فهرست مطالب
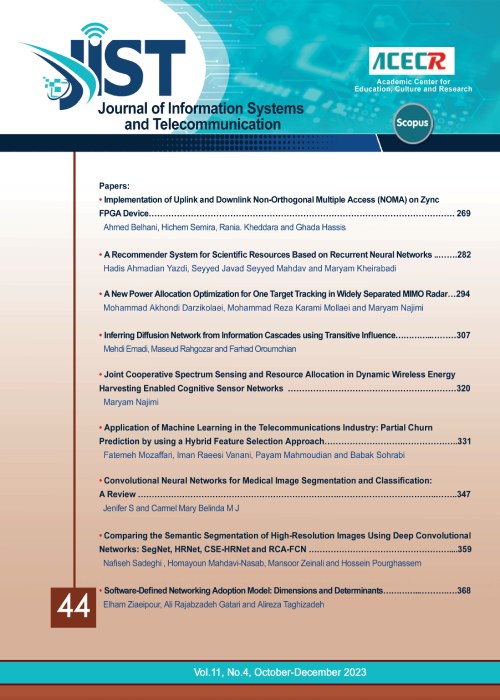
Journal of Information Systems and Telecommunication
Volume:11 Issue: 4, Oct-Dec 2023
- تاریخ انتشار: 1402/09/29
- تعداد عناوین: 9
-
-
Pages 269-281
The non-orthogonal access schemes are one of the multiple access techniques that are candidates to become an access technique for the next generation access radio. Power-domain non-orthogonal multiple-access (NOMA) is among these promising technologies. Improving the network capacity by providing massive connectivity through sharing the same spectral resources is the main advantage that this technique offers. The NOMA technique consists of exploiting the power domain which multiplex multiple users on the same resources applying a superposition coding then separating the multiplexed users at the receiver side. Due to the non-orthogonality access technique, the main disadvantage of NOMA is the presence of interferences between users. That is why this scheme is based on a successive interference cancelation (SIC) detector that separates the multiplexed signals at the receiver. In this paper, an embedded system is considered for designing and implementation of the power-NOMA For two users. The implementation is realized by employing a Zynq FPGA (Field programmable gate array) device through the Zybo-Z7 board using MATLAB/Simulink environment and Xilinx System Generator. The features offered by this device, hemps to consider the design of an uplink and a downlink scenario over Rayleigh fading channel in additive white Gaussian noise (AWGN) environment.
Keywords: Non-Orthogonal Multiple Access (NOMA), Successive Interference Cancelation (SIC), Multi-use Detection, Bit Error Rate (BER), QPSK, BPSK, Xilinx System Generator (XSG -
Pages 282-293
Over the last few years, online training courses have had a significant increase in the number of participants. However, most web-based educational systems have drawbacks compared to traditional classrooms. On the one hand, the structure and nature of the courses directly affect the number of active participants; on the other hand, it becomes difficult for teachers to guide students in choosing the appropriate learning resource due to the abundance of online learning resources. Students also find it challenging to decide which educational resources to choose according to their condition. The resource recommender system can be used as a Guide tool for educational resource recommendations to students so that these suggestions are tailored to the preferences and needs of each student. In this paper, it was presented a resource recommender system with the help of Bi-LSTM networks. Utilizing this type of structure involves both long-term and short-term interests of the user and, due to the gradual learning property of the system, supports the learners' behavioral changes. It has more appropriate recommendations with a mean accuracy of 0.95 and a loss of 0.19 compared to a similar article that uses DBN networks and only addresses the users' interests and tastes in the recent past.
Keywords: article Keywords: Deep Learning Networks, Recurrent Methods, Educational Resource Recommender System -
Pages 294-306
In this paper, a new power allocation scheme for one target tracking in MIMO radar with widely dispersed antennas is designed. This kind of radar applies multiple antennas which are deployed widely dispersed from each other. Therefore, a target is observed simultaneously from different uncorrelated angles and it offers spatial diversity. In this radar, a target’s radar cross section (RCS) is different in each transmit-receive path. So, a random complex Gaussian RCS is supposed for one target. Power allocation is used to allocate the optimum power to each transmit antenna and avoid illuminating the extra power in the environment and hiding it from interception. This manuscript aims to minimize the target tracking error with constraints on total transmit power and the power of each transmit antenna. For calculation of target tracking error, the joint Cramer Rao bound for a target velocity and position is computed and this is assumed as an objective function of the problem. It should be noted that a target RCS is also considered as unknown parameter and it is estimated along with target parameters. This makes a problem more similar to real conditions. After the investigation of the problem convexity, the problem is solved by particle swarm optimization (PSO) and sequential quadratic programming (SQP) algorithms. Then, various scenarios are simulated to evaluate the proposed scheme. The simulation results validate the accuracy and the effectiveness of the power allocation structure for target tracking in MIMO radar with widely separated antennas.
Keywords: unknown RCS, Target tracking, Power allocation, MIMO radar, Joint Cramer Rao bound, PSO, SQP -
Pages 307-319
Online social networks have a great impact on people’s life and how they interact. When information is transmitted from one person to another in a social network, a diffusion process occurs. Each node of a network that participates in the diffusion process leaves some effects on this process, such as its transmission time. In most cases, despite the visibility of such effects of diffusion process, the structure of the network is unknown. Knowing the structure of a social network is essential for many research studies such as: such as community detection, expert finding, influence maximization, information diffusion, sentiment propagation, immunization against rumors, etc. In recent years, various methods have been proposed for inferring a diffusion network. A wide range of proposed models, named parametric models, assume that the pattern of the propagation process follows a particular distribution. Also, the models provided for large volumes of data do not have the required performance due to their high execution time. However, in this article, a nonparametric model is proposed that infers the underlying diffusion network. In the proposed model, all potential edges between the network nodes are identified using a similarity-based link prediction method. Then, a fast algorithm for graph pruning is used to reduce the number of edges. The proposed algorithm uses the transitive influence principle in social networks. Comparison of the proposed method on different network types and various models of information cascades show that the model performs better precision and decreases the execution time too.
Keywords: Transitive influence, network inferring, diffusion network -
Pages 320-330
Due to the limitations of the natural frequency spectrum, dynamic frequency allocation is required for wireless networks. Spectrum sensing of a radio channel is a technique to identify the spectrum holes. In this paper, we investigate a dynamic cognitive sensor network, in which the cognitive sensor transmitter has the capability of the energy harvesting. In the first slot, the cognitive sensor transmitter participates in spectrum sensing and in the existence of the primary user, it harvests the energy from the primary signal, otherwise the sensor transmitter sends its signal to the corresponding receiver while in the second slot, using the decode-and-forward (DF) protocol, a part of the bandwidth is used to forward the signal of the primary user and the remained bandwidth is used for transmission of the cognitive sensor. Therefore, our purposed algorithm is to maximize the cognitive network transmission rate by selection of the suitable cognitive sensor transmitters subject to the rate of the primary transmission and energy consumption of the cognitive sensors according to the mobility model of the cognitive sensors in the dynamic network. Simulation results illustrate the effectiveness of the proposed algorithm in performance improvement of the network as well as reducing the energy consumption.
Keywords: Cognitive Sensor Network, Transmission rate, Mobility Model, Decode-and-forward(DF) Protocol, Energy Consumption -
Pages 331-346
The telecommunications industry is one of the most competitive industries in the world. Because of the high cost of customer acquisition and adverse effects of customer churn on the company's performance, customer retention becomes an inseparable part of strategic decision-making and one of the main objectives of customer relationship management. Although customer churn prediction models are widely studied in various domains, several challenges remain in designing and implementing an effective model. This paper attempts to address the customer churn prediction problem with a practical approach. The experimental analysis was conducted on the actual customers' data gathered from available data sources at a telecom company in Iran. First, the partial churn was defined in a new way which exploits the status of customers based on the criteria that can be measured easily in the telecommunications industry. Moreover, a hybrid feature selection approach was proposed in which various feature selection methods, along with the wisdom of the crowd, were applied. It was found that the wisdom of the crowd can be used as a useful feature selection method. Finally, a predictive model was developed using advanced machine learning algorithms such as bagging, boosting, stacking, and deep learning. The partial customer churn was predicted with more than 88% accuracy by the Gradient Boosting Machine algorithm by using 5-fold cross-validation. Comparative results indicate that the proposed model performs efficiently compared to the ones applied in the previous studies.
Keywords: Partial Churn, Churn Prediction, Machine Learning, Feature Selection, Telecommunications Industry, The Wisdom of the Crowd -
Pages 347-358
Deep learning has revamped medical image analysis, yielding excellent results in image processing tasks such as registration, segmentation, feature extraction, and classification. The prime motivations for this are the availability of computational resources and the resurgence of deep Convolutional Neural Networks. Deep learning techniques are good at observing hidden patterns in images and supporting clinicians in achieving diagnostic perfection. In this paper, we review the works exploiting current state-of-the-art deep learning approaches in medical image processing. We begin the survey by providing a synopsis of research works in medical imaging based on convolutional neural networks. Second, we discuss popular pre-trained models and General Adversarial Networks that aid in improving convolutional networks’ performance. Finally, to ease direct evaluation, we compile the performance metrics of deep learning models focusing on covid-19 detection and child bone age prediction.
Keywords: Medical image analysis, Deep learning, Convolutional neural networks, Transfer learning, Generative adversarial network -
Pages 359-367
Semantic segmentation is a branch of computer vision, used extensively in image search engines, automated driving, intelligent agriculture, and disaster management, and other machine-human interactions. Semantic segmentation aims to predict a label for each pixel from a given label set, according to semantic information. Among the proposed methods and architectures, researchers have focused on deep learning algorithms due to their good feature learning results. Thus, many studies have explored the structure of deep neural networks, especially convolutional neural networks. Most of the modern semantic segmentation models are based on fully convolutional networks (FCN), which first replaces the fully connected layers in common classification networks by convolutional layers, getting pixel-level prediction results. After that, a lot of methods are proposed to improve the basic FCN methods results. With the increasing complexity and variety of existing data structures, more powerful neural networks and the development of existing networks are needed. This study aims to segment a high-resolution (HR) image dataset into six separate classes. This paper semantic segmentation methods based on deep learning. Here, an overview of some important deep learning architectures will be presented with a focus on methods producing remarkable scores in segmentation metrics such as accuracy and F1-score. Finally, their segmentation results will be discussed.
Keywords: Semantic segmentation, Convolutional neural network, Deep neural network, High-resolution image processing -
Pages 368-382
The recent technical trend in the field of communication networks shows a paradigm change from hardware to software. Software Defined Networking (SDN) as one of the enablers of digital transformation could have prominent role in this paradigm shift and migration to Knowledge-based network. In this regard, telecom operators are interested in deploying SDN to migrate their infrastructure from a static architecture to a dynamic and programmable platform. However, it seems that they do not consider SDN as one of their priorities and still depend on traditional methods to manage their network (especially in some developing countries such as Iran). Since the first step in applying new technologies is to accept them, we have proposed a comprehensive SDN adoption model with the mixed-method research methodology. At first, the theoretical foundations related to the research problem were examined. Then, based on Grounded theory, in-depth interviews were conducted with 12 experts (including university professors and managers of the major telecom operators). In result, more than a thousand initial codes were determined, which in the review stages and based on semantic commonalities, a total of 112 final codes, 14 categories and 6 themes have been extracted using open, axial and selective coding. Next, in order to confirm the indicators extracted from the qualitative part, the fuzzy Delphi method has been used. In the end, SPSS and SmartPLS 3 software were used to analyze the data collected from the questionnaire and to evaluate the fit of the model as well as confirm and reject the hypotheses.
Keywords: Adoption, Service provider, Software Defined Network, Technology