فهرست مطالب
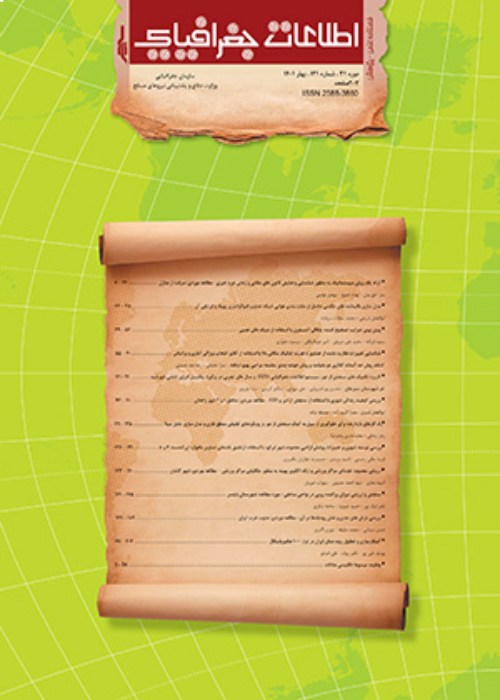
نشریه اطلاعات جغرافیایی (سپهر)
پیاپی 125 (بهار 1402)
- تاریخ انتشار: 1402/03/01
- تعداد عناوین: 10
-
-
صفحات 7-27
در این پژوهش آلودگی هوای ایجاد شده توسط کارخانه ذوب مس خاتون آباد و تعیین شعاع تاثیر آن با استفاده از داده های سنتینل 5 پی (Sentinel-5P) در سامانه گوگل ارث انجین مورد بررسی قرار گرفته است. داده های مربوط به میانگین گازهای دی اکسید سولفور و دی اکسید نیتروژن با استفاده از سامانه گوگل ارث انجین در محدوده 50 کیلومتری از کارخانه و در بازه ی زمانی یک روزه، هفت روزه، چهارده روزه، یک ماهه، دوماهه، سه ماهه، شش ماهه، دوازده ماهه و سی ماهه از ماه دسامبر2020 برای ارزیابی غلظت آلودگی در روزهای سرد سال و در بازه های زمانی مشابه از ماه ژوین 2021 به منظور ارزیابی غلظت آلودگی در روزهای گرم سال به دست آمد. تحلیل این داده ها برای تعیین بازه های زمانی موثر و میزان غلظت تجمعی آن ها با استفاده از روش آماری - مکانی تحلیل لکه داغ صورت گرفته است. نتایج حاصل از تحلیل لکه داغ تصاویر مربوط به گاز دی اکسید نیتروژن نشان می دهد که در بازه های زمانی دو هفته تا دو ماهه در ماه های سرد سال لکه داغ نشانگر وجود گاز دی اکسید نیتروژن در جو مستقر در بالای کارخانه است. اما در بازه های سه ماهه، شش ماهه، یک ساله و سی ماهه در ماه های سرد لکه داغ به سمت شمال غرب و در فاصله دورتر از کارخانه مشاهده می شود. همچنین تصاویر مربوط به ماه ژوین در اطراف کارخانه مورد مطالعه روند مشابهی را نمایان می سازد. نتایج حاصل از تحلیل لکه داغ تصاویر مربوط به گاز دی اکسید سولفور نشانگر مقدار زیاد غلظت این گاز در اطراف کارخانه است و تصاویر با بازه های زمانی یک ماهه و طولانی تر قادر به ارایه اطلاعات دقیق تر و منطقی تر در مورد میزان غلظت گاز دی اکسید سولفور هستند. باتوجه به نتایج به دست آمده فعالیت این کارخانه می تواند دلیلی بر افزایش میزان غلظت گاز دی اکسید سولفور باشد که شعاع حدود 4 تا 6 کیلومتری و مساحت حدود 10700 هکتار در اطراف کارخانه را تحت تاثیر قرار داده است. نتایج این تحقیق می تواند کارشناسان محیط زیست و محققین را در استفاده و تفسیر بهتر از داده های ماهواره سنتینل 5 پی در ارزیابی آلودگی هوا در مناطق صنعتی در بازه های زمانی متفاوت با درک بهتری از میزان پراکنش هر گاز در فصول گرم و سرد سال و در هر بازه زمانی یاری رساند.
کلیدواژگان: آلودگی هوا، سامانه گوگل ارث انجین، GIS، سنتینل 5 پی، کارخانه ذوب مس خاتون آباد -
صفحات 29-52
گسترش شهرها یک فرآیند پویاست و به روزرسانی نقشه های شهری به منظور ارایه خدمات بسیار مهم است. داده های سنجش ازدوری منبع قدرتمندی برای استخراج مناطق ساخته شده می باشند؛ یکی از داده هایی که می تواند پویایی مناطق شهری را تشخیص دهد داده های نور شب است. ازآنجایی که مناطق شهری در هنگام شب توسط نورهای مصنوعی موجود در منازل و خیابان ها روشن می شوند، درنتیجه به خوبی از پس زمینه متمایز خواهند شد. ازاین روی مطالعه حاضر در تلاش است رشد و گسترش مناطق شهری را با استفاده از نمونه های آموزشی با کیفیت و اتوماتیک از روی ترکیبی از تصاویر نور شب و اپتیک، در یک بازه 24 ساله با دقت بالایی شناسایی و استخراج کند. دراین راستا برای تولید نمونه های آموزشی با کیفیت، شاخص نور شب تحت عنوان [1]VTNUI توسعه داده شده است که با ترکیب ویژگی های مختلف بدست آمده از تصاویر لندست و نور شب در محدوده های شهری و در نظر گرفتن روابط بین مناطق، پدیده اشباع[2] و شکوفایی[3] تصاویر نور شب در محدوده شهری را کاهش داد. سپس با بررسی حد آستانه های خودکار بر روی شاخص توسعه داده شده نمونه های آموزشی با کیفیت تولید شد تا طبقه بندی دقیق تری از مناطق شهری ارایه شود. ازاین روی ابتدا از نمونه های آموزشی اولیه به دست آمده با استفاده از حد آستانه خودکار بر روی تصاویر نور شب طبقه بندی اولیه صورت پذیرفت سپس نمونه ها با اعمال حد آستانه خودکار بر روی شاخص معرفی شده پالایش شدند و طبقه بندی نهایی صورت گرفت. درنهایت بر اساس تحلیل سری زمانی، روند رشد منطقه بررسی شده است. به منظور بررسی اثربخشی روش پیشنهادی، دو منطقه دارای اقلیم متفاوت انتخاب شد و بررسی های مختلف بصری و کمی برای ارزیابی صورت پذیرفت. نتایج طبقه بندی نهایی برای بابل و کرمان به ترتیب با میانگین ضریب کاپا 0/93 و 0/74 و میانگین دقت کلی 97/76 و 87/63 برای تمام سال های مورد بررسی به دست آمده است. [1] vegetation and Temperature -NTL urban index[2] saturation[3] blooming
کلیدواژگان: داده نور شب، اشباع و شکوفایی، پویایی گسترش شهری، تصاویر ماهواره ای، نمونه های آموزشی خودکار -
صفحات 53-66
برنج اصلی ترین محصول غذایی بیش از نیمی از مردم جهان است. نظارت بر سطح زیر کشت محصول برنج، نقش مهمی در برنامه ریزی های کشاورزی دارد. امروزه می توان با تکیه بر فن آوری سنجش از دور و روش های یادگیری ماشین، روش های مدیریتی را بهبود بخشید. تحقیق فوق با هدف شناسایی برنج در سال 2020 به کمک نقشه های سری زمانی شاخص های NDVI و LST استخراج شده از تصاویر لندست-8، با الگوریتم SVM در ایالت کالیفرنیا، انجام گرفته است. یکی از انگیزه های اصلی این تحقیق، بررسی قابلیت های نقشه های سری زمانی شاخص LST در کنار نقشه های سری زمانی شاخص NDVI به منظور بهبود دقت شناسایی مزارع برنج ، با الگوریتم SVM است. در گام اول از روش پیشنهادی پس از اخذ سری زمانی تصاویر لندست-8 و انجام تصحیحات رادیومتری و اتمسفری، نقشه های سری زمانی دو شاخص NDVI و LST تولید شد. در گام دوم، شناسایی مزارع برنج با الگوریتم طبقه بندی SVM در دو سناریوی وجود یا عدم وجود نقشه ی سری زمانی LST در کنار نقشه ی سری زمانی NDVI پیشنهاد شد. در نهایت از الگوریتم های طبقه بندی نزدیک ترین همسایگی، درخت تصمیم گیری، رگرسیون لجستیک و پرسپکترون چند لایه برای مقایسه ی روش پیشنهادی استفاده شد. نتایج حاصل از شاخص پیشنهادی باعث بهبود دقت کلی به مقدار متوسط 3.572 درصد و ضریب کاپا به مقدار متوسط 7.112 درصد در روش های شناسایی مزارع برنج هنگام بکار گیری همزمان نقشه های سری زمانی شاخص LST و NDVI با کاهش خطای نوع اول به کمک استخراج ویژگی های فصل رشد حرارتی (حذف کلاس های غیر برنج همچون پنبه، لوبیای سبز و... از کلاس برنج) شد. همچنین الگوریتم ماشین بردار پشتیبان، بالاترین دقت کلی 94.28 درصد و ضریب کاپای 88.29 درصد را در شناسایی مزارع برنج از سایر مزارع کشاورزی، در مقایسه با سایر روش های مقایسه ای نشان داد. نتایج حاصل از روش های مقایسه ای کارآیی پایین الگوریتم درخت تصمیم گیری را در شناسایی لبه های مزارع برنج، نشان داد.
کلیدواژگان: شناسایی برنج، لندست-8، نقشه ی دمای سطح زمین، الگوریتم های یادگیری ماشین -
صفحات 67-80
امروزه در کشورهای در حال توسعه، منابع طبیعی به خصوص جنگل ها به منظور اهداف اقتصادی با شدت زیادی در حال بهره برداری هستند که این امر موجب تاثیر بر تنوع زیستی، خصوصیات خاک، کمیت و کیفیت آب و اقلیم جهانی می شود. بنابراین لزوم توجه بیشتر به حفظ و احیاء جنگل ها بیش از پیش احساس می شود. امروزه تکنولوژی استفاده از اطلاعات مکانی حاصل از سنجش از دور و تصاویر ماهواره ای، اطلاعات جامع و هدفمندی را برای مطالعات محیط زیستی فراهم کرده است. در این پژوهش نیز با استفاده از تصاویر ماهواره سنتینل2 که دارای قدرت تفکیک مکانی، طیفی و زمانی مطلوب می باشند، به بررسی و تعیین سطح، پراکنش و انبوهی جنگل های منطقه ارسباران و سایر کاربری ها پرداخته شد. جنگل های ارسباران در ارتفاعات قره داغ در شمال استان آذربایجان شرقی قرار گرفته است ومساحت منطقه مورد مطالعه 551211 هکتار می باشد. طبقه بندی منطقه به کاربری جنگل(انبوه، نیمه انبوه، تنک و خیلی تنک)، مرتع، کشاورزی، مسکونی و بایر، باغ و آب با استفاده از الگوریتم های مختلف انجام شد که نتایج نشان داد روش طبقه بندی نظارت شده حدکثر احتمال، بهترین روش برای طبقه بندی جنگل های ارسباران است. بر اساس نتایج به دست آمده مساحت جنگل های با تراکم بیشتر از 5 درصد،131019 هکتار محاسبه است که 39 درصد آن مربوط به جنگل انبوه، 36 درصد نیمه انبوه، 17 درصد تنک و 8 درصد خیلی تنک می باشد. در منطقه مورد مطالعه بیشترین سطح کاربری به ترتیب مربوط به مرتع، جنگل، کشاورزی، مسکونی و بایر، باغ و آب محاسبه شده است. با ارزیابی و تعیین صحت نقشه نهایی، ضریب کاپا 0.88 و صحت کلی 89.8% به دست آمد که این نتایج نشان داد روش طبقه بندی نظارت شده پیکسل پایه، روشی قابل قبول بوده و نقشه به دست آمده قابل اطمینان و کاربردی می باشد. نتایج حاصل از این تحقیق می تواند در تهیه برنامه های کوتاه مدت، میان مدت و بلندمدت مدیریت پایدار جنگل های کشور استفاده شود که منجر به شناسایی اراضی تخریب شده و جلوگیری از تعرض به اراضی منابع ملی جنگلی خواهد شد و همچنین به عنوان ابزاری مناسب برای برنامه ریزی و تصمیم گیری برای آینده جنگل ها به کار رود.
کلیدواژگان: جنگل ارسباران، طبقه بندی نظارت شده، تصاویر ماهواره ای سنتینل2، کاربری زمین، انبوهی جنگل -
صفحات 81-100
با گسترش تکنولوژی لیزر اسکنر و لایدار و در نظر گرفتن برتری های این تکنولوژی و اخذ داده های مکانی محیط و اشیاء، استفاده از این فناوری روز به روز بیش تر شده است. این تکنولوژی توانایی استخراج نقاط از سطوح خارجی محیط و اشیاء در حجم بالا و در مدت زمان کوتاه را داشته که با توجه به امکان جا به جایی آسان لیزر اسکنر ها، ابر نقاط مربوط به محیط و اشیا معمولا از زوایای متفاوتی اخذ می شوند که هر کدام در یک سیستم مختصات متفاوت قرار دارند و همگی باید به یک سیستم مختصات واحد منتقل شوند. به این منظور ابتدا باید جفت نقاط متناظر در هر مجموعه ابر نقاط معین شوند و سپس با استفاده از فرآیندی نقاط متناظر روی هم بیافتند تا مدل سه بعدی از طریق مجموعه های ابرنقاط ایجاد شود. یافتن مناسب ترین جفت نقاط متناظر در مجموعه های ابر نقاط از مهم ترین و چالش بر انگیزترین مراحل بازسازی سه بعدی مدل های مورد نظر محیط و اشیاء است. توصیفگر های سه بعدی از جمله ابزار های مناسب برای تعیین جفت نقاط متناظر در مجموعه های ابر نقاط هستند. این توصیفگر ها برای هر نقطه ی منفرد در ابر نقاط، مجموعه ای از اطلاعات را به منظور کمک به تعیین نقاط مشابه در هر مجموعه ابرنقاط می سازد. تعریف یک توصیفگر سه بعدی که از پیچیدگی محاسباتی کم و قدرت توصیفگری بالایی برخوردار باشد، می تواند به یافتن جفت نقاط متناظر صحیح و در ادامه مدل سازی سه بعدی مجموعه های ابرنقاط کمک شایانی کند. در این پژوهش یک توصیفگر سه بعدی هندسی-مکانی پیشنهاد داده شده است که از هر دو ویژگی هندسی و مکانی به صورت توام استفاده می کند. با توجه به آزمایش های انجام شده بر روی دو دسته مجموعه داده با ساختارهای متفاوت، توصیفگر سه بعدی پیشنهادی در مقایسه با توصیفگر هایی که تنها از یک ویژگی مکانی یا هندسی استفاده می کنند، قدرت توصیفگری بالاتری دارد.
کلیدواژگان: ابرنقاط سه بعدی، انطباق، توصیفگر، ویژگی هندسی، ویژگی مکانی -
صفحات 101-119
صخره های مرجانی ازنظر محیط زیستی جزء مهم ترین مناطق جهان هستند. در چند دهه اخیر این بوم سازگان با افزایش دمای اقیانوس ها به شدت توسط رویداد سفید شدن تهدید می شوند. با سفید شدن صخره های مرجانی این بوم سازگان تحت تنش بیشتری قرارگرفته و در معرض مرگ ومیر قرار خواهند گرفت. امروزه به منظور پیش بینی و شناسایی مناطق در معرض خطر سفید شدن مرجان ها از داده های مبتنی بر سنجش ازدور ماهواره ای و قابلیت های تحلیل روند استفاده می شود. در این پژوهش سعی شد با استفاده از روند داده های 35 ساله در بازه زمانی 1980 تا 2015 در قالب داده های چندبعدی، دمای سطح دریا در سال 2022 با به کار گیری ابزارهای ArcGIS Pro برای محدوده خلیج فارس پیش بینی شود و مناطقی که بیشتر در معرض تنش حرارتی منجر به سفید شدگی هستند شناسایی شوند. در این تحلیل برای برازش خط روند از روش هارمونیک استفاده شد. خط روند هارمونیک خطی منحنی است با تکرار دوره ای که بهترین استفاده را برای توصیف داده های دارای الگوی چرخه ای دارد. نتایج اولیه نشان داد که دمای سطح دریا در محدوده خلیج فارس از سال 1996 میانگین دمای بالاتری را تجربه کرده است. نقشه روند به دست آمده (1980 تا 2015)، بیانگر این بود که مناطق شمال غربی خلیج فارس و بخشی از جنوب آن بیشتر در معرض گرمای طولانی قرار دارند. دقت برازش روند داده ها توسط تابع رگرسیونی هارمونیک، پارامترهای آماری، 0.78= R2 و0.5 =RMSE را ارایه داد. در این مطالعه، ناهنجاری های موجود در داده ها محاسبه شد و مناطقی که دمای بالاتر از میانگین داشتند شناسایی شدند. در برش زمانی سالانه پیش بینی شده (2022)، شمال غربی و بخشی از جنوب منطقه خلیج فارس با تنش حرارتی منجر به سفید شدگی مواجه می شوند. نتایج به دست آمده با نقشه های سازمان نووآ در همان تاریخ مقایسه شدند و مورد تایید قرار گرفتند. پیشنهاد می شود تا سازمان های مسیول برای ارزیابی وضعیت و تهیه نقشه خطر محدوده های مرجانی از روش های مبتنی بر سنجش ازدور و تحلیل روند استفاده کنند.
کلیدواژگان: سفید شدن مرجان ها، تحلیل روند هارمونیک، سنجش ازدور، دمای سطح دریا، خلیج فارس -
صفحات 121-141
بی شک انگیزه ی اصلی تمام برنامه ریزی ها رسیدن به توسعه پایدار، تعادل منطقه ای، توزیع مناسب فعالیت ها و استفاده حداکثر از قابلیت های محیطی در فرایند توسعه مناطق می باشد. مهمترین ابزار برنامه ریزی در توسعه پایدار و آمایش سرزمین، اطلاعات مکانی است و مدیریت اطلاعات مکانی از درگاه زیرساخت ملی اطلاعات مکانی و با بهره گیری از فناوری های پیشرفته، به بالاترین سطح بهره وری خواهد رسید. اقدام در جهت به روز رسانی روش ها، ابزارها و قالب های مدیریت اطلاعات مکانی، رسالت مهمی بنظر می رسد. بگونه ای که مدیران در فرآیند تصمیم سازی، بتوانند از اطلاعات مکانی بهره برده و در مسیر پیشبرد اهداف توسعه پایدار، قدم بردارند. این پژوهش بر پایه مطالعه روش ها و فناوری های روز در مدیریت جامع اطلاعات مکانی سه حوزه پیشرو جهان شامل ایالات متحده آمریکا- استرالیا و اتحادیه اروپا بنا شده است. زمینه های مطالعاتی شامل رویکرد سیاسی و اقتصادی حاکمیت- فناوری های نوین- معماری و ساختار و نهایتا قوانین و محدودیت ها می باشد. هدف این تحقیق، تهیه نقشه راه برازش وضعیت موجود در مدیریت اطلاعات مکانی کشور با وضعیت مطلوب و دستیابی به توسعه مکان محور از سریع ترین مسیر ممکن است. نتایج این تحقیق نشان می دهند که بازطراحی سیستم زیرساخت اطلاعات مکانی کشور با رویکرد تکاملی، نیازمند سرمایه گذاری و تلاش جدی کلیه دست اندرکاران این حوزه می باشد و با تهیه نقشه راه عملیاتی و حرکت زمان بندی شده در این راستا و همچنین، بومی سازی دستورالعمل های موجود، می توان به فناوری های روز و معماری بهینه دست یافت واز نتایج آن که تحقق توسعه پایدار و عدالت منطقه ای و بازگشت چندین برابر سرمایه است، بهره مند شد.
کلیدواژگان: Digital Twin، NSDI، آمایش سرزمین، توسعه پایدار -
صفحات 143-161
شهر مشهد در پهنه ای با میزان آسیب پذیری بالا قرار گرفته است که وجود 3894 هکتار سکونتگاه غیررسمی در این شهر، بر میزان این آسیب پذیری افزوده است. لذا در این پژوهش، با رویکرد توصیفی_تحلیلی و با استفاده از روش WLC، FUZZY و AHP ابتدا میزان تاب آوری محلات حاشیه نشین شهر مشهد ارزیابی شده است و سپس با استفاده از آمار فضایی، الگوی تاب آوری در این محلات مورد ارزیابی قرار گرفته است. نتایج حاصل از کاربرد شاخص موران در توزیع فضایی تاب آوری اجتماعی، نشان می دهد که این ضریب مثبت و برابر 18/0است. در واقع این مقدار، خوشه ای بودن توزیع فضایی تاب آوری اجتماعی در محلات حاشیه نشین شهر را نشان می دهد. تحلیل آماره G نشان می دهد که خوشه های با تاب آوری اجتماعی بالا درکنار یکدیگر قرار دارند و از الگوی خوشه ای باتمرکز بالا پیروی می کنند. یعنی بلوک هایی با ناکارآمدی زیاد یا کم در مجاورت یکدیگر قرار دارند. خوشه های گرم و نسبتا گرم حدود 41 درصد از مساحت کل محلات حاشیه نشین را شامل می شوند که 31 محله از شهر مشهد را تشکیل داده اند. همچنین مدل سازی عوامل موثر بر تاب آوری اجتماعی محلات دارای بافت ناکارامد شهر مشهد با استفاده از رگرسیون وزنی جغرافیایی (GWR) نشان داد که متغیرهای درصد جمعیت شاغل، درصد جمعیت فعال و میانگین سنی بر تاب آوری اجتماعی محلات مورد مطالعه اثرگذاری بیشتری دارند. از این رو می توان این متغیرها را مبنای علمی برای افزایش تاب آوری اجتماعی این محلات در روند احیای آن ها دانست. به گونه ای که با ایجاد فرصت های شغلی و جذب جمعیت فعال و جوان، در راستای افزایش تاب آوری اجتماعی محلات گام برداشت.
کلیدواژگان: تاب آوری، سیستم پشتیبانی تصمیم فضایی چندمعیاره، خودهمبستگی فضایی، رگرسیون وزنی جغرافیایی -
صفحات 163-177
پدیده جزیره حرارتی شهری، از معمول ترین پدیده های شهری است که طی آن برخی از نقاط شهری به خصوص مرکز شهرها چند درجه از مناطق اطرافشان گرم تر می شوند. هدف این پژوهش، ارزیابی روند تغییرات دما، تغییرات جزیره حرارتی و پوشش گیاهی دوره گرم سال در شهر یزد می باشد. برای بررسی روند تغییرات دمای ماه های گرم سال، از اطلاعات دمایی ایستگاه یزد و آزمون من-کندال استفاده شد. به منظور تعیین جزیره گرمایی شهر یزد در ماه های گرم سال، از تصاویر ماهواره ای لندست 4-8 در محدوده زمانی33 ساله(2020-1987) که شامل چهار دوره با فاصله زمانی 11 ساله است (میانگین ماه های تیر، مرداد و شهریور سال های 1987، 1998، 2009 و 2020) استفاده شد. نتایج حاصل از تصاویر ماهواره ای نشان داد که در طی دوره آماری هر چه به سال های جاری نزدیک تر شویم از میزان پوشش گیاهی و زمین های بایر کاسته شده و در عوض به وسعت ناحیه ی شهری افزوده شده است. نقشه های LST نیز نشان از دماهای بالای 50 درجه سانتی گراد به صورت خوشه های داغ در قسمت های غرب و جنوب غرب شهر یزد بوده که در دهه های اخیر ایجاد و تقویت شده اند. آزمون من-کندال و شیب سنس نیز حاکی از روند صعودی معنی دار در سطح اطمینان 99 درصد به خصوص در سال های اخیر است. میزان این افزایش دما دریک دوره 45 ساله حدود 2.3 درجه سانتی گراد بوده است که این شرایط می تواند در پیوند احتمالی با پدیده روند افزایش جمعیت شهری طی دهه های اخیر و به دنبال آن افزایش سازه های شهری(مسکونی، تجاری) و پدیده جزیره حرارتی مرتبط باشد.
کلیدواژگان: جزیره حرارتی، دمای سطح زمین، تغییرات کاربری، NDVI، شهر یزد -
صفحات 179-192
پایش و بررسی تغییرات کاربری اراضی در عرصه های جنگلی، اطلاعات قابل قبولی را به منظور مدیریت کارآمد این منابع فراهم می کند. همچنین حفاظت از منابع طبیعی نیازمند آگاهی از شرایط و نحوه تغییر کاربری های مختلف اراضی است؛ بنابراین هدف از این پژوهش ارزیابی روند تغییر کاربری جنگل در محدوده جنگلی فندقلو از سال 2010 تا سال 2019 با استفاده از تصاویر لندست 5، 8 و ادغام آن ها باتصاویر سنتینل2 و استر است. پس از تهیه تصاویر برای سال های 2010، 2015 و 2019، تصحیح هندسی، رادیومتریک و اتمسفری تصاویر انجام گرفت و نقشه کاربری ها با دقت کاپا به ترتیب 93، 83، 91 درصد تهیه شدند. مدل سازی تغییر کاربری برای سال 2025 با مدل GEOMOD، نیازمند تهیه نقشه تناسب منطقه است که با استفاده از روش Fuzzy ANP و ضریب ناسازگاری کمتر از 0.06 تهیه شده و برای تهیه نقشه تناسب اراضی از چهار معیار: انسانی، زیست شناختی، توپوگرافی و اقلیمی و 11 زیر معیار با توابع بولین بدست آمد و نقشه های کاربری اراضی بولین (جنگل و غیر جنگل) 2010 و 2015 مدل سازی برای سال 2019 انجام گرفت. مدل سازی کاربری برای سال 2025 از نقشه پایه 2019 و ماتریس انتقال زنجیره مارکوف کاربری اراضی 2025 با مدل CA- Markov صورت پذیرفت و نتیجه تغییرات مکانی برای سال 2025 به دست آمد. برای ارزیابی دقت مدل از میزان توافق و عدم توافق مکانی پیکسل ها با Klocation و Standard به ترتیب با دقت 98 و 95 استفاده شد. نتایج مدل سازی برای سال 2025 تغییرات به صورت کاهش پوشش جنگل بوده که از 3204.18 هکتار در سال 2010 به 3070.55 هکتار در 2019 هزار هکتار کاهش یافته است. با توجه به نتایج وزن دهی، معیار انسانی و زیرمعیارهای کاربری اراضی و فاصله از جاده وزن بالایی را به دست آوردند. علت آن می تواند پتانسیل گردشگری این منطقه در جذب گردشگران و همچنین دخالت های ساکنان محلی باشد که تاثیر مستقیمی بر روند کاهشی جنگل دارد.
کلیدواژگان: مدل سازی تغییر پوشش جنگل، CA مارکوف، GEOMOD، فندقلو
-
Pages 7-27Introduction
Air pollution is one of the most crucial environmental problem in the glob and its impact on human live and ecosystem is undeniable. The International Agency for Research on Cancer introduced air pollution as one of main causes of cancer. Therefore, by monitoring air pollution would be a necessity in industrialized cities. Air quality index include evaluation of the amount of NO2, SO2, O3, CO and Aerosol in the air. As, ground station has limited ability to assess the amount and distribution of these harmful gases in the urban and rural areas, therefore, remote sensing technology become a popular tool in assisting research to shed light on this subject. The current study evaluates air pollution caused by Khatoonabad Copper Smelting Factory using Sentinel P5 satellite images.
Materials & MethodsThis research investigates the air pollution created by Khatoonabad Copper Smelting Factory and determines its impact radius, using Google Earth Engine system and Sentinel P5 satellite images. Khatoonabad Copper Smelting Factory is located in the northwest of Kerman province at the latitude of 29 Degree 59 Minute to 30 Degree 32 Minute and longitude of 54 Degree 52 Minute to 55 Degree 55 Minute. By performing the coding operation in the Google Earth Engine system, the images related to the average air pollution for So2 and No2 in the area of 50 km from the factory and in a period of 30 months from 07/04/2018 to 12/30/2021 were obtained. The amount and distribution of pollutants were examined based on one-day, seven-days, fourteen-days, one-month, two-months, three-months, six-months and twelve-months’ time periods from December 2020 to assess the concentration of pollution in the cold months of the year, also for the same time periods from June 2021 to assess the concentration of pollution in the warm months of the year.
In order to map distribution of each pollutants, Natural Break Classification and Hot Spot Analysis methods were performed on the images obtained from Google Earth Engine in GIS. Natural Break Classification method is based on Jenk optimization and classify spatial data based on statistical properties of each input where variances between classes maximize. Hot Spot Analysis methods is a spatial and statistical method that consider spatial autocorrelation among the spatial data to classify the data according to statistical significance of each class. Points that surrounded by high values and they are statistically significant called hot spot and areas that are surrounded by low values and have high negative Z score and low P values ( P value < 0.05) are called cold spot.Results & DiscussionThe results based on an averaged image for the period of 30 months indicated that the amount of So2 from 0.0000987 to 0.000698 (mol/m2) and the amount of No2 from 0.00005854 to 0.00006932 (mol/m2) in the study area that by increasing the distance from the factory, the amount of So2 and No2 decreased. Furthermore, analyzing the average amount of So2 and No2 in different period of daily, weekly, two weeks, and monthly have showed dispersed spatial distributions in warm and cold season of the year. Therefore, Sentinel 5P data in short-term periods such as daily, weekly, two-week and even one-month cannot provide accurate information on the spatial distribution of No2 and So2 in the study area.
In the data obtained from the two-month, three-month, six-month and one-year intervals, the amount of sulfur dioxide concentration has less dispersion than the short-term intervals, and as the time interval increases, the images show less dispersion of sulfur dioxide gas in polluted areas. Therefore, the obtained results indicate that Sentinel 5P images with longer time intervals of two months are able to provide more accurate and logical information about the concentration of sulfur dioxide gas in the area. However, in case of nitrogen dioxide, the imaged longer than two weeks can provide accurate information regarding the spatial distribution of this pollutant in the area.
Hot spot analysis was also performed on the images obtained in one-day, seven-day, fourteen-day, one-month, two-month, and three-month intervals from June in order to investigate the concentration and dispersion of pollution in the hot days of the year. Then the maps obtained from the hot months were compared with the maps of the same period from the cold months of the year. This comparison showed that in the maps obtained from the short-term intervals related to the hot months of the year, the density of hot spots was more observed in areas prone to the presence of sulfur dioxide gas. For example, the one-day image from December showed a lot of dispersion, while the one-day image from June indicated less dispersion and more density of gases in polluted areas. In addition, in the one-week, two-week and one-month maps from December hot spots and cold spots show much greater dispersion compared to similar maps in the same periods from June. However, by comparing the two-months and three-months hot spot maps of the cold months to the same maps of the hot months of the year, almost similar results were obtained, even more density were observed in the hot spot map of longer periods (more than two months) in winter time. The same trend happened by analyzing nitrogen dioxide in the studied area.
ConclusionThe results obtained from the classification of images related to sulfur dioxide gas showed that the concentration of sulfur dioxide gas in the area around the desired factory has the highest concentration value and as the distance from the factory increases, the concentration of sulfur dioxide gas decreases. Also, according to the minimum and maximum concentration of sulfur dioxide in the studied area, it is concluded that more sulfur dioxide is observed in the cold months of the year than in the warm months of the year. However, in the cold months the concentration of sulfur dioxide has a greater range of changes than the hot months of the year.
According to the results, the dispersion of sulfur dioxide concentration in short time intervals such as daily, weekly, fortnightly and even one month was very high in these time intervals. As a result, Sentinel 5P images are not able to provide logical and accurate information about the distribution of atmospheric sulfur dioxide concentration in daily, weekly, two-week and one-month intervals. In order to obtain accurate and logical information, images with time intervals longer than one month should be used, and the longer the time interval is, the more reliable the results will be.
The results of the hot spot analysis of the images related to sulfur dioxide concentration also indicated a high concentration of sulfur dioxide gas in the area around the factory. According to the obtained results, the activity of the studied factory can be a reason for the increase in the concentration of sulfur dioxide gas in this area, which has affected a radius of about 4 to 6 kilometers and an area of about 10,700 hectares around the factory.
The results obtained from the classification of images related to nitrogen dioxide gas show that the concentration of nitrogen dioxide in the area around the factory has a higher limit. According to the minimum and maximum concentration of this gas in the study area, it can be concluded that in the hot months of the year, the concentration of nitrogen dioxide gas is higher than in the cold months of the year. Considering the rapid spread of nitrogen dioxide gas in the atmosphere by the wind due to the high dynamics of this gas (Vîrghileanu et al., 2020), it can be concluded that the images obtained from the time intervals of two weeks of more can provide more information about the concentration of nitrogen dioxide in the atmosphere.
The results of the hot spot analysis of the images related to nitrogen dioxide gas showed that in the time intervals of two weeks to two months in the cold months of the year, there are hot spots that indicated the presence of nitrogen dioxide gas in the atmosphere located above the factory. However, in long-term intervals such as three months, six months, one year and thirty months, in the cold and hot months, hot spots are observed towards the northwest and at a distance from the factory.
The result of this research can assist environmentalist and researchers in using and interpreting Sentinel 5P data by considering different periods in cold and warm seasons for making informed decisions.Keywords: Air pollution, Google Earth Engine, GIS, Hot spot analysis, Sentinel P5, SO2, NO2, Khatoonabad, Kerman -
Pages 29-52Introduction
Urbanization can be defined as social and economic development and thus, urban planners need timely information for service provision and management in urban areas. Due to the difficulties of traditional methods, automatic and semi-automatic methods have gained special importance. Therefore, remote sensing data and image classification techniques have been used to help identify different types of land use. Nighttime light emission data can help researchers effectively identify human activities and urban areas. These satellite images are collected from the surface of the earth at night and can clearly separate nighttime light emission of urban areas from the surrounding dark areas. Thus, it can be concluded that various types of data with different nature and capabilities (spectral, nighttime light, etc.) are available for any specific area each of which has its own advantages and limitations. As a result, using a combination of these data types will increase accuracy and reduce uncertainty. Algorithms and scientific methods enabling this combination are thus of great importance. The present study applies a combination of nighttime light emission and daytime multispectral images to produce automatic and high-quality optimal training samples and locate built-up areas.
Material & MethodsTwo study areas (in Babol and Kerman) with two different climates have been investigated in the present study. Also, DMSP and VIIRS nighttime light emission images and Landsat 5, 7 and 8 images collected during the statistical period have been used.
Research MethodsThe present study has proposed an approach consisting of four main phases of pre-processing, feature extraction and production of initial training samples, selection of optimal training samples and finally classification and evaluation. Nighttime light emission images were corrected and primary samples including two classes of built and unbuilt areas were produced using the limit of automatic thresholds. Nighttime light emission is generally related with human activities, and thus, built-up areas usually have a higher nighttime light emission value compared with unbuilt areas which have a lower or zero value. Due to the saturation and blooming problems occurring in DMSP images and the relatively low spatial resolution of nighttime light emission data, training samples extracted from built areas using these data still include unbuilt areas such as water bodies and vegetation cover. Therefore, an index has been developed using features extracted from nighttime light emission and Landsat images. Considering the inverse relationship between various features of urban and rural areas (vegetation cover and soil) in LST images obtained from the thermal band of Landsat images and the NDVI vegetation index obtained from Landsat and features of urban areas in nighttime light emission image, an index was provided which maintains the main characteristics of urban areas in nighttime light emission images while minimizing saturation and blooming. Finally, time series of classified images was investigated and urban expansion was analyzed.
Result & DiscussionFollowing nighttime light emission data correction, an upward trend was observed for the values of pixels collected from each city which verifies the pre-processing stage. Then, an appropriate automatic threshold limit was selected in accordance with the features of each nighttime image and applied to produce the initial training samples. Nighttime light emission images were corrected using the introduced index to minimize saturation and blooming in urban and suburban areas. Training samples thus optimized were used for final classification. Due to the low quality of initial training samples, classified pixels obtained from urban areas did not confirm to reality. Thus, classification faded in Kerman city in some years and practically no classification was performed which shows the low quality of initial training samples. Due to the low spatial resolution of nighttime light emission images, the size of samples collected from built-up areas was falsely detected to be large, and thus, there were definitely samples related to vegetation, soil, and etc. in the specified range. In the next step, classification was performed using optimal training samples in which built-up regions were modified. In this way, results got closer to the reference data and reality. In fact, using a combination of nighttime light emission and Landsat data can overcome the limitations of both methods.
ConclusionSelection of training samples is considered to be the main and fundamental challenge of classification. With a valid training sample, classification is precisely performed. Since, traditional and manual methods of obtaining training samples are costly and time-consuming, automatic and semi-automatic methods have become specifically important. Therefore, the present study has classified and extracted built-up areas using satellite images. The initial training samples can be obtained automatically from nighttime light emission images, however high saturation and blooming of these images have reduced their quality. To solve this problem, a nighttime light index has been developed based on the relationship between the characteristics of urban areas in optical images and nighttime light emission images which has minimized related problems in both study areas with two different climates to a great extent. This shows the flexibility and effectiveness of the proposed method. High-quality training samples thus obtained were highly effective in the final classification phase. Investigating urban expansion time series has shown that urban growth and expansion have generally occurred around the city.
Keywords: Nighttime light emission data, saturation, blooming, automatic training samples, urban built-up areas -
Pages 53-66Introduction
Rice is an important crop and the main food of more than half of the world’s population, which needs water and heat to grow. Thus, mapping and monitoring rice fields with efficient means such as remote sensing technology is necessary for food security and the lack of water sources. The phenology extracted from the time series of vegetation indices is used for monitoring and mapping the area under rice cultivation. In addition to the phenological curve, the LST time series map, which is calculated from Landsat 8 images and is related to the phenomenon of evaporation and transpiration of irrigated crops, can cause the separation of rice cultivation from rainfed crops, summer crops, water, etc. Therefore, in this study, the effect of the LST time series map is investigated map for improving the accuracy of rice field identification.
Materials & MethodsSince the planting to harvest period of rice is from May to October, in this study, the time-series maps of LST and NDVI for the 3rd of April, 21st of May, 6th of John, 22nd of John, 8th of July, 24th of July, 9th of August, 12th of October, and 28th of October have been calculated after download the Landsat-8 time-series in 2020 The ground truth map of the study area has been obtained from the US Department of Agriculture. To identify rice fields and calculate the LST and NDVI using the Landsat-8 images, initial pre-processing including radiometric and geometric corrections has been applied to these images first. After initial corrections and the calculation of NDVI and LST maps, to identify rice fields in the study area, machine learning algorithms such as Support Vector Machine, K-Nearest Neighborhood, Multilayer Perspective, Logistic Regression, and Decision Tree, have been proposed.
Results & DiscussionThe results of the proposed method at the state of California showed that using the time series map of Land surface temperature (LST) with the time-series map of Normalized Difference vegetation Index, improved the results of identifying rice fields (the average Overall Accuracy= + 3/572% and the average kappa coefficient= +7/112%). Visual results showed that some cultivation such as tomato, corn, cucumber, fallow, and water were removed from the rice final map when using the LST time-series map with the NDVI time-series map. According to the numerical results, the Support Vector Machine algorithm (Overall Accuracy 94/28 and Kappa Coefficient 88/29), the Multilayer Perceptron algorithm (Overall Accuracy 94/26 and Kappa Coefficient 88/21), and the K-Nearest Neighborhood algorithm (Overall Accuracy 93/71 and Kappa Coefficient 87/08) showed the highest Overall Accuracy and Kappa Coefficient compared to the Logistic Regression algorithm (overall accuracy 91/96 and kappa coefficient 83/54) and the Decision Tree algorithm (Overall Accuracy 91/34 and Kappa Coefficient 81/97), respectively.
ConclusionAlthough, many methods have been proposed to identify rice fields from satellite images. But, the similarity of rice class with other classes is one of the main challenges related to rice identification. In this research, the effect of LST time series maps to improve the identification accuracy of rice fields in Landsat-8 time-series images was investigated. In this study, the effect of the time series map of land surface temperature index extracted from Landsat-8 images on improving the accuracy of identifying rice fields from other rice fields due to the evapotranspiration process using machine learning algorithms was investigated. The results showed the effectiveness of the proposed index in improving the identification accuracy of rice fields. One of the reasons for improving the accuracy of identifying rice fields is to extract the characteristics of the thermal growing season from the Earth's surface temperature time series (LST) maps along with the rice phenology curve. The results showed that due to the flooding of rice fields when using the NDVI time series map, water class and fields summer crops were identified as rice class. But, water and summer crops classes were removed from the rice final map using a land surface temperature time-series map with the extraction of thermal growth season characteristics. Therefore, the results showed that there was a direct relationship between LST time-series maps and rice cultivation.
Keywords: Rice identification, Landsat-8, Land Surface temperature map, Machine learning algorithms -
Pages 67-80Introduction
Nowadays, natural resources are exploited for the purpose of economical development in developing countries. Expansion of agricultural lands, supply of charcoal and fuel wood and wood production play an important role in forest degradation which affects biodiversity, soil conservation, the quantity and quality of water and the global climate conducted to the importance of forest conservation and reforestation. Therefore quantitative assessment of forests is required for conservation programs and forest monitoring is defined as a tool for sustainable forest management.Today, remote sensing techniques and satellite images can widely provide functional information in environmental studies. In this work, sentinel-2 satellite images with high spatial, temporal and spectral resolution were applied to determine the area, distribution and density of the Arasbaran forestsas well as other land use classes in the area.
Materials and MethodsArasbaran area is located in Qaradagh mountainous region inthe north of East Azerbaijan province between 38°25′ 59 " N- 39°20′ 7.7 " N latitude and 46°09′ 18 " E- 47°16′ 5.3 " E longitude which covers an area of 551211 hectares and the deciduous forests of this area are known as the 11th Biosphere Reserved in Iran. The altitude varies from ca. 256 m tomore than 2000 m. the importance of the area is in having a rich flora (about 1334plant species) and unique vegetation among the vegetation of the country.
For the first time, the Sentinel-2 images with a combination of high spatial and temporal resolution were used to classify the land use of the area.The best band combination was found for bands 2, 3, 6, 12 and NDVI index. Land use classification included dense, semi -dense, sparse and very sparse forests as well as rangeland, agriculture, residential area-bare soil, garden and water was implemented using 9 different algorithms in a pilot area to find the best algorithm. 280 training sample points were collected from all different land use classes in the area.Consequently, supervised classification technique and Maximum Likelihood algorithm with the Kappa coefficient of 0.886 and anoverall accuracy of 89.6% was identified as the best classification method for the Arasbaran area.Accuracy assessment of the final map was done using ground control points and Google earth images with a total accuracy of 95%.Finally creating an error matrix with 880 ground reference test pixels revealed the accuracy indices.ResultsThe final land use map of the Arasbaran area based onthe Supervisedclassification technique and Maximum Likelihood algorithm was created.Based on the results, the accuracy assessment of the final map showed that the Kappa coefficient and the overall accuracy of the classified map were 0.88 and 89.8% respectively.The forest distribution and canopy cover density map were extracted from the land use area map. The total area of forests with a canopy cover of more than 5%, obtained 131019 ha consisting of 39% dense forest, 36% semi -dense forest, 17% sparse forest and 8% very sparse forest. In addition, the largest type of land use accounted for rangeland with 270000, forest with 131019, agriculture with 101974, residential area-bare soil with 30028, garden with 15434 and water with 2756 hectares respectively. Based on the error matrix table and correct classified points as well as total ground control points, the highest user’s and producer’s accuracy belonged to the densed forest class as well as the lowest user’s accuracy and lowest producer’s accuracy belonged to garden and agriculture classes respectively.
ConclusionThe results conducted supervised pixel-based image classification based on the Maximum Likelihood algorithms an acceptable method. It can be because of well -distributed training sample points, the high spatial resolution of sentinel-2 images or Environmental heterogeneity of the area. According to the results, dense forests declined(from 56910 to 50628 ha)however semi -dense and sparse forests have increased (from 35280 to 47930 ha)with respect tothe last forest survey project in the Arasbaran area in 2003.In addition, the results revealed an overlap between agriculture and garden as well as rangeland and residential area-bare soil classes because of multi culture of crops and fruit trees together as well as dried or low vegetation cover of rangelands in the area. These results can provide useful information for decision- making and sustainable forest management for reducing forest degradation and it seems to be an important next step to manage these forests based on conservation policies.
Keywords: Arasbaran forest, Supervised classification, Sentinel2 satellite image, Land use, Forest density -
Pages 81-100Introduction
Reconstruction of 3D models and their use in photogrammetry and remote sensing has been considered as the most important and challenging topics in recent years. With the development of laser scanner technology and obtaining spatial data of the environment and objects, the use of this technology has increased nowadays. This technology extracts points from the external surfaces of the environment or objects in high volume, in a short time, which is called point cloud.
Due to laser scanners’ easy placement, point clouds are usually taken from different angles, so they define in the different coordinate systems, which must be unified to give a complete 3d view of the object. The process is considered as “registration”.
For this purpose, first, the corresponding pairs of points in each point cloud must be determined and then they must be matched correctly.after all a three-dimensional model is created.
Finding the best pair of corresponding points in the Point clouds as well as estimating the optimal error metric and the displacement between pairs of corresponding points is one of the most important and challenging steps of three-dimensional reconstruction.
Three-dimensional descriptors are one of the most suitable tools for determining the corresponding pairs of points in Point cloud. These descriptors create a set of information for every single point to determine the corresponding points in each Point cloud. Defining a three-dimensional descriptor whose computation complexity is low but its descriptive is high, can help to find the correct pair of points for 3d registration and modeling.Materials & MethodsThe main purpose of the present study is to define a strong three-dimensional descriptor to find the best corresponding pair of points to reconstruct the three-dimensional model.
The descriptor proposed in this study consists of two single local three-dimensional descriptors based on the spatial and geometric properties of the Point cloud, which combine to form a strong descriptor to determine corresponding points in the Point cloud.
Laser scanners extract a large volume of points from surfaces in a short period of time, which due to the reflection of laser beams, Point cloud may contain noise and mistakes. In the process of analyzing and using the data, these mistakes cause problems and should be removed in the pre-processing phase. To define the desired descriptor, in the pre-processing phase the Point cloud gets ready to extract the required properties.
The Statistical removal filter method is used to remove the noise and the voxel grid filter method is used to improve the speed of future preprocessing.
Each point in the neighborhood of Query Point provides a lot of that can be used to create the desired descriptor.
In the present study, by determining the appropriate neighborhood radius and Nearest Neighbor Search (NNS) method, using the k-dimensional tree, correct and efficient neighborhoods are determined for each point.
In the first step, a spatial descriptor is formed for each point. This descriptor is defined in the form of a histogram based on two distances for the point in its neighborhood. In the second step, the angles of the normal vectors of the Point cloud in different states are used to create a descriptor based on geometric information. In this research, two features called and have been used, which for each descriptor is formed in the form of a histogram. Then the spatial descriptor is combined with each of the descriptors based on the geometric feature and forms two desired descriptors.
To ensure the accuracy of the matching process based on the proposed descriptor, by assigning a suitable threshold for the basis of the distance between the Query point and its neighborhood, with the corresponding point of the Query point and its neighborhood in the second Point Cloud, incorrect correspondences are detected and removed. Next, the remained correct corresponding pairs of points are used to reconstruct the three-dimensional model.Results & DiscussionIn this research, two sets of Point cloud have been used to evaluate the proposed process. These two data sets are obtained in such a way that in the first data set the perspective and angle of view and in the second data set the position and arrangement of objects are changed.
By forming descriptors based on spatial and geometric features in different neighborhood radii and then forming a proposed combination descriptor based on what has been mentioned, it can be considered that combining the geometric descriptors with spatial descriptors, in cases where The two datasets have less relative overlap or more relative rotation than each other, in contrast to the position shift, leading to improved descriptor performance and increased matching accuracy.
Considering the results obtained from the comparison of the proposed descriptors, it can be said that because of the existence of two different radii in each part of descriptors based on spatial and geometric relations in the proposed descriptors, it turns out that the required descriptor is high quality.
On the other hand, the properties used in these descriptors are also resistant to changing the position of objects and have high efficiency in mentioned category. Also, the process of identifying and eliminating incorrect correspondences improves the matching process and increases the matching percentage of similar points up to 25% in the study data set.ConclusionThe results of comparing the set of Point Cloud studied using the proposed descriptor indicate that this descriptor is more efficient in cases where two data sets rotate relative to each other, compared to cases where the location of the data pair has changed relative to each other. And the accuracy of the comparison obtained from the proposed method, in this case, increases compared to other data pair placement modes.
Keywords: Point Cloud, Registration, Descriptor, Geometric Properties, Spatial Propertied -
Pages 101-119Introduction
Coral reefs are one of the most diverse and ecologically important areas in the world. However, with increasing ocean temperatures, many coral reefs are severely threatened by bleaching events. When the water is too warm, corals expel the algae that live in their tissues, causing the coral to turn completely white. When a coral bleaches, it is not dead, and corals can survive a bleaching event, but they are more stressed and at risk of dying. Today, in order to predict and identify areas at risk of coral bleaching, data based on satellite remote sensing are used. In this research, using 35-year data trends, the sea surface temperature in 2022 was predicted using ArcGIS Pro tools for the Persian Gulf area and possible areas exposed to thermal stress leading to coral bleaching were identified.
Materials & MethodsIn order to predict the bleaching of corals, the research data archive of the American National Center for Atmospheric Research (NCAR) has been used. In this analysis, the harmonic method was used to fit the trend line. A harmonic trendline is a periodically repeating curved line that is best used to describe data that follows a cyclical pattern. For anomaly analysis parameters, the average monthly temperature in each location was compared with the overall average temperature to identify anomalies. There are three mathematical methods for calculating anomaly values with the Anomaly function, in this research, the method of difference From mean was used. At the end, the dimension value or band index was extracted, in which a certain statistic is obtained for each pixel in a multi-dimensional or multi-band raster, and the final map of coral bleaching prediction was prepared, and then using the data and global maps of the National Oceanic Administration NOAA , it was evaluated.
Results, discussion and conclusionThe preliminary results showed that the sea surface temperature has changed in the Persian Gulf. The range has experienced higher average temperatures since 1996, which could put the area at risk of coral bleaching. The minimum average temperature in the studied time period is 298.758 degrees Kelvin in 1991 and the maximum average temperature in 1399 is 300.737 degrees Kelvin. The parameters that were chosen for multidimensional data trend analysis include water surface temperature variable (SST) and time dimension. The obtained trend map (1980-2015) indicated that the northwestern regions of the Persian Gulf and a part of its south are more exposed to prolonged heat. In this study, frequency parameter 2 was used in the harmonic model, which uses the combination of the first-order linear harmonic curve and the second-order harmonic curve to fit the data. The accuracy of data trend fitting by harmonic regression function provided statistical parameters, R2=0.78 and RMSE=0.5. The value of R2 indicates that the observed value of sea surface temperature (SST) was predicted by the harmonic regression model by 78% and the rest remains undefined. This value of the determination coefficient confirmed the accuracy of the trend map. Another statistical parameter is the root mean square error, the lower the value, the better the fit. In the obtained results, the mean of this error is 0.5, which shows that the harmonic regression model can accurately predict the data. In this study, forecast data was analyzed to find locations where water temperatures remain warm for extended periods of time. In this context, first, anomalies in the data were calculated, anomaly or anomaly is the deviation of an observed value from its average value, and in the analysis, it shows areas that have a temperature higher than the average. As a result of this step, the anomalies in the data were calculated and the areas with higher temperature than the average were identified. In the predicted annual time frame (2022), the north-west and a part of the south of the Persian Gulf region will face a longer period of high temperature. To evaluate the accuracy of the results obtained from the analysis and the method used in predicting sea surface temperature and identifying anomalies (2022-09-03), they were compared with the maps of Nova organization on the same date and were confirmed. It is suggested that responsible organizations use methods based on remote sensing and trend analysis to assess the situation and prepare a risk map of coral reefs.
Keywords: Coral bleaching, harmonic trend analysis, Remote Sensing, sea surface temperature, Persian Gulf -
Pages 121-141Introduction
Undoubtedly, the main motivation of all planning is to achieve sustainable development, regional balance, proper distribution of activities and maximum use of environmental capabilities in the process of regional development. Land is a limited and vulnerable resource, but many of its benefits, if not to be abused, are eternal and renewable. In the planning system, the dimension of space is very important and the principles of spatial planning include the principles of sustainability, integrity and comprehensiveness.Developed countries and developing countries both need skills and guidelines in the field of spatial information, methods, frameworks, tools and templates that can use spatial information in timely and accessible decision-making and move forward and to be supportive to sustainable development goals.The purpose of this study is to investigate the applications and political and economic effects as well as the feasibility of using modern technologies such as: Internet of Things - Cloud Computing and Edge Computing - Artificial Intelligence and Machine Learning - Data Mining and Spatial Behavior Mining with the help of Virtual Reality and monitoring Spatial-Temporal changes, all of which are essential to Digital Twin, focusing on land management and sustainable development.
Materials & MethodsThis article is based on studying the findings and trends implemented in the three leading areas of land administration: The United States, Australia and the European Union and trying to localize those trends in our country.In this research, the comparative study method has been used, which shows a gap of at least ten years between the current situation of spatial information management in our country and what is happening in the leading countries in this field. The study areas include: architecture and structure-political and governance approach-emerging technologies-software- rules and restrictions.The enabler tools used in this research are the complete familiarity with architecture, software, technologies and current instructions in the field of geospatial sciences in the country, and on the other hand, the study of more than eighty articles and more than ten books in the field of the latest global achievements and the review of all reliable portals of the geospatial information and finally a comparative comparison of these two concepts and drawing a road map.Preparation of 2D and 3D cadastral maps of all geographic entities and assignment of legal and ownership information to these entities and then 3D visualization of these combined spatial data in a suitable portal for the preparation of functional spatial analysis are discussed in detail in the implementation method of this research.
Results & DiscussionUtilizing the knowledge and technology in creating a Digital Twin platform and adding its powerful tools to the country's national geospatial information infrastructure will lead to maximum productivity and the growth of the economy and social equality, and its highest feedback is the realization of sustainable development in the country.As the Gartner Institute's technology evaluation indexes as well as the CAGR index and the economic impact evaluation reports of the leading countries show, the acquisition of the Digital Twin technology will greatly contribute to the prosperity of the country's economy.In this research, an attempt has been made to mention all the technical and management tools necessary to achieve this infrastructure, and also a prioritization has been done according to the time of achieving the goals.
ConclusionThe results of this research show that filling the gap between the current state of the country's spatial information management and the desired state requires investment, culture and serious efforts of those involved in this field, and on the other hand, a positive point can be mentioned that with following the paths taken by developed countries, it is possible to achieve an optimal model and a clear and error-free path to achieve these goals.The localization of global instructions and the development of a conceptual model of action to resolve the existing technology gap will pave the way for the establishment of new technologies in the country, and with the maturity of the technical and operational branches of these models, we will come closer to the realization of sustainable development in the country.On the other hand, without cooperation and coordination between all the governmental bodies involved in the country's geomatic sciences, in addition to the private sector and the society, it seems unlikely to achieve this great achievement, because the creation of such a powerful infrastructure requires a harmonious and coherent national movement.It is hoped that this article will make a small achievement to the formation of strategic thinking in the management of spatial information sciences in the country and provide a complete picture of the dark corners of the development path as well as helping the decision makers in our beloved country.
Keywords: Didital Twin, NSDI, Land-use planning, Sustainable development -
Pages 143-161Introduction
More than 55% of the world's population now lives in cities, while around one billion people worldwide living in informal settlements. The city of Mashhad, as the second metropolis of Iran, has not been deprived of the phenomenon of marginalization and despite 3894 hectares of informal settlements is the second city in Iran in terms of the size of such settlements. Informal settlements in the city are less resilient than other parts of the city, mainly due to their distinct social and physical characteristics. Some argue are focusing only on physical factors of resilient, but this is not possible without considering the social factors and social and demographic characteristics of communities. if society is prepared to deal with that crisis, a large volume of disturbances and irregularities after the crisis will be reduced. Since the approach of sustainable urban regeneration in Mashhad has started since 2019 with the establishment of facilitation offices and considering that these offices emphasize the social dimension of regeneration and the participation of local community in this process, therefore, the results of the present study can be effective in this way. Therefore, this study aims to find the answers to the following questions with the aim of spatial analysis of social resilience in the suburbs of the city:How is the social resilience of the suburbs in Mashhad?
How is the spatial pattern of social resilience in Mashhad?Research MethodsSince the present study tries to analyze social resilience in the suburbs of Mashhad by using MCDM methods in the framework of urban resilience criteria, it has used descriptive-analytical method in the form of an applied research. For this purpose, based on library studies (articles, books, reports, and various documents), the required information in the field of social equity was collected. Then, by examining the dimensions and frameworks of social resilience, its criteria were determined and operationally defined. In this research, IDRISI software has been used to analyze the research data and evaluate them. Spatial statistics tools in ArcGIS software have been used to analyze the relationship between inefficiency distribution. Inefficiency pattern analysis is also performed by spatial autocorrelation technique. For this purpose, there are different models for measuring spatial autocorrelation statistics, among which the global Moran model and Gi statistic have been used. Spatial modeling of factors affecting inefficiency has been done by geographical weight regression.
Discussion and resultsSince there are 3894 hectares of informal settlements in Mashhad and due to the fact that these settlements have been formed over time and without regard to urban planning standards, so they are very sensitive to natural and unnatural hazards and in case of any crisis, returning to pre-accident conditions is important. There are several factors involved in this field, including physical, economic, and social factors. The outcome of all these dimensions will affect the return of these settlements to pre-crisis conditions. Meanwhile, a review of studies on resilience showed that the physical dimension of resilience has been emphasized more than its social dimension. In the current situation of a metropolis such as Mashhad, an important part of the population and area of Mashhad is its suburbs, which includes 66 neighborhoods with a population of nearly one million people and an area of 3894 hectares. If we consider the city as an integrated system, it should be said that other dimensions of resilience, including social resilience, will also affect other sectors, including the physical one.
ConclusionAnalysis of WLC, AHP and FUZZY methods, which were used in this study to evaluate the resilience of marginalized neighborhoods, showed that neighborhoods located in the northeast of Mashhad have more resilience than other areas, while the eastern and southeastern areas are less resilient. The social resilience pattern of these neighborhoods was evaluated by using the global Moran method and G general statistics. The results of this study showed that this zoning in the northeast and southeast is not random and has a spatial autocorrelation, so that in the northeast of the cluster of resilient neighborhoods, has led to increased resilience of other neighborhoods and in the southeast, low resilience has affected its reduction in adjacent areas. Warm and relatively warm clusters make up 1631 hectares of suburban areas, which is estimated to be equivalent to 42% of these neighborhoods. In fact, 31 neighborhoods in the suburbs of Mashhad are in hot and relatively hot clusters. The pattern of resilience is not significant elsewhere. Also, modeling the criteria studied in the study showed that the percentage of employed population, percentage of active population and average age have a significant effect on social resilience. According to the results of the leading research, in the process of re-creation that is taking place in the city of Mashhad, there should be a special look at the social dimensions of neighborhoods because the promotion of these dimensions can affect other aspects of resilience. Also, considering the impact of employment on the rate of resilience, it is suggested that in the process of recreating marginalized neighborhoods, special attention must be paid to job creation in these neighborhoods.
Keywords: resilience, Multi-criteria spatial decision support system, Spatial Autocorrelation, Geographic Weight Regression -
Pages 163-177Introduction
Replacing natural vegetation cover with impermeable urban surfaces) stone, cement, metal, etc.) has resulted in increased land surface temperature which is considered to be the most important problem of urban areas. Distinct temperature difference between the city and the surrounding areas is called heat island (Melkpour et al., 2018). Increased land surface temperature and resulting heat islands in urban areas built without proper preplanning (Khakpour et al., 2016) especially in developing countries such as Iran experiencing a rapid growth rate have resulted in widespread environmental problems. Heat islands mainly occur due to the presence of man-made surfaces which prevent the reflection of sunlight and result in temperature increase. In general, urban heat islands result in increased air and land surface temperature and thermal inversion (Gartland, 2012).
MethodologyThe present study applies a statistical-analytical research method based upon statistical data received from meteorological stations and extracted from satellite images. Climatic data recorded from 1976 to 2020 in Yazd Meteorological Station were retrieved from the General Meteorological Department of Yazd Province and used to measure temperature changes. Urban climate studies mainly take advantage of long-term patterns and thus, the present study has applied the common Man-Kendall method to measure the trend of temperature changes in warm season (July, August, and September). Also, satellite images collected by Landsat 4-8 in a 33-year period, including four statistical periods with a time interval of 11 years (the average recorded in July, August and September of 1987, 1998, 2009 and 2020), have been used to extract heat islands of Yazd city in warm seasons. These images collected under clear weather conditions were retrieved from the United States Geological Survey website (http://glovis.usgs.gov/) in the WGS-1984 UTM image system. NDVI index was used to investigate the vegetation cover. Main land uses discussed in the present study included barren lands, urban areas, vegetation cover and roads. Sample land uses were collected from Google Earth and visually interpreted in ArcMap. Maximum likelihood algorithm was used for the classification process. Finally, Land Surface Temperature was extracted from satellite images and compared with air temperature trend using the Mann-Kendall test.
Results & DiscussionResults indicate that due to thicker vegetation cover in summer, there has been a negative relationship between the vegetation cover and land surface temperature. In other words, land surface temperature has increased with decreased vegetation cover and vice versa. Types of land use identified in satellite images collected from Yazd city have showed that the city has experienced a widespread physical expansion during the 33-year statistical period regardless of the season under investigation and thus, built-up urban land use class has expanded significantly. As a result, vegetation cover has experienced a negative trend and decreased. Land surface temperature extracted from thermal images of Yazd city has proved parts of northwest and south of the city to be the core of its heat islands. This is due to the presence of barren lands, lack of evapotranspiration mechanisms, high heat absorption capacity and low conduction capacity. Man-Kendall test has found a significant increasing trend for temperature especially in recent years in which the temperature has increased about 2.3 °C. This is most possibly due to the increasing trend of urban population in recent decades, followed by increased residential structures and resulting heat island phenomenon.
ConclusionIn general, classification of urban land use types in Yazd has shown a significant physical expansion of the city during the statistical period. This physical development has occurred in all directions; beginning from the central and northeast-southeast parts, and moving towards northwest-southwest parts. Maximum NDVI was observed in a strip along the central part of Yazd in which vegetation cover is thicker. Green spaces are also observed in some areas of the city. Color spectrum of the LST map has shown relative changes of the ambient temperature in various parts of the city. High and very high temperature (between 41.5 and 50 °C) show the location of the heat islands on LST maps. Also, areas with a deep red color and a temperature above 50 °C have formed hot clusters formed or strengthened between 2009 and 2020 in the west and southwest parts of the city. Satellite images and related graphs have showed that in 2020, Yazd have witnessed a sharp increase in temperature and a heat island. Temperature data of Yazd Meteorological Station and Man-Kendall test have shown a significant increasing trend (about 2.3°C), especially in recent years. These are related to the urban population growth in recent decades, followed by increased urban structures (residential-commercial) and heat island phenomenon.
Keywords: Heat Island, Land surface temperature, Land use changes, NDVI, Yazd city -
Pages 179-192Introduction
Monitoring and investigation of land use changes in forest areas provides acceptable information for efficient management of these resources. Also, taking care protecting natural resources requires awareness of the conditions and how to change different land uses. Therefore, the purpose of this research is to evaluate the change of forest use in the forest area of Fandoqlu from 2010 to 2019 by using Landsat 5, 8 images and integrating them with Sentinel 2, Ester images. After preparing images from the years 2010, 2015 and 2019, geometrical, radiometric and atmospheric corrections of the images were done and the classification accuracy using kappa accuracy was% 93, %83, 91% respectively. The land use of Fandoqlu forest area was To model the change of use for 2025 with the Geomod model, it is necessary to prepare a suitability map of the area, which is prepared using the Fuzzy ANP method and the incompatibility coefficient is less than 0.06. In order to prepare a suitability map of four general factors: human, biological, topographic and climatic, and 12 sub-criteria were obtained with Boolean functions, and Boolean land use maps (forest and non-forest) 2010 and 2015 were modeled for 2019 and for modeling Land use for 2025 was done from the base map of 2019 and the transition matrix of Markov chain of land use in 2025 with the CA-Markov model And the result of location changes for 2025 was obtained. To evaluate the accuracy of the model, the agreement and non-agreement of pixels with Klocation and Standard were done with 98 and 95 accuracy, respectively. Modeling results for the year 2025 changes in a decreasing manner; The increase of non-utilized covers and the reduction of forest use, which will decrease from 3204.18 hectares in 2010 to 3070.55 hectares in 2019; According to the results of the human criterion and the sub-criteria of land use and distance from the road, the tourism potential of this area and the attraction of tourists as well as the interference of local residents can have a direct effect on this forest reduction process.
Materials & Methodsorganizations, people and local, is the only way to protect the forests of this region. In this study, remote sensing data such as satellite images of Landsat8,5, ASTER and Sentinel 2A were used to prepare the baseline map. Climatic data of all parameters up to 1396 were received from the synoptic station of Ardabil province. The digital model of 12.5 altitude was prepared from NASA website to prepare slope maps, slope direction, border layer of the study area and vegetation layer from Ardabil Natural Resources Organization. The research used Arc GIS, ENVI 5.3, TerrSet, eCognition 9 Google Earth pro and SUPER DECITION software. then based on the value and purpose of Reclassify and layer fuzzy. to predict the future conditions of forest cover changes by GEOMOD method, a time map of the start of the modeling process and a map of change appropriateness are needed. Geomod is used to model spatial patterns, forecast and probability of change. GEOMOD is used to simulate patterns of spatial change of use or change between two categories of use (forest and non-forest).
Results and DiscussionIn order to implement the GEOMOD model, a fit map prepared from the study area is required. Fuzzy ANP method was used to prepare the appropriateness map of the study area, which has four criteria: human (distance from the road, distance from the village, population), topography (slope, direction, height) and biological (land use, lithology, soil), criteria. And the following criteria are used in the map. Climatic parameter (average annual rainfall, temperature, altitude, slope, direction of slope, waterway) was used. 2025 user is required, so using 2015 and 2019 user with CA Markov model for 2025 was modeled. Decreased accuracy was associated. The results of predicting forest spatial changes for 2025 were used from the 2019 Boolean user map and the CA Markov modeled user map. Conclusion To implement the GEOMOD model, we need a fit map for spatial modeling of changes. In this study, four criteria and 12 sub-criteria discussed in Chapters 3 and 4 were used to prepare a fit map of the region. They have acquired Super Decision software.
ConclusionUsing the Boolean forest and non-forest boards and the 2015 and 2019 land use maps with the CA Markov model for 2025, it was modeled. Human, climate and biological have weights of 0.358, 0.258, 0.203 and 0.165, respectively, which the topography achieved the highest weight in Super Decision software. Among the sub-criteria, the type of land use has a high impact on changes in the region. The final output of the fit map was prepared by applying the OR function after applying the weights, which had a better result than the other functions. Finally, using the 2015 and 2025 user maps for 2025, forest spatial changes were made. To evaluate the accuracy of the model, the agreement and non-agreement of spatial pixels were used, which was modeled with Kappa 98% for 2019. The results of spatial change modeling show the high accuracy of the model in predicting spatial changes. GEOMOD results for 2025 will reach 3085 thousand hectares from 3151.9 hectares. Research conducted in different places. the country indicates a decline in forest areas in the coming decades.
Keywords: Land Use Change Modeling, GEOMOD, Fandoqlu