایینی که دارند، بسیار مورد توجه قرار گرفتهاند. با این وجود، این شبکهها هنوز محدودیتهای زیادی دارند که یکی از این محدودیتها پیادهسازی لایه غیر خطی در آنهاست. در این نوشتار، پیادهسازی واحد غیر خطی برای شبکههای عصبی پیچشی نوری مورد بررسی قرار گرفته تا در نهایت با استفاده از این واحد غیر خطی بتوان به یک شبکه عصبی پیچشی تمامنوری عمیق با دقتی مشابه شبکههای الکتریکی، سرعت بالاتر و توان مصرفی کمتر رسید و بتوان قدمی در راستای کاهش محدودیتهای این شبکهها برداشت. در این راستا ابتدا روشهای مختلف پیادهسازی واحد غیر خطی مرور شدهاند. سپس به بررسی تاثیر استفاده از جاذب اشباعشونده به عنوان واحد غیر خطی در لایههای مختلف بر دقت شبکه پرداخته شده و نهایتا روشی نوین و ساده برای جلوگیری از کاهش دقت شبکههای عصبی در صورت استفاده از این تابع فعالساز ارایه گردیده است.
کلیدواژگان: پردازش نوری، تابع فعال ساز نوری، سرعت بالا، شبکه عصبی پیچشی، شبکه عصبی پیچشی نوری -
صفحات 236-244
سیستمهای توصیهگر مبتنی بر جلسه بر اساس رفتار و تعاملات کاربر در یک جلسه، رفتار بعدی یا علاقه کاربر را پیشبینی کرده و بر این اساس، آیتمهای مناسب را به کاربر پیشنهاد میدهند. مطالعات اخیر برای ایجاد توصیهها عمدتا روی اطلاعات جلسه فعلی متمرکز شدهاند و اطلاعات جلسات قبلی کاربر را نادیده میگیرند. در این مقاله، یک مدل توصیهگر مبتنی بر جلسه شخصیسازی شده با شبکههای خودتوجه پیشنهاد میشود که علاوه بر جلسه فعلی از جلسات قبلی اخیر کاربر هم استفاده میکند. مدل پیشنهادی به منظور یادگیری وابستگی کلی بین همه آیتمهای جلسه، از شبکههای خودتوجه (SAN) استفاده میکند. ابتدا SAN مبتنی بر جلسات ناشناس آموزش داده میشود و سپس برای هر کاربر، توالیهای جلسه فعلی و جلسات قبلی به صورت جداگانه به شبکه داده میشود و با ترکیب وزنی نتایج رتبهبندی حاصل از هر جلسه، آیتمهای توصیهشده نهایی به دست میآید. مدل پیشنهادی بر روی مجموعه داده واقعی Reddit در دو معیار دقت و میانگین رتبه متقابل، تست و ارزیابی شده است. مقایسه نتایج حاصل از مدل پیشنهادی با رویکردهای قبلی، توانایی و اثربخشی مدل پیشنهادی را در ارایه توصیههای دقیقتر نشان میدهد.
کلیدواژگان: توصیه گر شخصی سازی شده، توصیه گر مبتنی بر جلسه، شبکه های خودتوجه، یادگیری عمیق -
صفحات 245-252
بازیابی تصاویر مبتنی بر محتوا که با عنوان پرس وجو بر اساس محتوای تصویر نیز شناخته می شود، یکی از زیرشاخه های بینایی ماشین است که جهت سازماندهی و تشخیص محتوای تصاویر دیجیتال با بهره گیری از ویژگی های بصری به کار می رود. این تکنولوژی، تصاویر مشابه با تصویرپرس وجو را از پایگاه داده های عظیم تصویری بهصورت خودکار جستجو میکند و با استخراج مستقیمویژگی های بصری از داده های تصویری و نه کلمات کلیدی و حاشیه نویسی های متنی، مشابهترین تصاویر را برای کاربران فراهم میآورد؛ بنابراین در این مقاله روشی ارایهشده است که جهت کاهش شکاف معنایی میانویژگیهای بصری سطح پایین و معانی سطح بالای تصاویر از تبدیل موجک و ترکیب ویژگیها با هیستوگرام رنگ استفاده می گردد. در این راستا، خروجی نهایی، از پایگاه داده ی تصویر با بهره گیری از روش استخراج ویژگی از تصاویر ورودی، خواهد بود.در گام بعد، هنگامیکه تصاویر پرس وجو توسط کاربر هدف به سیستم داده می شود، جهت بازیابی تصاویر مرتبط، از یادگیری نیمه نظارتشده که از ترکیب روشهای خوشهبندی و طبقهبندی بر اساس کاوش الگوهای مکرر حاصل می شود، مشابهترین تصاویربرای کاربران بازیابی میگردد. نتایج آزمایش نشان میدهد که سیستم پیشنهادی، بالاترین میزاناثربخشی را در مقایسه با سایر روشهای مورد مقایسه ارایه نموده است.
کلیدواژگان: تبدیل موجک، توصیه گر تصویر، کاوش الگوهای مکرر، یادگیری ماشین
-
Pages 185-195
Today, with the increase in data production volume, attention to machine learning algorithms to extract knowledge from raw data has increased. Raw data usually has redundant or irrelevant features that affect the performance of learning algorithms. Feature selection algorithms are used to improve efficiency and reduce the computational cost of machine learning algorithms. A variety of methods for selecting features are provided. Among the feature selection methods are evolutionary algorithms that have been considered because of their global optimization power. Many evolutionary algorithms have been proposed to solve the feature selection problem, most of which have focused on the target space. The problem space can also provide vital information for solving the feature selection problem. Since evolutionary algorithms suffer from the pain of not leaving the local optimal point, it is necessary to provide an effective mechanism for leaving the local optimal point. This paper uses the PSO evolutionary algorithm with a multi-objective function. In the proposed algorithm, a new mutation method that uses the particle feature score is proposed along with elitism to exit the local optimal points. The proposed algorithm is tested on different datasets and examined with existing algorithms. The simulation results show that the proposed method has an error reduction of 20%, 11%, 85%, and 7% in the Isolet, Musk, Madelon, and Arrhythmia datasets, respectively, compared to the new RFPSOFS method.
Keywords: Feature selection, multi-objective optimization, PSO algorithm, adaptive weight sum method, intelligent mutation, elitism -
Pages 196-206
Due to the increasing speed of information production and the need to convert information into knowledge, old machine learning methods are no longer responsive. When using classifications with the old machine learning methods, especially the use of inherently lazy classifications such as the k-nearest neighbor (KNN) method, the operation of classifying large data sets is very slow. Nearest Neighborhood is a popular method of data classification due to its simplicity and practical accuracy. The proposed method is based on sorting the training data feature vectors in a binary search tree to expedite the classification of big data using the nearest neighbor method. This is done by finding the approximate two farthest local data in each tree node. These two data are used as a criterion for dividing the data in the current node into two groups. The data set in each node is assigned to the left and right child of the current node based on their similarity to the two data. The results of several experiments performed on different data sets from the UCI repository show a good degree of accuracy due to the low execution time of the proposed method.
Keywords: Overlap buffer, big data, binary decision tree, nearest neighbor classification -
Pages 207-216
Quantum computing is a new method of information processing that is based on the concepts of quantum mechanics and leads to strange and powerful events in the quantum field. The logic synthesis of quantum circuits refers to the process of converting a given quantum gate into a set of gates that can be implemented in quantum technologies. The most famous logic synthesis methods are CSD and QSD. The main goal of this study is to present a multi-objective logical synthesis method combining the above two methods in the quantum circuit model with the aim of optimizing the evaluation criteria. In this proposed method, the solution space is created from different combinations of CSD and QSD decomposition methods. The created solution space is a space with a very large exponential size. Then, using a bottom-up approach of multi-objective dynamic programming, a method is presented to search only a part of the entire solution space to find circuits with the optimal Pareto costs. The obtained results show that this method creates a balance between the evaluation criteria and produces many optimal Pareto solutions that can be selected according to different quantum technologies.
Keywords: Quantum computing, quantum circuit model, logic synthesis, multi-objective optimization, dynamic programming -
Pages 217-226
Using the unlabeled data in the semi-supervised learning can significantly improve the accuracy of supervised classification. But in some cases, it may dramatically reduce the accuracy of the classification. The reason of such degradation is incorrect labeling of unlabeled data. In this article, we propose the method for high confidence labeling of unlabeled data. The base classifier in the proposed algorithm is the support vector machine. In this method, the labeling is performed only on the set of the unlabeled data that is closer to the decision boundary from the threshold. This data is called informative data. the adding informative data to the training set has a great effect to achieve the optimal decision boundary if the predicted label is correctly. The Epsilon- neighborhood Algorithm (DBSCAN) is used to discover the labeling structure in the data space. The comparative experiments on the UCI dataset show that the proposed method outperforms than some of the previous work to achieve greater accuracy of the self-training semi-supervised classification.
Keywords: Epsilon- neighborhood Algorithm (DBSCAN), Self-training Algorithm, Semi-supervised classification, Support vector machine -
Pages 227-235
In recent years, optical neural networks have received a lot of attention due to their high speed and low power consumption. However, these networks still have many limitations. One of these limitations is implementing their nonlinear layer. In this paper, the implementation of nonlinear unit for an optical convolutional neural network is investigated, so that using this nonlinear unit, we can realize an all-optical convolutional neural network with the same accuracy as the electrical networks, while providing higher speed and lower power consumption. In this regard, first of all, different methods of implementing optical nonlinear unit are reviewed. Then, the impact of utilizing saturable absorber, as the nonlinear unit in different layers of CNN, on the network’s accuracy is investigated, and finally, a new and simple method is proposed to preserve the accuracy of the optical neural networks utilizing saturable absorber as the nonlinear activating function.
Keywords: Optical Processing, optical activation function, high-speed, convolutional neural networks, optical convolutional neural networks -
Pages 236-244
Session-based recommender systems predict the next behavior or interest of the user based on user behavior and interactions in a session, and suggest appropriate items to the user accordingly. Recent studies to make recommendations have focused mainly on the information of the current session and ignore the information of the user's previous sessions. In this paper, a personalized session-based recommender model with self-attention networks is proposed, which uses the user's previous recent sessions in addition to the current session. The proposed model uses self-attention networks (SANs) to learn the global dependencies among all session items. First, SAN is trained based on anonymous sessions. Then for each user, the sequences of the current session and previous sessions are given to the network separately, and by weighted combining the ranking results from each session, the final recommended items are obtained. The proposed model is tested and evaluated on real-world Reddit dataset in two criteria of accuracy and mean reciprocal rank. Comparing the results of the proposed model with previous approaches indicates the ability and effectiveness of the proposed model in providing more accurate recommendations.
Keywords: Personalized recommendation, session-based recommendation, self-attention network, deep learning -
Pages 245-252
Content-based image retrieval, which is also known as query based on image content, is one of the sub-branches of machine vision, which is used to organize and recognize the content of digital images using visual features. This technology automatically searches the images similar to the query image from huge image database and it provides the most similar images to the users by directly extracting visual features from image data; not keywords and textual annotations. Therefore, in this paper, a method is proposed that utilizes wavelet transformation and combining features with color histogram to reduce the semantic gap between low-level visual features and high-level meanings of images. In this regard, the final output will be presented using the feature extraction method from the input images. In the next step, when the query images are given to the system by the target user, the most similar images are retrieved by using semi-supervised learning that results from the combination of clustering and classification based on frequent patterns mining. The experimental results show that the proposed system has provided the highest level of effectiveness compared to other methods.
Keywords: Wavelet transform, image recommender, frequent patterns mining, machine learning