فهرست مطالب
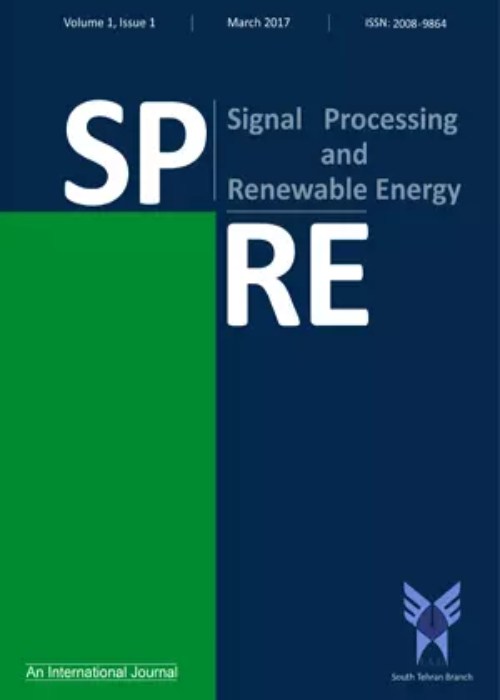
Signal Processing and Renewable Energy
Volume:7 Issue: 1, Winter 2023
- تاریخ انتشار: 1402/03/28
- تعداد عناوین: 6
-
Pages 1-12The length between the current point in the degradation process and the time of reaching the failure threshold, or the remaining usable life (RUL) prediction of systems, is of the greatest priority in the industry. More accurate estimation is useful for maintenance decisions as it helps to avoid catastrophic breakdowns and may also assist in reducing additional costs. Deep learning approaches have made impressive advancements in this field in recent years by becoming widely attractive and employed. However, most deep learning approaches don’t fully consider the information implications of sensors adaptively. To overcome this problem, a novel adaptive hybrid model that combines a convolutional neural network (CNN) and gated recurrent unit (GRU) is introduced in this work. The RUL estimation is based on the best practical option of sequence data through CNN-GRU. In the first step, optimal sensor selection is applied to the dataset to collect the most useful sensors. Then, the input data is transformed into a predefined range of values using standard and min-max scalars; in the next step, the normalized data is fed into the CNN-GRU model with an adaptive activation function for deep feature extraction, training, and RUL prediction. Utilizing CNN to extract features from the multivariate input data automatically, the features are then fed into the GRU layer to train the model for RUL prediction. To test the effectiveness of this framework, the suggested methodology is applied to the NASA Commercial Modular Aero-Propulsion System Simulation (C-MAPSS) dataset. The findings demonstrate that CNN-GRU is capable of accurate RUL prediction. In addition, CNN-GRU outperforms CNN-LSTM and CNN-RNN in terms of computation efficiency and accuracy.Keywords: Optimal sensor selection, Adaptive learning, CNN-GRU, Remaining Useful Life, Turbofan Engine
-
Pages 13-25
Fluorescent lamps are used as one of the most important sources of light in residential and commercial applications. A method to increase the power factor in electronic ballasts is proposed in this paper. For the power factor correction circuit, the amplification-based switching method is used, which increases the power factor and provides the DC voltage for starting the half-bridge inverter circuit. Finally, the laboratory sample of the circuit is made at a voltage of 110 V with a power factor of 0.996. This circuit has an efficiency of 94.7% and a total harmonic distortion less than 5%. In addition, the pressure on the lamp at the moment of commissioning is reduced, which increases the life of the lamp.
Keywords: power factor, Total harmonic distortion, fluorescent lamp -
Pages 27-39This study presents Interval Type-2 Fuzzy Evolutionary models to manage uncertainty in the process of uncertain time-series prediction. This study presents two type-2 fuzzy evolutionary models for rule extraction that were proposed: 1) Evolutionary Interval Type-2 Fuzzy Rule Learning (EIT2FRL), and 1) Evolutionary Interval Type-2 Fuzzy Rule-Set Learning (EIT2FRLS). A ROC curve analysis was applied for performance evaluation, and the results were validated using a 10-fold cross-validation technique. The results reveal that the proposed methods have an AUC of 0.96 for EIT2FRLS and 0.93 for EIT2FRL proposed methods. The results are promising for knowledge extraction in uncertain circumstances, predicting uncertain patterns prediction, and making suitable strategies and optimal decisions.Keywords: Evolutionary Algorithm, Type-2 Fuzzy Logic, Time-Series Prediction
-
Pages 41-55Nowadays, vehicles must be able to localize themselves in all environments, including urban areas and indoor environments where the performance of Global Navigation Satellite Systems (GNSS) may be reduced. In the studies conducted so far in urban environments, an inertial measurement unit (IMU) and an extended Kalman filter (EKF) have been presented. For indoor environments, a light and range detection system (LiDAR) has been developed, which was more accurate than previous methods. However, the accuracy of diagnosis should be improved by providing newer methods. Therefore, in this article, in order to increase the accuracy of the position error of the internal navigation system, an integrated developed IMU/LiDAR Kalman filter was used, and then by optimizing the parameters of the Kalman filter and the gray wolf meta-heuristic algorithm, an attempt was made to reduce the position error. In order to check the performance of the presented method, the results before using the optimization algorithm and after the optimization were evaluated using three different paths. The obtained results, including position, speed, and direction show that the accuracy of the results increases by using the gray wolf algorithm compared to the conventional model.Keywords: Indoor Vehicular Navigation, IMU, LiDAR, Extended kalman filter (EKF), Grey Wolf optimization
-
Pages 57-67In this research, we first present some background on the sample size estimation for conducting clinical trials, discussing the necessity of a computational enrichment criterion. The Denoising Autoencoder Stacked Deep Learning (DASDL) design and development are directly motivated by the optimal enrichment design. Although there are many types of deep architectures in the literature, we focus our presentation using two of the most widely used models stacked denoising autoencoders and fully-supervised dropout. The ideas presented here are applied to any such architectures used for learning problems in the small-sample regime. In this work, we propose a novel, scalable, deep learning method that is applicable for learning problems in the small sample regime and obtains reliable performance. The results show via extensive analyses using imaging, cognitive, and other clinical data alongside a ROC curve analysis. When used as trial inclusion criteria, the new computational markers result in cost-efficient clinical Alzheimer’s disease trials with moderate sample sizes.Keywords: Pattern Analysis, Medical imaging, deep learning, Alzheimer’s disease, Signal processing
-
Pages 69-78
Among the common structures of filters, electrostatic filters have been very important in the indu stry due to their various advantages. Pollutant separation in these filters happens by charging them and absorbing them in the electric field. Using a high-amplitude dc voltage to create a strong field and as a result discharging the corona and charging the pollutant particles are the basis of this sys tem. However, the use of pulsed voltages with high amplitude can bring better efficiency and lower energy consumption. For this reason, in this article, a pulsed voltage source for starting an ele ctrostatic filter is investigated. The existing pulse structures have been investigated and by combining two of them, a new structure including the energy storage capacitor network and a magnetic switch to produce the required pulse has been presented. In this structure, a saturable inductor plays the role of a switch, which can overcome the voltage limitations of semiconductor switches and cover any voltage level. The proposed structure is analyzed and simulated for a specific example.
Keywords: pulse power, saturable inductors, magnetic switches, pulse magnetic compressor, electrostatic filters